Re: PolyWorld - A Graph Neural Network for Polygonal Scene Parsing.
Proceedings of the IEEE/CVF International Conference on Computer Vision (ICCV)(2023)
摘要
While most state-of-the-art instance segmentation methods produce pixel-wise segmentation masks, numerous applications demand precise vector polygons of detected objects instead of rasterized output. This paper proposes Re:PolyWorld as a remastered and improved version of PolyWorld, a neural network that extracts object vertices from an image and connects them optimally to generate precise polygons. The objective of this work was to overcome weaknesses and shortcomings of the original model, as well as introducing an improved polygonal representation to obtain a general-purpose method for polygon extraction in images. The architecture has been redesigned to not only exploit vertex features, but to also make use of the visual appearance of edges. To this end, an edge-aware Graph Neural Network predicts the connection strength between each pair of vertices, which is further used to compute the assignment by solving a differentiable optimal transport problem. The proposed redefinition of the polygonal scene turns the method into a powerful generalized approach that can be applied to a large variety of tasks and problem settings, such as building extraction, floorplan reconstruction and even wireframe parsing. Re:PolyWorld not only outperforms the original model on building extraction in aerial images, thanks to the proposed joint analysis of vertices and edges, but also beats the state-of-the-art in multiple other domains.
更多查看译文
AI 理解论文
溯源树
样例
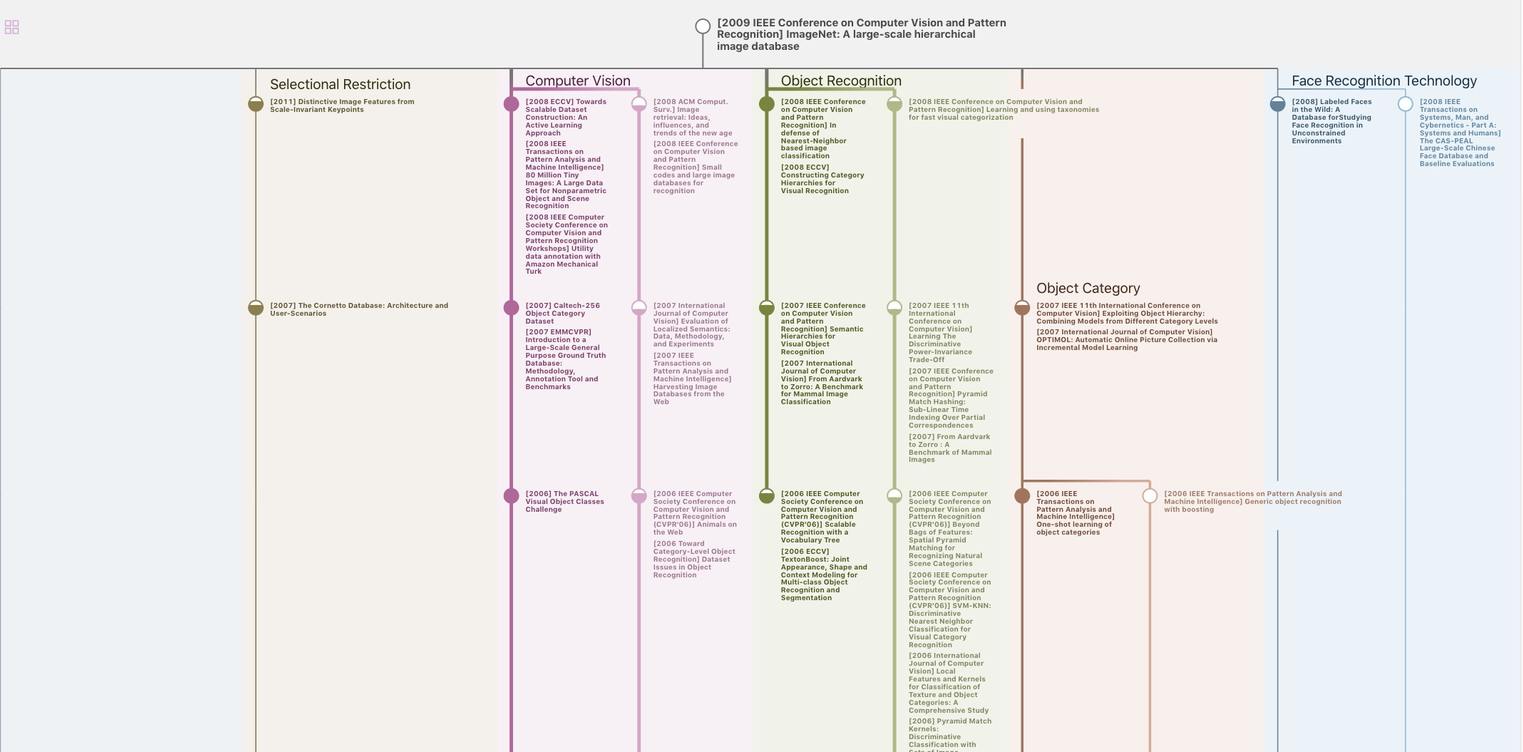
生成溯源树,研究论文发展脉络
Chat Paper
正在生成论文摘要