Exploiting Semantic Localization in Highly Dynamic Wireless Networks Using Deep Homoscedastic Domain Adaptation
arXiv (Cornell University)(2023)
摘要
Localization in GPS-denied outdoor locations, such as street canyons in an urban or metropolitan environment, has many applications. Machine Learning (ML) is widely used to tackle this critical problem. One challenge lies in the mixture of line-of-sight (LOS), obstructed LOS (OLOS), and non-LOS (NLOS) conditions. In this paper, we consider a semantic localization that treats these three propagation conditions as the ''semantic objects", and aims to determine them together with the actual localization, and show that this increases accuracy and robustness. Furthermore, the propagation conditions are highly dynamic, since obstruction by cars or trucks can change the channel state information (CSI) at a fixed location over time. We therefore consider the blockage by such dynamic objects as another semantic state. Based on these considerations, we formulate the semantic localization with a joint task (coordinates regression and semantics classification) learning problem. Another problem created by the dynamics is the fact that each location may be characterized by a number of different CSIs. To avoid the need for excessive amount of labeled training data, we propose a multi-task deep domain adaptation (DA) based localization technique, training neural networks with a limited number of labeled samples and numerous unlabeled ones. Besides, we introduce novel scenario adaptive learning strategies to ensure efficient representation learning and successful knowledge transfer. Finally, we use Bayesian theory for uncertainty modeling of the importance weights in each task, reducing the need for time-consuming parameter finetuning; furthermore, with some mild assumptions, we derive the related log-likelihood for the joint task and present the deep homoscedastic DA based localization method.
更多查看译文
关键词
semantic localization,dynamic wireless networks
AI 理解论文
溯源树
样例
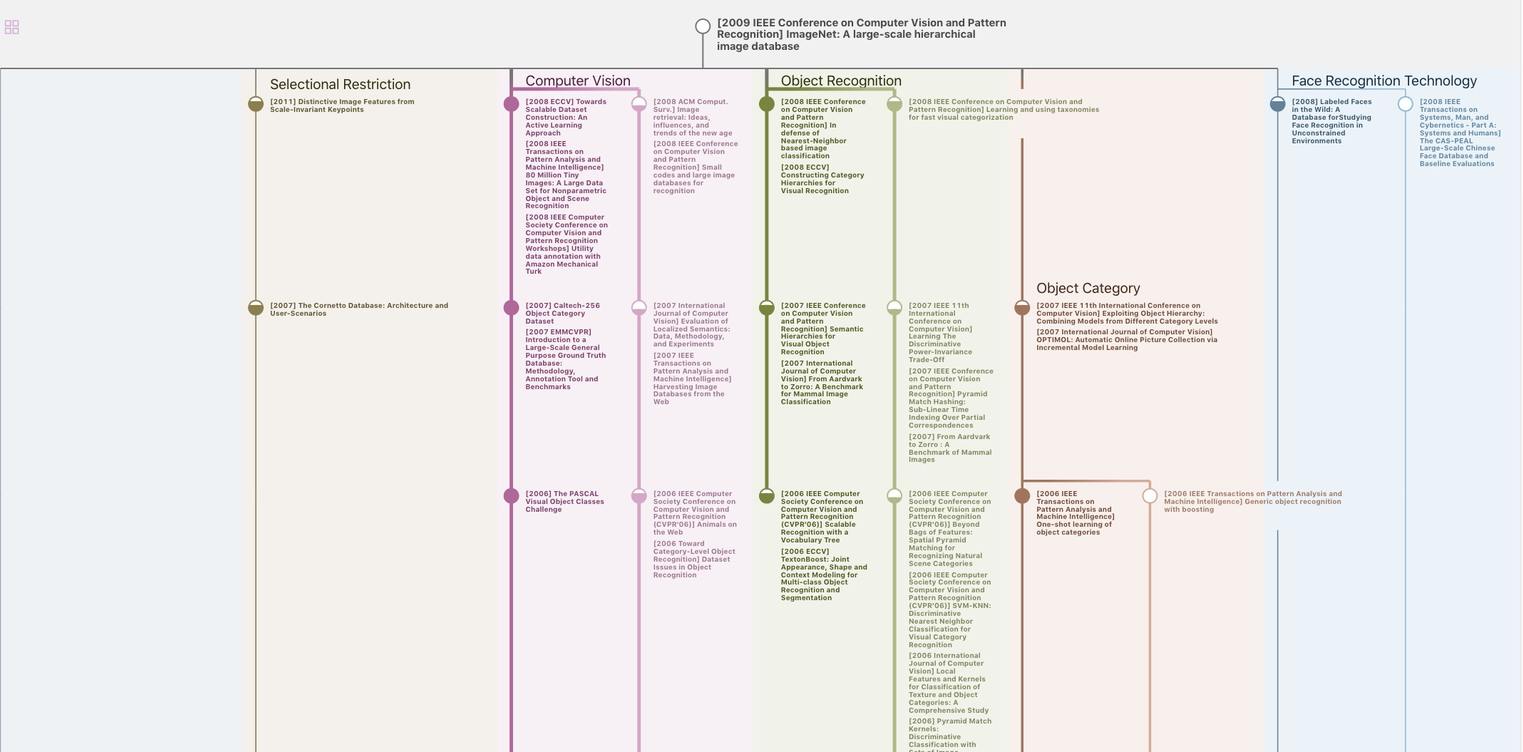
生成溯源树,研究论文发展脉络
Chat Paper
正在生成论文摘要