Attention Discriminant Sampling for Point Clouds.
Proceedings of the IEEE/CVF International Conference on Computer Vision (ICCV)(2023)
摘要
This paper describes an attention-driven approach to 3-D point cloud sampling. We establish our method based on a structure-aware attention discriminant analysis that explores geometric and semantic relations embodied among points and their clusters. The proposed attention discriminant sampling (ADS) starts by efficiently decomposing a given point cloud into clusters to implicitly encode its structural and geometric relatedness among points. By treating each cluster as a structural component, ADS then draws on evaluating two levels of self-attention: within-cluster and between-cluster. The former reflects the semantic complexity entailed by the learned features of points within each cluster, while the latter reveals the semantic similarity between clusters. Driven by structurally preserving the point distribution, these two aspects of self-attention help avoid sampling redundancy and decide the number of sampled points in each cluster. Extensive experiments demonstrate that ADS significantly improves classification performance to 95.1% on ModelNet40 and 87.5% on ScanObjectNN and achieves 86.9% mIoU on ShapeNet Part Segmentation. For scene segmentation, ADS yields 91.1% accuracy on S3DIS with higher mIoU to the state-of-the-art and 75.6% mIoU on ScanNetV2. Furthermore, ADS surpasses the state-of-the-art with 55.0% mAP
50
on ScanNetV2 object detection.
更多查看译文
AI 理解论文
溯源树
样例
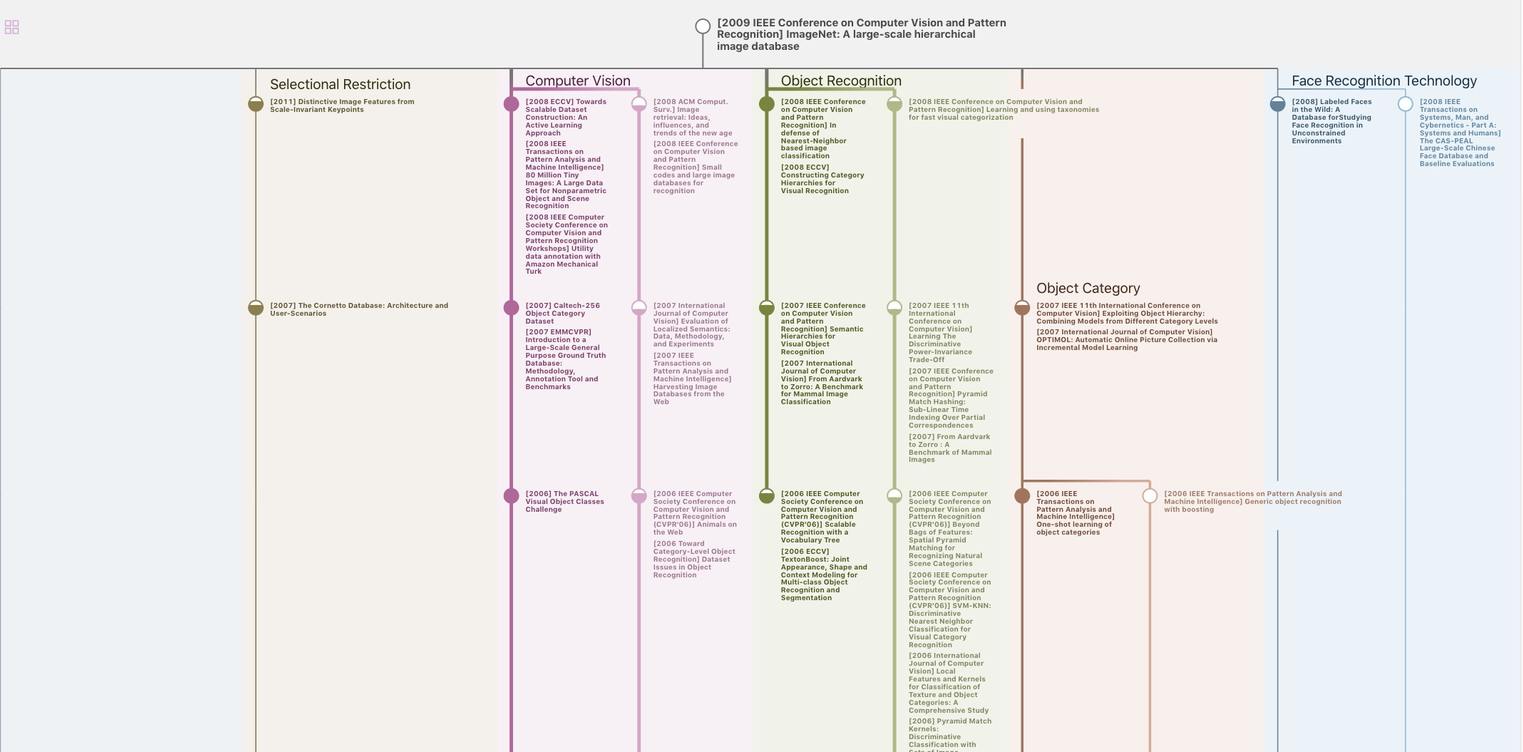
生成溯源树,研究论文发展脉络
Chat Paper
正在生成论文摘要