Open-vocabulary Object Segmentation with Diffusion Models.
Proceedings of the IEEE/CVF International Conference on Computer Vision (ICCV)(2023)
摘要
The goal of this paper is to extract the visual-language correspondence from a pre-trained text-to-image diffusion model, in the form of segmentation map, i.e., simultaneously generating images and segmentation masks for the corresponding visual entities described in the text prompt. We make the following contributions: (i) we pair the existing Stable Diffusion model with a novel grounding module, that can be trained to align the visual and textual embedding space of the diffusion model with only a small number of object categories; (ii) we establish an automatic pipeline for constructing a dataset, that consists of {image, segmentation mask, text prompt} triplets, to train the proposed grounding module; (iii) we evaluate the performance of open-vocabulary grounding on images generated from the text-to-image diffusion model and show that the module can well segment the objects of categories beyond seen ones at training time, as shown in Fig. 1; (iv) we adopt the augmented diffusion model to build a synthetic semantic segmentation dataset, and show that, training a standard segmentation model on such dataset demonstrates competitive performance on the zero-shot segmentation (ZS3) benchmark, which opens up new opportunities for adopting the powerful diffusion model for discriminative tasks.
更多查看译文
关键词
segmentation,diffusion models,open-vocabulary
AI 理解论文
溯源树
样例
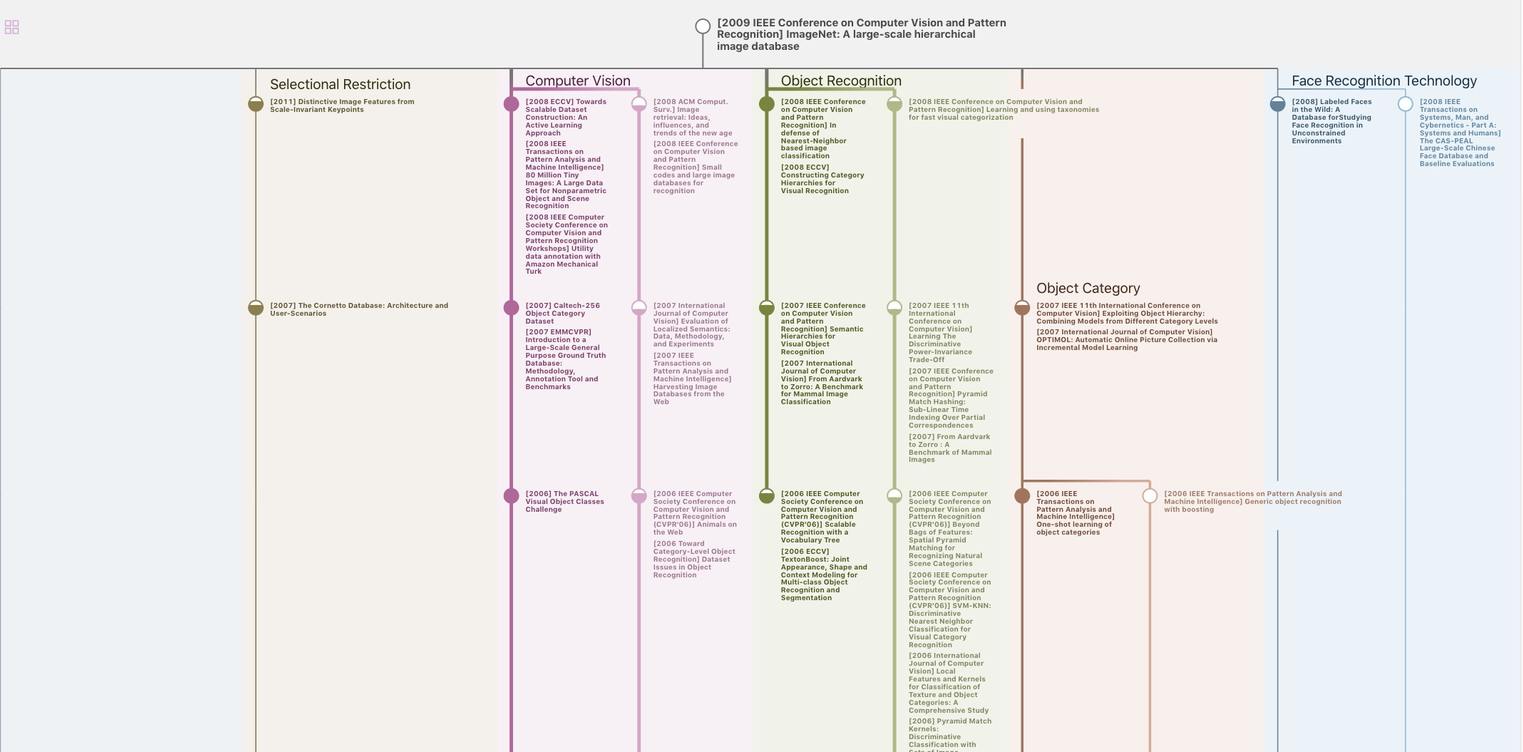
生成溯源树,研究论文发展脉络
Chat Paper
正在生成论文摘要