Gramian Attention Heads are Strong yet Efficient Vision Learners
Proceedings of the IEEE/CVF International Conference on Computer Vision (ICCV)(2023)
摘要
We introduce a novel architecture design that enhances expressiveness by incorporating multiple head classifiers (i.e., classification heads) instead of relying on channel expansion or additional building blocks. Our approach employs attention-based aggregation, utilizing pairwise feature similarity to enhance multiple lightweight heads with minimal resource overhead. We compute the Gramian matrices to reinforce class tokens in an attention layer for each head. This enables the heads to learn more discriminative representations, enhancing their aggregation capabilities. Furthermore, we propose a learning algorithm that encourages heads to complement each other by reducing correlation for aggregation. Our models eventually surpass state-of-the-art CNNs and ViTs regarding the accuracy-throughput trade-off on ImageNet-1K and deliver remarkable performance across various downstream tasks, such as COCO object instance segmentation, ADE20k semantic segmentation, and fine-grained visual classification datasets. The effectiveness of our framework is substantiated by practical experimental results and further underpinned by generalization error bound. We release the code publicly at: https://github.com/Lab-LVM/imagenet-models.
更多查看译文
关键词
attention,vision,learners,efficient
AI 理解论文
溯源树
样例
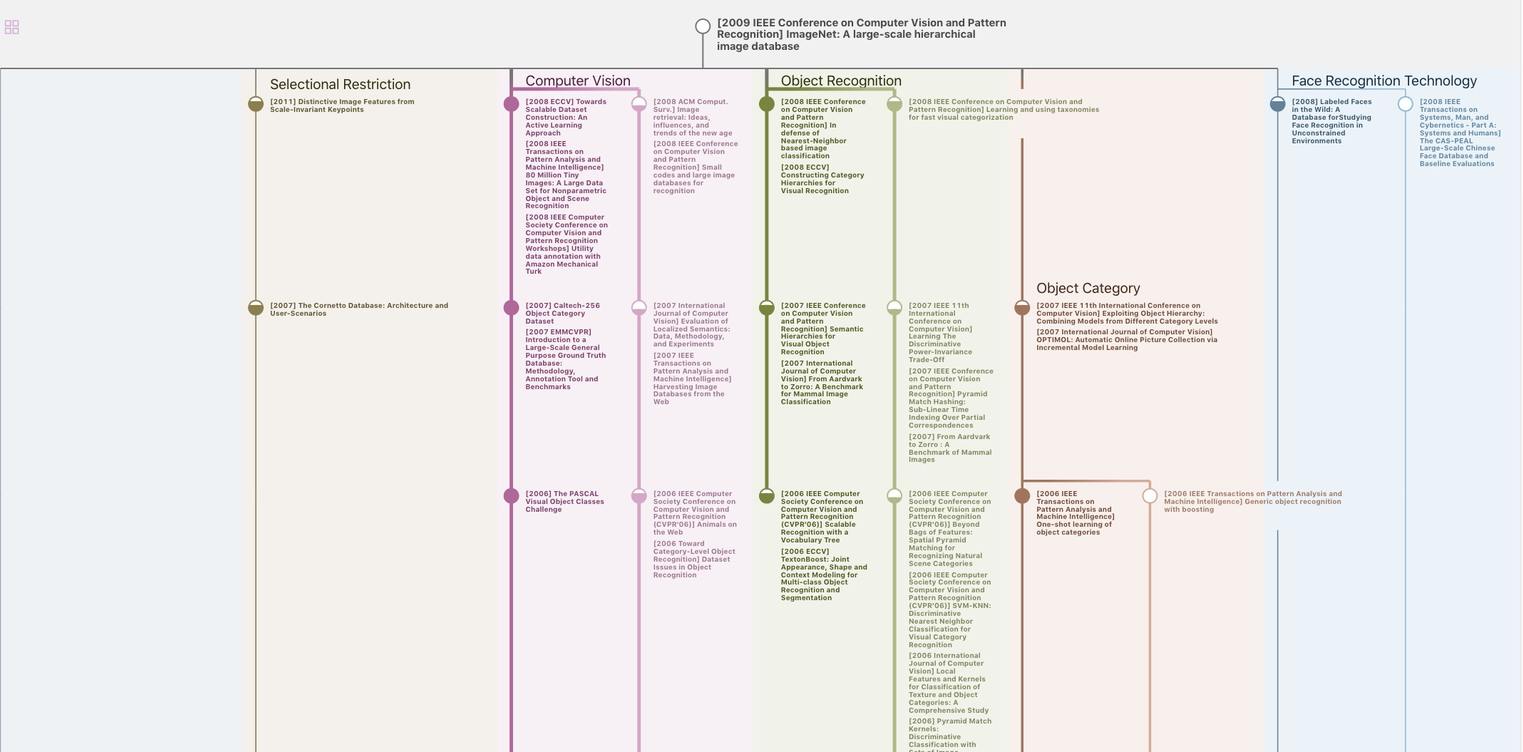
生成溯源树,研究论文发展脉络
Chat Paper
正在生成论文摘要