How connectivity structure shapes rich and lazy learning in neural circuits.
ArXiv(2024)
摘要
In theoretical neuroscience, recent work leverages deep learning tools to explore how some network attributes critically influence its learning dynamics. Notably, initial weight distributions with small (resp. large) variance may yield a rich (resp. lazy) regime, where significant (resp. minor) changes to network states and representation are observed over the course of learning. However, in biology, neural circuit connectivity could exhibit a low-rank structure and therefore differs markedly from the random initializations generally used for these studies. As such, here we investigate how the structure of the initial weights -- in particular their effective rank -- influences the network learning regime. Through both empirical and theoretical analyses, we discover that high-rank initializations typically yield smaller network changes indicative of lazier learning, a finding we also confirm with experimentally-driven initial connectivity in recurrent neural networks. Conversely, low-rank initialization biases learning towards richer learning. Importantly, however, as an exception to this rule, we find lazier learning can still occur with a low-rank initialization that aligns with task and data statistics. Our research highlights the pivotal role of initial weight structures in shaping learning regimes, with implications for metabolic costs of plasticity and risks of catastrophic forgetting.
更多查看译文
关键词
Computational neuroscience,recurrent neural networks,learning,connectivity structure,inductive bias,rich and lazy learning,deep learning theory,neural representations
AI 理解论文
溯源树
样例
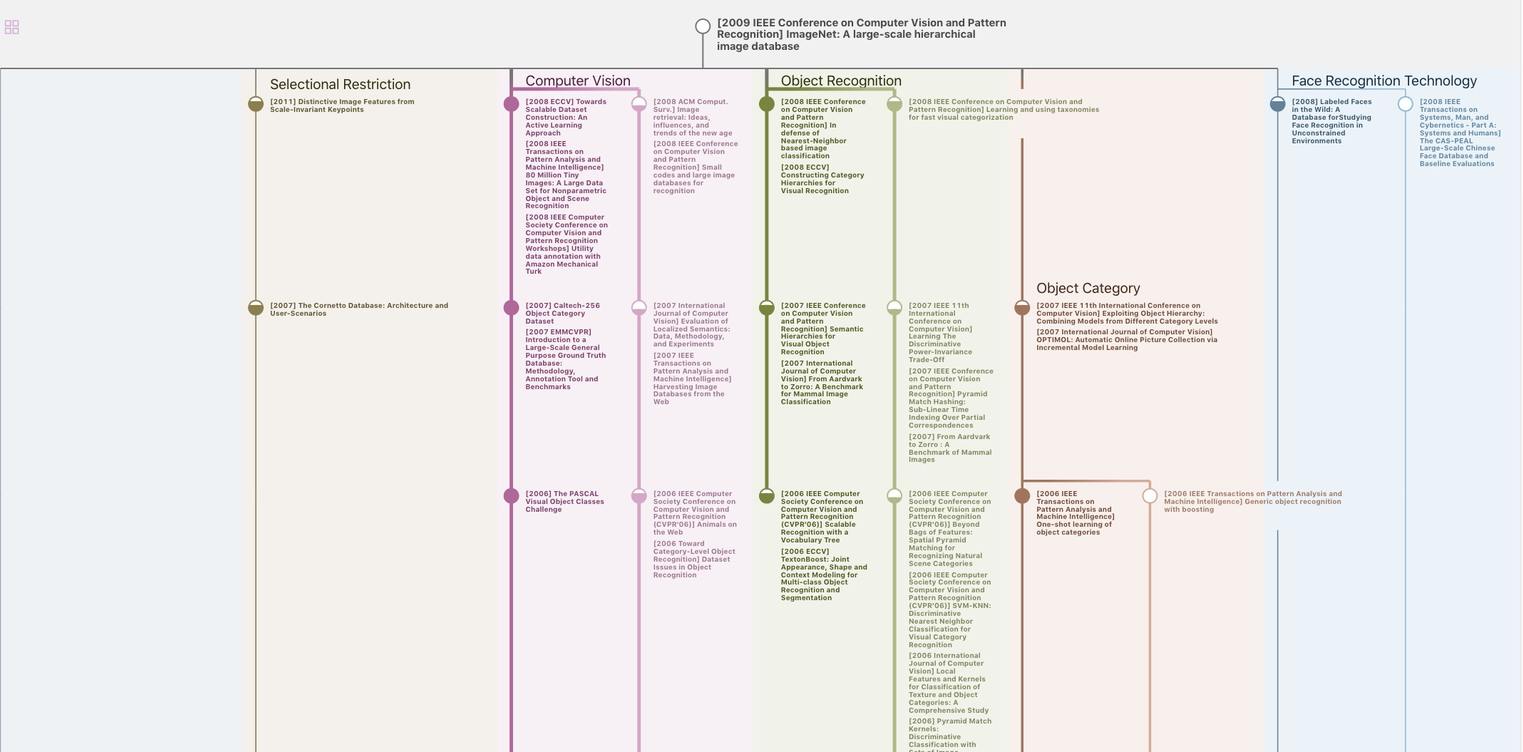
生成溯源树,研究论文发展脉络
Chat Paper
正在生成论文摘要