Extreme Image Transformations Facilitate Robust Latent Object Representations
CoRR(2023)
摘要
Adversarial attacks can affect the object recognition capabilities of machines in wild. These can often result from spurious correlations between input and class labels, and are prone to memorization in large networks. While networks are expected to do automated feature selection, it is not effective at the scale of the object. Humans, however, are able to select the minimum set of features required to form a robust representation of an object. In this work, we show that finetuning any pretrained off-the-shelf network with Extreme Image Transformations (EIT) not only helps in learning a robust latent representation, it also improves the performance of these networks against common adversarial attacks of various intensities. Our EIT trained networks show strong activations in the object regions even when tested with more intense noise, showing promising generalizations across different kinds of adversarial attacks.
更多查看译文
关键词
representations,image
AI 理解论文
溯源树
样例
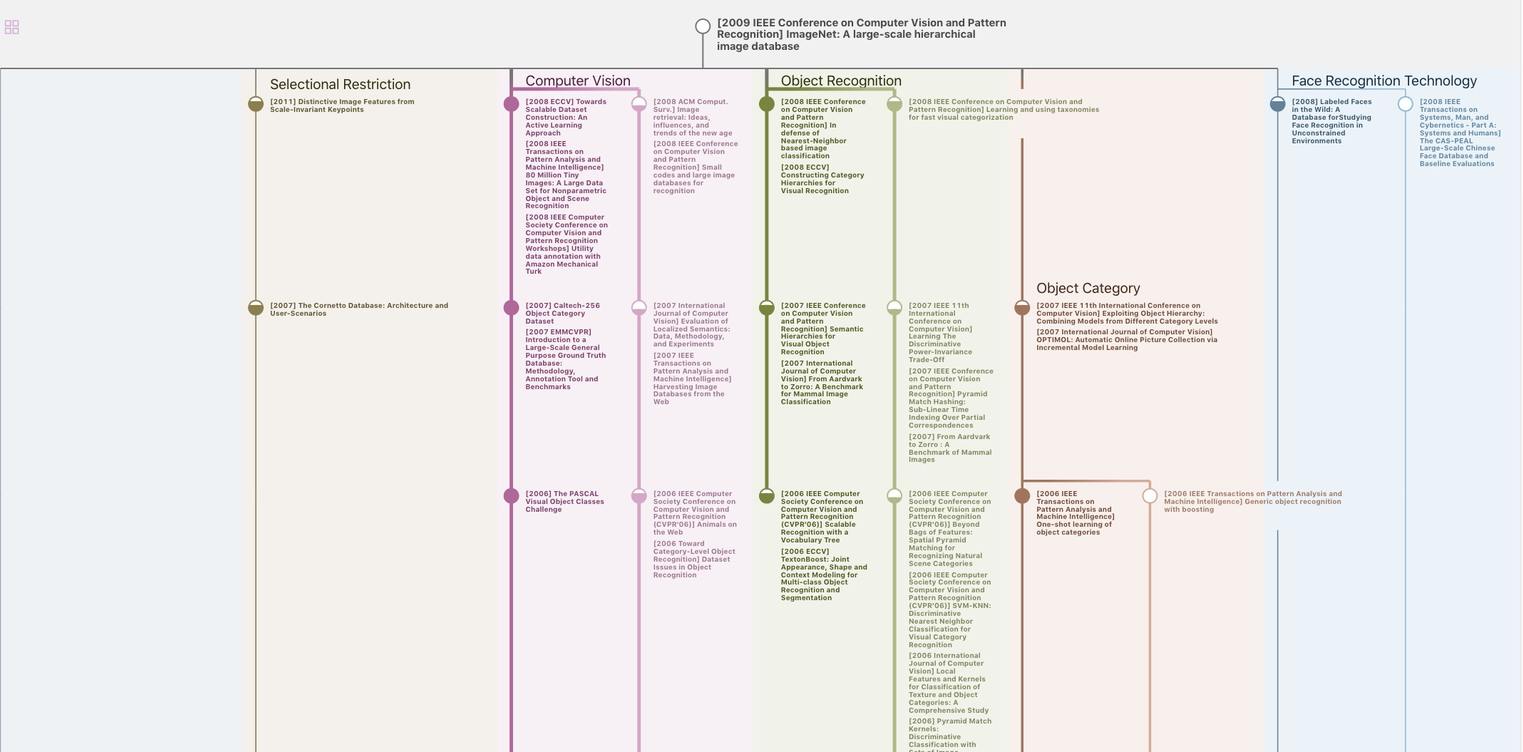
生成溯源树,研究论文发展脉络
Chat Paper
正在生成论文摘要