Tiny Updater: Towards Efficient Neural Network-Driven Software Updating.
Proceedings of the IEEE/CVF International Conference on Computer Vision (ICCV)(2023)
摘要
Significant advancements have been accomplished with deep neural networks in diverse visual tasks, which have substantially elevated their deployment in edge device software. However, during the update of neural network-based software, users are required to download all the parameters of the neural network anew, which harms the user experience. Motivated by previous progress in model compression, we propose a novel training methodology named Tiny Updater to address this issue. Specifically, by adopting the variant of pruning and knowledge distillation methods, Tiny Updater can update the neural network-based software by only downloading a few parameters (10%~20%) instead of all the parameters in the neural network. Experiments on eleven datasets of three tasks, including image classification, image-to-image translation, and video recognition have demonstrated its effectiveness. Codes have been released in https://github.com/ArchipLab-LinfengZhang/TinyUpdater.
更多查看译文
AI 理解论文
溯源树
样例
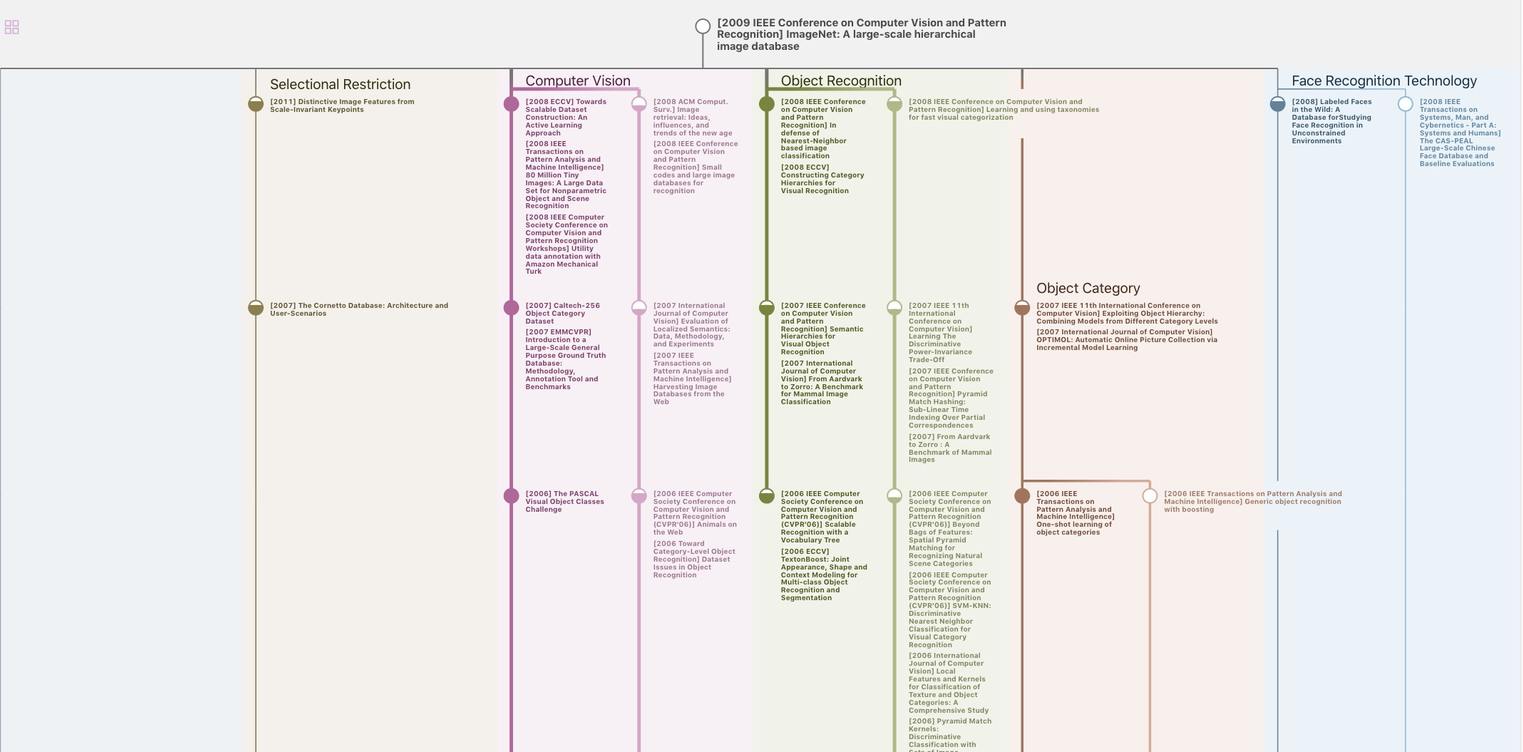
生成溯源树,研究论文发展脉络
Chat Paper
正在生成论文摘要