Overcoming Forgetting Catastrophe in Quantization-Aware Training.
Proceedings of the IEEE/CVF International Conference on Computer Vision (ICCV)(2023)
摘要
Quantization is an effective approach for memory cost reduction by compressing networks to lower bits. However, existing quantization processes learned only from the current data tend to suffer from forgetting catastrophe on streaming data, i.e., significant performance decrement on old task data after being trained on new tasks. Therefore, we propose a lifelong quantization process, LifeQuant, to address the problem. We theoretically analyze the forgetting catastrophe from the shift of quantization search space with the change of data tasks. To overcome the forgetting catastrophe, we first minimize the space shift during quantization and propose Proximal Quantization Space Search (ProxQ), for regularizing the search space during quantization to be close to a pre-defined standard space. Afterward, we exploit replay data (a subset of old task data) for retraining in new tasks to alleviate the forgetting problem. However, the limited amount of replay data usually leads to biased quantization performance toward the new tasks. To address the imbalance issue, we design a Balanced Lifelong Learning (BaLL) Loss to reweight (to increase) the influence of replay data in new task learning, by leveraging the class distributions. Experimental results show that LifeQuant achieves outstanding accuracy performance with a low forgetting rate.
更多查看译文
AI 理解论文
溯源树
样例
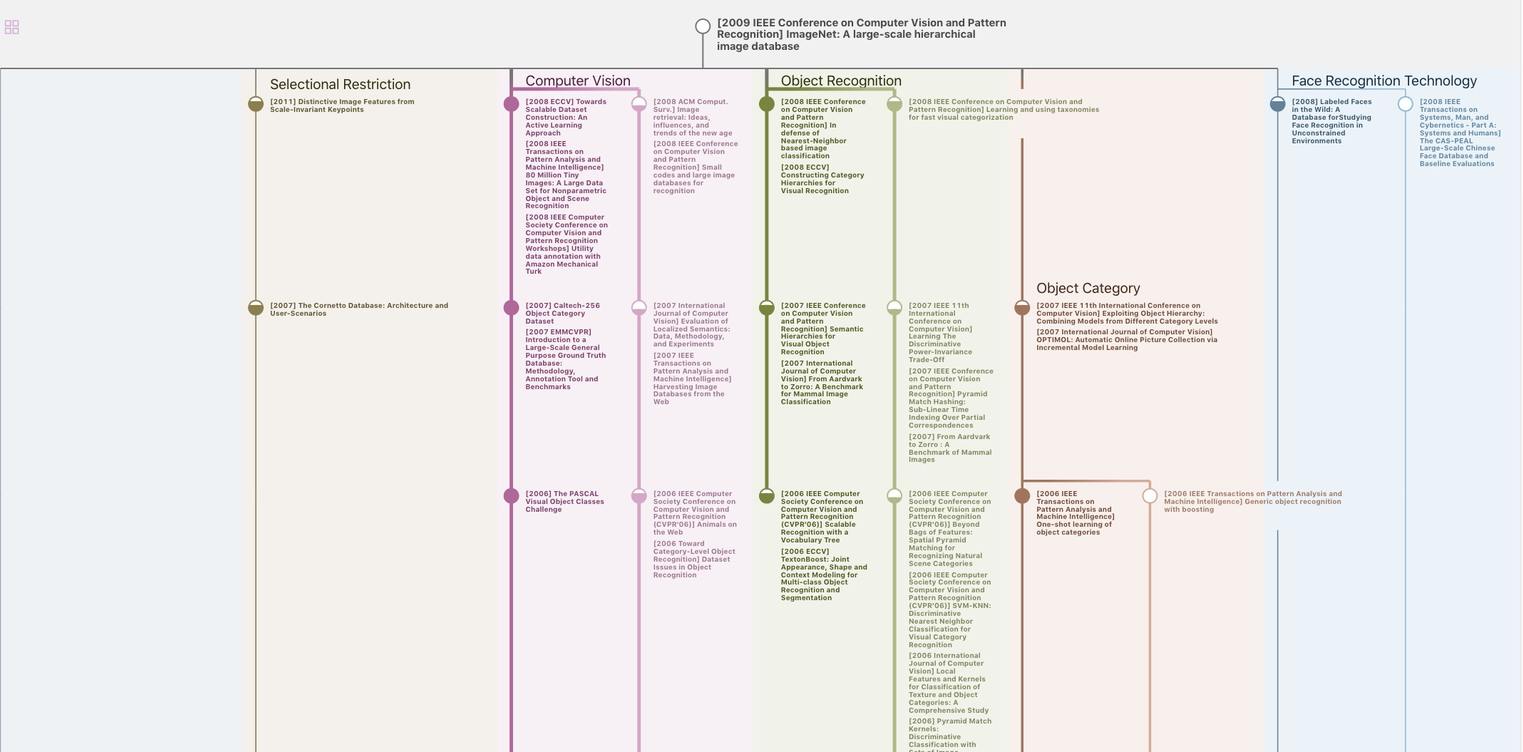
生成溯源树,研究论文发展脉络
Chat Paper
正在生成论文摘要