TradeFL: A Trading Mechanism for Cross-Silo Federated Learning
2023 IEEE 43rd International Conference on Distributed Computing Systems (ICDCS)(2023)
摘要
Cross-silo federated learning (CFL) is a distributed learning paradigm that allows organizations (e.g., financial or medical entities) to train a global model on siloed data. Recent studies on mechanisms designed for CFL, however, rarely jointly consider the potential inter-organizational competition and the lack of credibility between organizations, which may discourage organizational participation. In this paper, we investigate the problem of inter-organizational competition and credibility assurance. We propose a distributed trading mechanism, called
$TradeFL$
, to incentivize organizations to contribute data and computational resources through mutual trading among organizations. Technically, TradeFL characterizes the competition among organizations and compensates for their damage incurred by competition. TradeFL runs on distributed organizations and provides credibility guarantees for compensation through a customized smart contract
1
1
Illustration of the prototype: https://github.com/user10963.. We prove that the interaction among organizations that contribute resources to maximize personal payoffs is a weighted potential game. Then, we propose a centralized algorithm and a distributed algorithm to determine the optimal resource contribution. Simulation results and evaluations based on real-world datasets demonstrate that our scheme achieves higher social welfare, increases the amount of contributed data by up to 64%, and improves the accuracy of the global model by at most 23.2%.
更多查看译文
关键词
Trading mechanism, federated learning, potential game, smart-contract
AI 理解论文
溯源树
样例
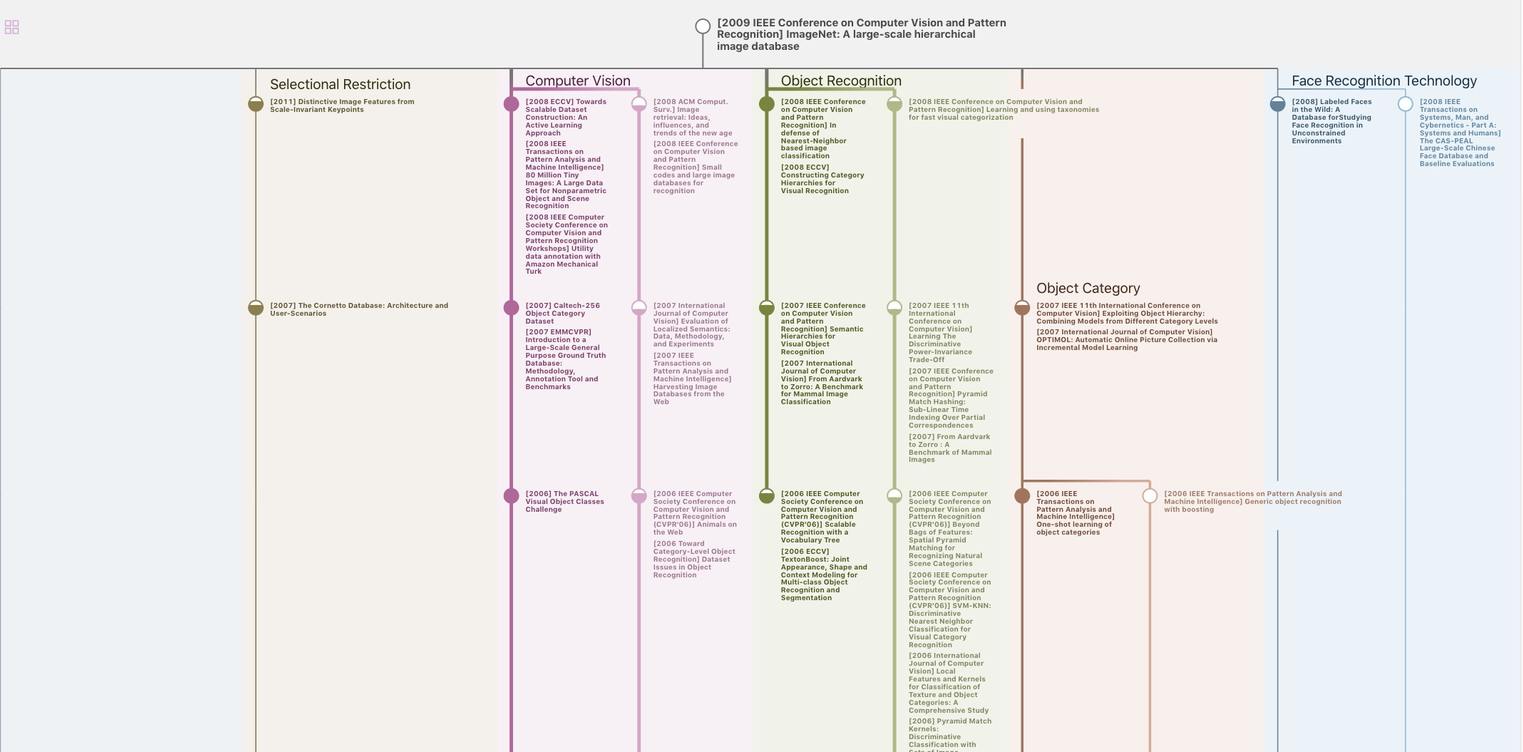
生成溯源树,研究论文发展脉络
Chat Paper
正在生成论文摘要