An Embarrassingly Simple Backdoor Attack on Self-supervised Learning.
Proceedings of the IEEE/CVF International Conference on Computer Vision (ICCV)(2023)
摘要
As a new paradigm in machine learning, self-supervised learning (SSL) is capable of learning high-quality representations of complex data without relying on labels. In addition to eliminating the need for labeled data, research has found that SSL improves the adversarial robustness over supervised learning since lacking labels makes it more challenging for adversaries to manipulate model predictions. However, the extent to which this robustness superiority generalizes to other types of attacks remains an open question.We explore this question in the context of backdoor attacks. Specifically, we design and evaluate Ctrl, an embarrassingly simple yet highly effective self-supervised backdoor attack. By only polluting a tiny fraction of training data (≤ 1%) with indistinguishable poisoning samples, Ctrl causes any trigger-embedded input to be misclassified to the adversary's designated class with a high probability (≥ 99%) at inference time. Our findings suggest that SSL and supervised learning are comparably vulnerable to backdoor attacks. More importantly, through the lens of Ctrl, we study the inherent vulnerability of SSL to backdoor attacks. With both empirical and analytical evidence, we reveal that the representation invariance property of SSL, which benefits adversarial robustness, may also be the very reason making SSL highly susceptible to backdoor attacks. Our findings also imply that the existing defenses against supervised backdoor attacks are not easily retrofitted to the unique vulnerability of SSL. Code is available at: https://github.com/meet-cjli/CTRL
更多查看译文
关键词
embarrassingly simple backdoor attack,learning,self-supervised
AI 理解论文
溯源树
样例
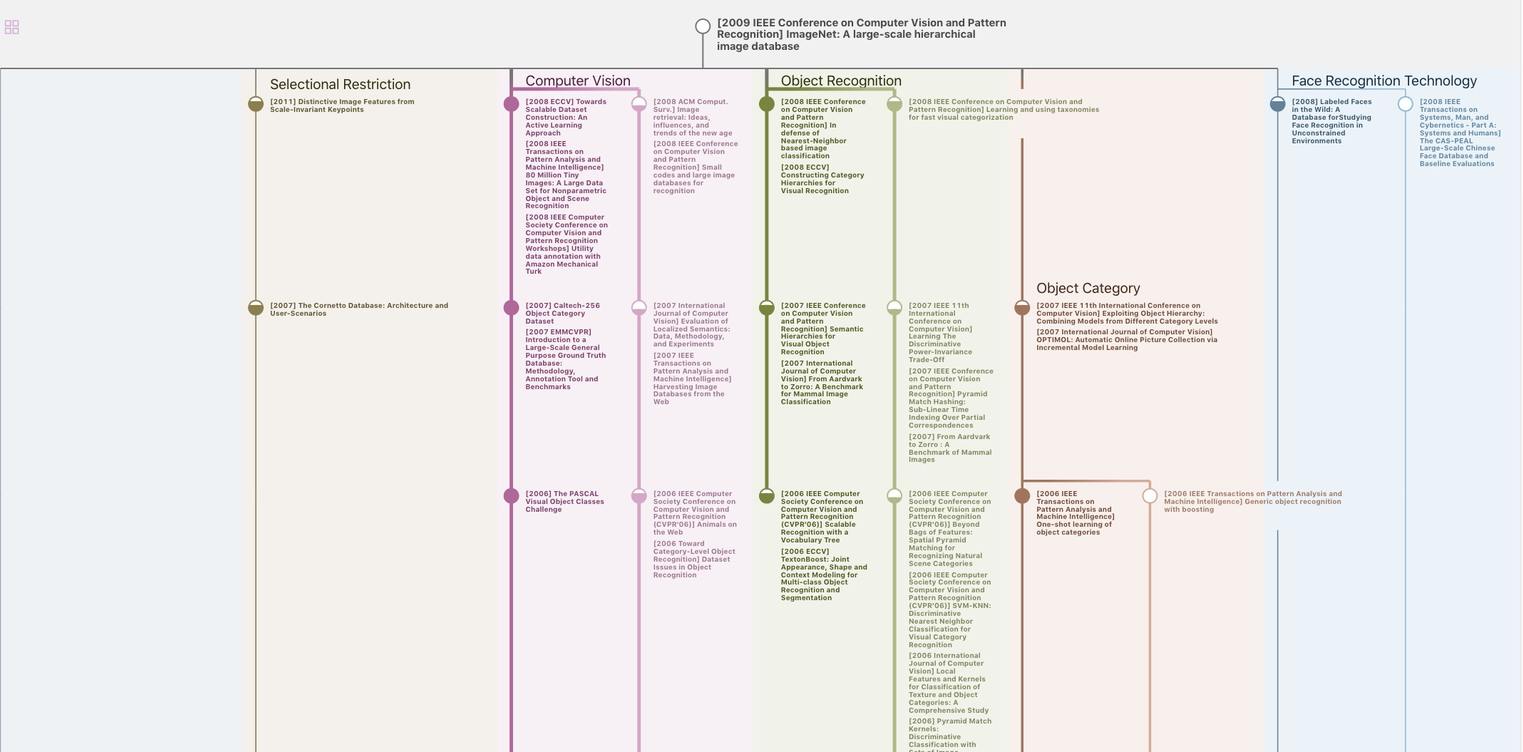
生成溯源树,研究论文发展脉络
Chat Paper
正在生成论文摘要