Learning Regularized Monotone Graphon Mean-Field Games
NeurIPS(2023)
摘要
This paper studies two fundamental problems in regularized Graphon Mean-Field Games (GMFGs). First, we establish the existence of a Nash Equilibrium (NE) of any $\lambda$-regularized GMFG (for $\lambda\geq 0$). This result relies on weaker conditions than those in previous works for analyzing both unregularized GMFGs ($\lambda=0$) and $\lambda$-regularized MFGs, which are special cases of GMFGs. Second, we propose provably efficient algorithms to learn the NE in weakly monotone GMFGs, motivated by Lasry and Lions [2007]. Previous literature either only analyzed continuous-time algorithms or required extra conditions to analyze discrete-time algorithms. In contrast, we design a discrete-time algorithm and derive its convergence rate solely under weakly monotone conditions. Furthermore, we develop and analyze the action-value function estimation procedure during the online learning process, which is absent from algorithms for monotone GMFGs. This serves as a sub-module in our optimization algorithm. The efficiency of the designed algorithm is corroborated by empirical evaluations.
更多查看译文
关键词
games,learning,mean-field
AI 理解论文
溯源树
样例
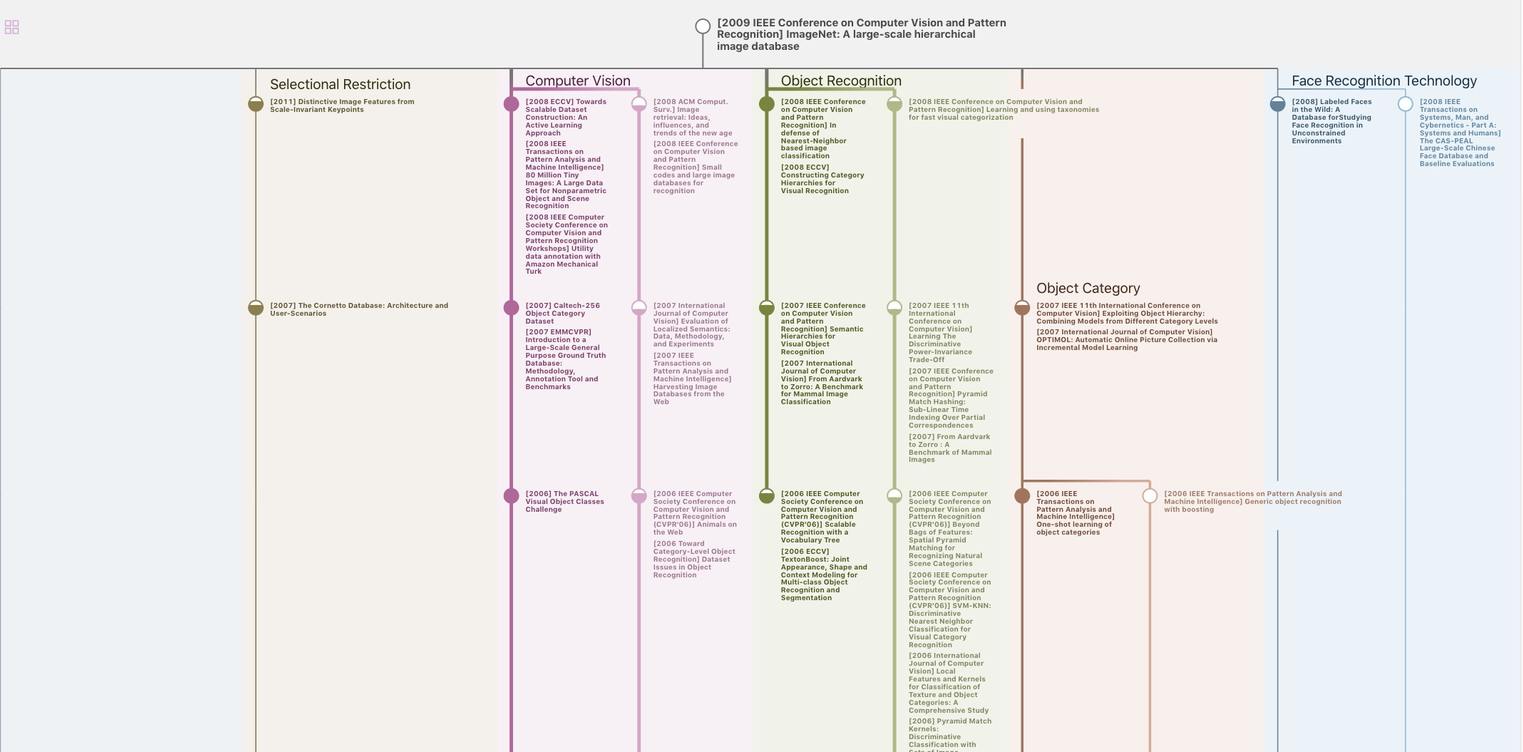
生成溯源树,研究论文发展脉络
Chat Paper
正在生成论文摘要