Data-Free Class-Incremental Hand Gesture Recognition.
Proceedings of the IEEE/CVF International Conference on Computer Vision (ICCV)(2023)
摘要
This paper investigates data-free class-incremental learning (DFCIL) for hand gesture recognition from 3D skeleton sequences. In this class-incremental learning (CIL) setting, while incrementally registering the new classes, we do not have access to the training samples (i.e. data-free) of the already known classes due to privacy. Existing DFCIL methods primarily focus on various forms of knowledge distillation for model inversion to mitigate catastrophic forgetting. Unlike SOTA methods, we delve deeper into the choice of the best samples for inversion. Inspired by the well-grounded theory of max-margin classification, we find that the best samples tend to lie close to the approximate decision boundary within a reasonable margin. To this end, we propose BOAT-MI – a simple and effective boundary-aware prototypical sampling mechanism for model inversion for DFCIL. Our sampling scheme outperforms SOTA methods significantly on two 3D skeleton gesture datasets, the publicly available SHREC 2017, and EgoGesture3D – which we extract from a publicly available RGBD dataset. Both our codebase and the EgoGesture3D skeleton dataset are publicly available: https://github.com/humansensinglab/dfcil-hgr.
更多查看译文
AI 理解论文
溯源树
样例
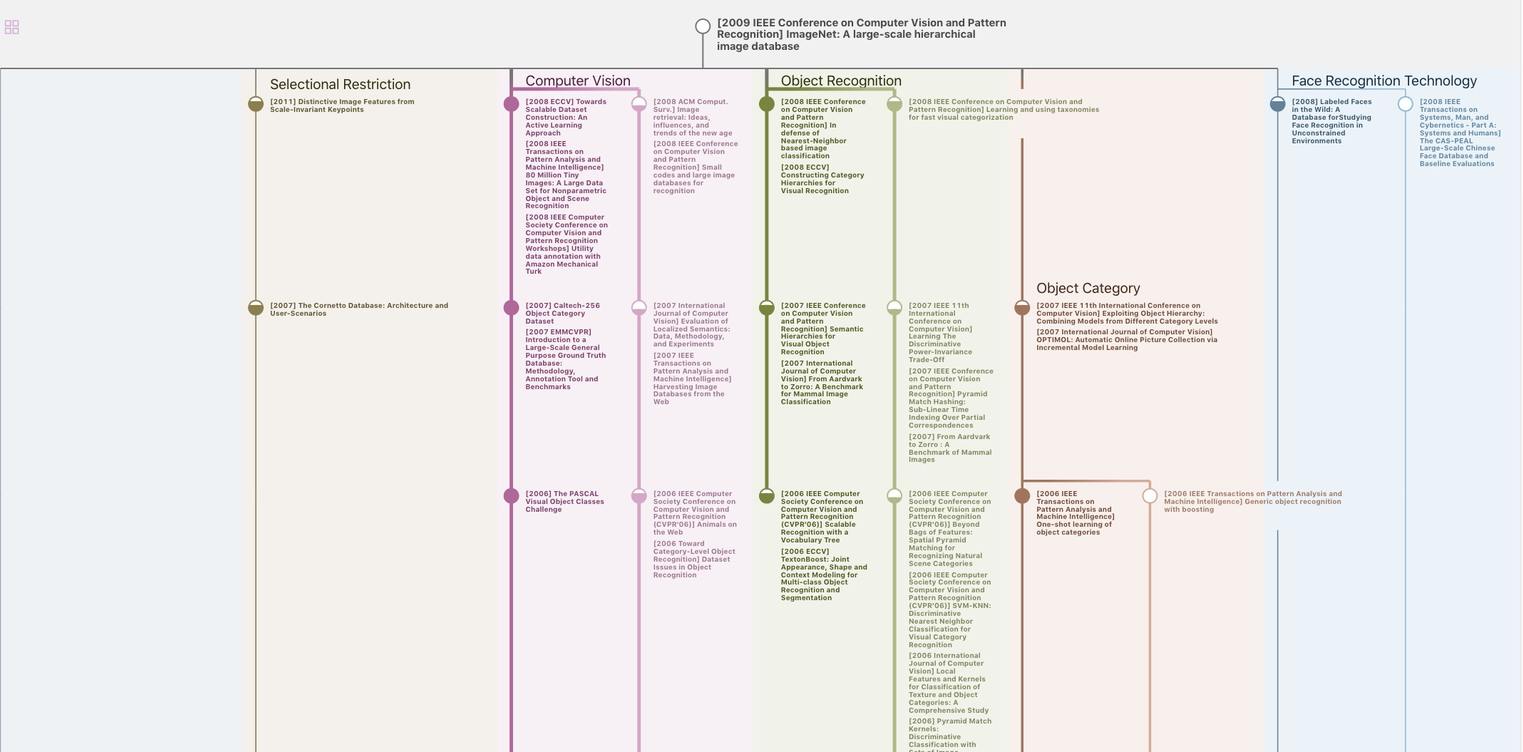
生成溯源树,研究论文发展脉络
Chat Paper
正在生成论文摘要