Counterfactual Explanations for Time Series Forecasting
23RD IEEE INTERNATIONAL CONFERENCE ON DATA MINING, ICDM 2023(2023)
摘要
Among recent developments in nine series forecasting methods, deep forecasting models have gained popularity as they can utilize hidden feature patterns in time series to improve forecasting performance. Nevertheless, the majority of current deep forecasting models are opaque, hence making it challenging to interpret the results. While counterfactual explanations have been extensively employed as a post-hoc approach for explaining classification models, their application to forecasting models still remains underexplored. In this paper, we formulate the novel problem of counterfactual generation for time series forecasting, and propose an algorithm, called ForecastCF that solves the problem by applying gradient-based perturbations to the original time series. The perturbations are further guided by imposing constraints to the forecasted values. We experimentally evaluate ForecastCF using four state-of-the-art deep model architectures and compare to two baselines. ForecastCF outperforms the baselines in terms of counterfactual validity and data manifold closeness, while generating meaningful and relevant counterfactuals for various forecasting tasks.
更多查看译文
关键词
time series forecasting,counterfactual explanations,model interpretability,deep learning
AI 理解论文
溯源树
样例
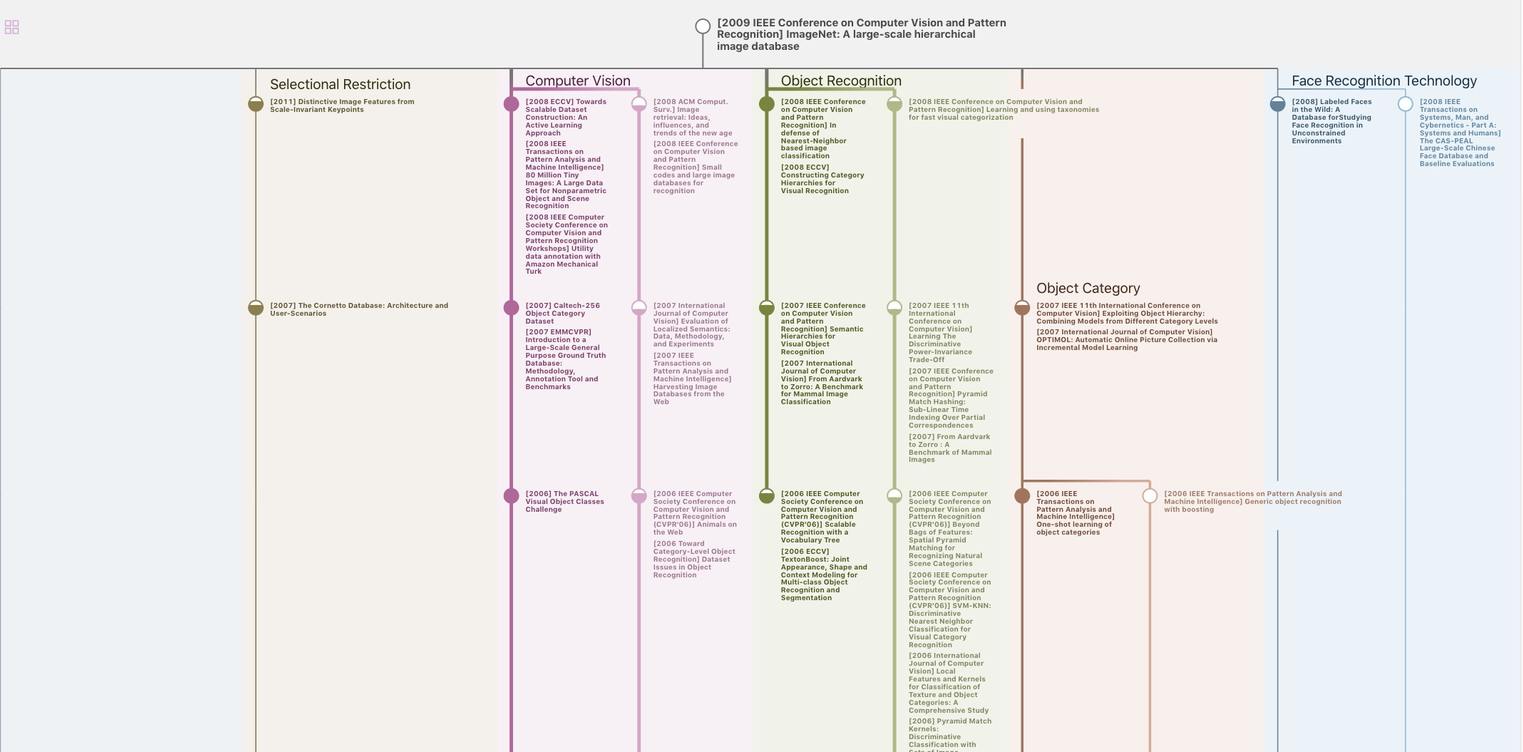
生成溯源树,研究论文发展脉络
Chat Paper
正在生成论文摘要