Neural Fields for Structured Lighting.
Proceedings of the IEEE/CVF International Conference on Computer Vision (ICCV)(2023)
摘要
We present an image formation model and optimization procedure that combines the advantages of neural radiance fields and structured light imaging. Existing depth-supervised neural models rely on depth sensors to accurately capture the scene’s geometry. However, the depth maps recovered by these sensors can be prone to error, or even fail outright. Instead of depending on the fidelity of processed depth maps from a structured light system, a more principled approach is to explicitly model the raw structured light images themselves. Our proposed approach enables the estimation of high-fidelity depth maps, including for objects with complex material properties (e.g., partially-transparent surfaces). Besides computing depth, the raw structured light images also confer other useful radiometric cues, which enable predicting surface normals and decomposing scene appearance in terms of a direct, indirect, and ambient component. We evaluate our framework quantitatively and qualitatively on a range of real and synthetic scenes, and decompose scenes into their constituent components for novel views.
更多查看译文
AI 理解论文
溯源树
样例
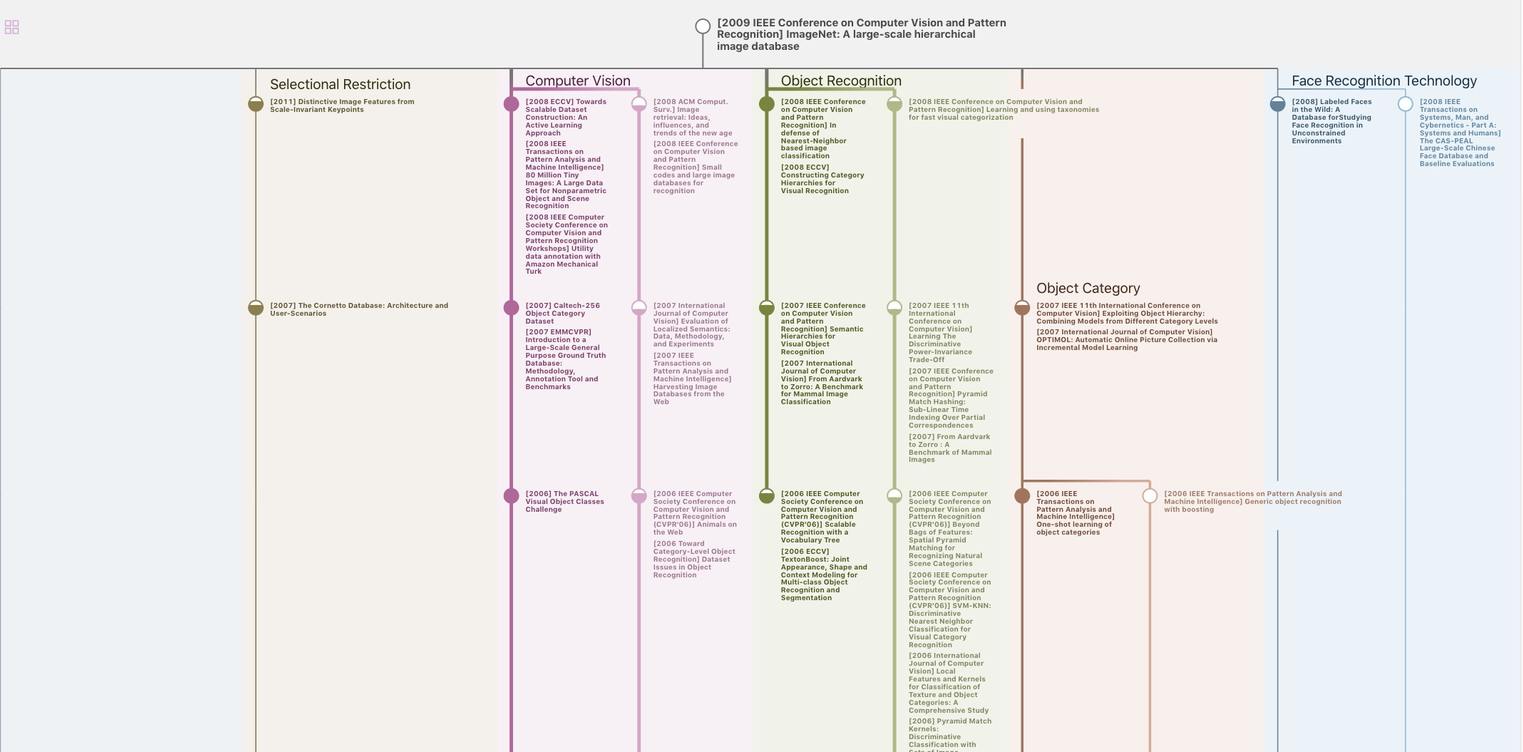
生成溯源树,研究论文发展脉络
Chat Paper
正在生成论文摘要