Samples on Thin Ice: Re-Evaluating Adversarial Pruning of Neural Networks
CoRR(2023)
摘要
Neural network pruning has shown to be an effective technique for reducing the network size, trading desirable properties like generalization and robustness to adversarial attacks for higher sparsity. Recent work has claimed that adversarial pruning methods can produce sparse networks while also preserving robustness to adversarial examples. In this work, we first re-evaluate three state-of-the-art adversarial pruning methods, showing that their robustness was indeed overestimated. We then compare pruned and dense versions of the same models, discovering that samples on thin ice, i.e., closer to the unpruned model's decision boundary, are typically misclassified after pruning. We conclude by discussing how this intuition may lead to designing more effective adversarial pruning methods in future work.
更多查看译文
关键词
adversarial pruning,thin ice,networks,neural
AI 理解论文
溯源树
样例
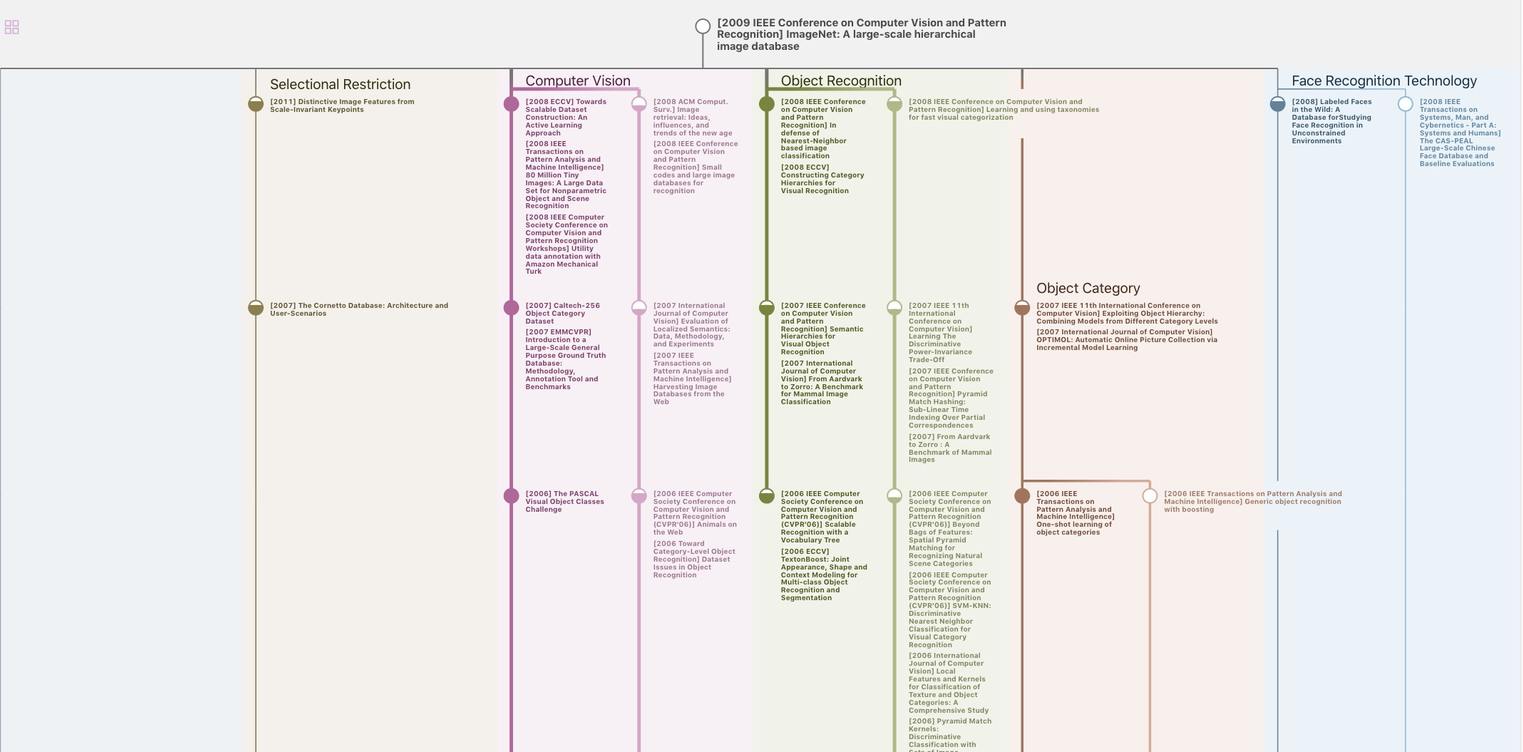
生成溯源树,研究论文发展脉络
Chat Paper
正在生成论文摘要