Model-Agnostic Covariate-Assisted Inference on Partially Identified Causal Effects
arXiv (Cornell University)(2023)
摘要
Many causal estimands are only partially identifiable since they depend on the unobservable joint distribution between potential outcomes. Stratification on pretreatment covariates can yield sharper partial identification bounds; however, unless the covariates are discrete with relatively small support, this approach typically requires consistent estimation of the conditional distributions of the potential outcomes given the covariates. Thus, existing approaches may fail under model misspecification or if consistency assumptions are violated. In this study, we propose a unified and model-agnostic inferential approach for a wide class of partially identified estimands, based on duality theory for optimal transport problems. In randomized experiments, our approach can wrap around any estimates of the conditional distributions and provide uniformly valid inference, even if the initial estimates are arbitrarily inaccurate. Also, our approach is doubly robust in observational studies. Notably, this property allows analysts to use the multiplier bootstrap to select covariates and models without sacrificing validity even if the true model is not included. Furthermore, if the conditional distributions are estimated at semiparametric rates, our approach matches the performance of an oracle with perfect knowledge of the outcome model. Finally, we propose an efficient computational framework, enabling implementation on many practical problems in causal inference.
更多查看译文
关键词
inference,effects,model-agnostic,covariate-assisted
AI 理解论文
溯源树
样例
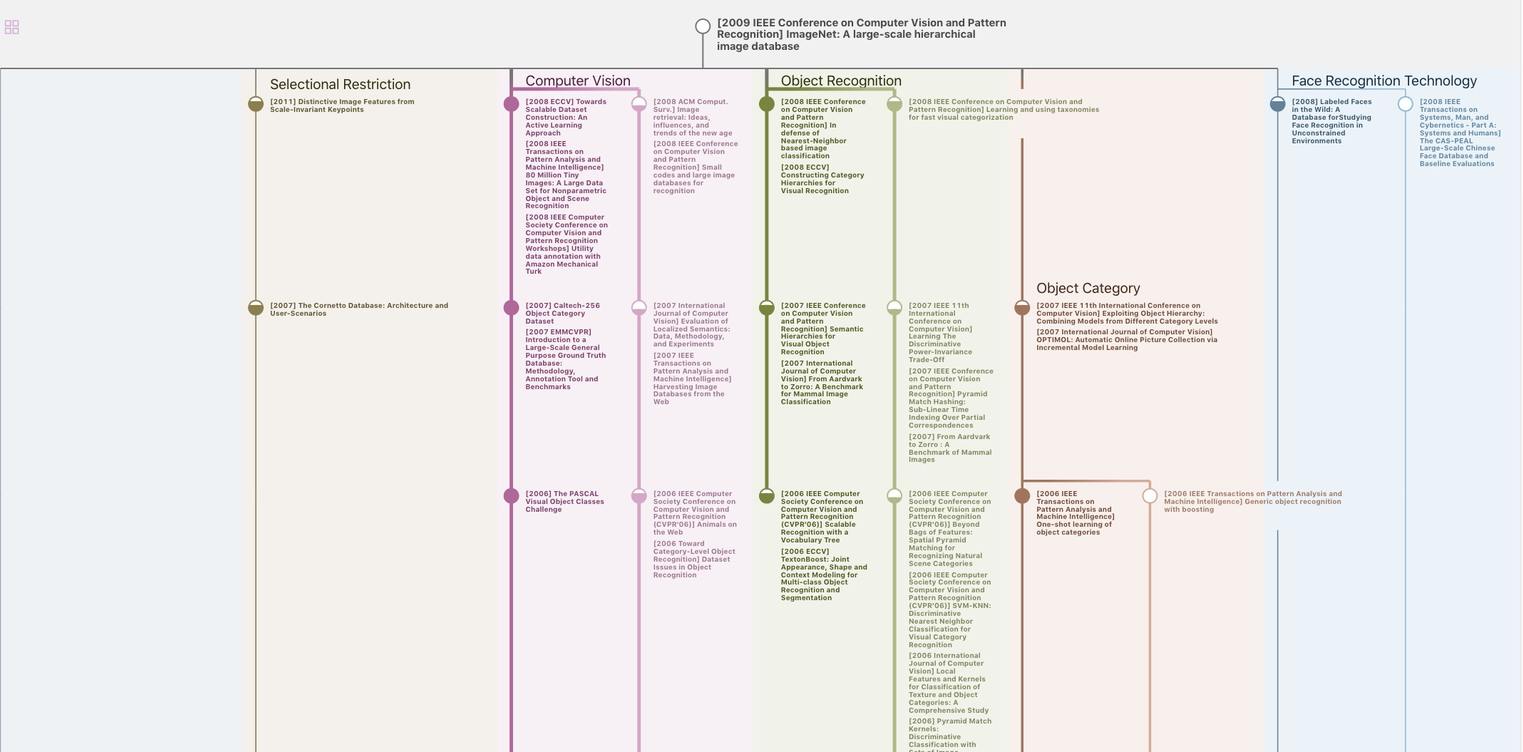
生成溯源树,研究论文发展脉络
Chat Paper
正在生成论文摘要