ZEST: Attention-based Zero-Shot Learning for Unseen IoT Device Classification
CoRR(2023)
摘要
Recent research works have proposed machine learning models for classifying
IoT devices connected to a network. However, there is still a practical
challenge of not having all devices (and hence their traffic) available during
the training of a model. This essentially means, during the operational phase,
we need to classify new devices not seen in the training phase. To address this
challenge, we propose ZEST – a ZSL (zero-shot learning) framework based on
self-attention for classifying both seen and unseen devices. ZEST consists of
i) a self-attention based network feature extractor, termed SANE, for
extracting latent space representations of IoT traffic, ii) a generative model
that trains a decoder using latent features to generate pseudo data, and iii) a
supervised model that is trained on the generated pseudo data for classifying
devices. We carry out extensive experiments on real IoT traffic data; our
experiments demonstrate i) ZEST achieves significant improvement (in terms of
accuracy) over the baselines; ii) SANE is able to better extract meaningful
representations than LSTM which has been commonly used for modeling network
traffic.
更多查看译文
关键词
iot device
AI 理解论文
溯源树
样例
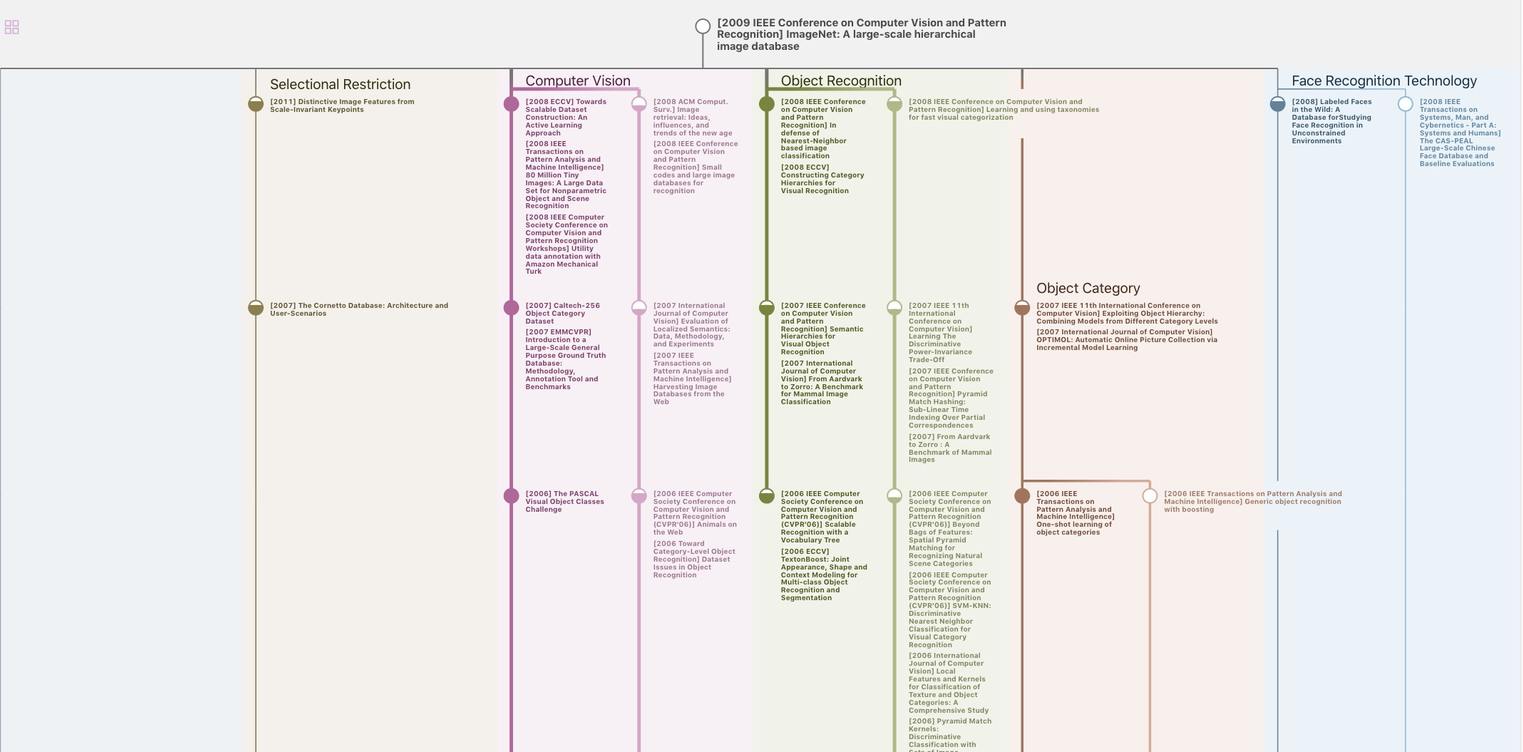
生成溯源树,研究论文发展脉络
Chat Paper
正在生成论文摘要