Evaluation of Query-Based Membership Inference Attack on the Medical Data
PROCEEDINGS OF THE 2023 ACM SOUTHEAST CONFERENCE, ACMSE 2023(2023)
摘要
In recent years, machine learning (ML) has achieved huge success in healthcare and medicine areas. However, recent work has demonstrated that ML is vulnerable to privacy leakage since it exhibits to overfit the training datasets. Especially, in healthcare and medical communities, there are concerns that medical images and electronic health records containing protected health information (PHI) are vulnerable to inference attacks. These PHI might be unwittingly leaked when the aforementioned data is used for training ML models to address necessary healthcare concerns. Given access to the trained ML model, the attacker is able to adopt membership inference attacks (MIA) to determine whether a specific data sample is used in the corresponding medical training dataset. In this paper, we concentrate on MIA and propose a new method to determine whether a sample was used to train the given ML model or not. Our method is based on the observation that a trained machine learning model usually is lesser sensitive to the feature value perturbations on its training samples compared with the non-training samples. The key idea of our method is to perturb a training sample's feature value in the corresponding feature space and then compute the relationship between each feature's perturbation and the corresponding prediction's change as features to train the attack model. We used publicly available medical datasets such as diabetes and heartbeat categorization data to evaluate our method. Our evaluation shows that the proposed attack can perform better than the existing membership inference attack method.
更多查看译文
关键词
Machine Learning,prediction sensitivity,Jacobian matrix,membership inference attack
AI 理解论文
溯源树
样例
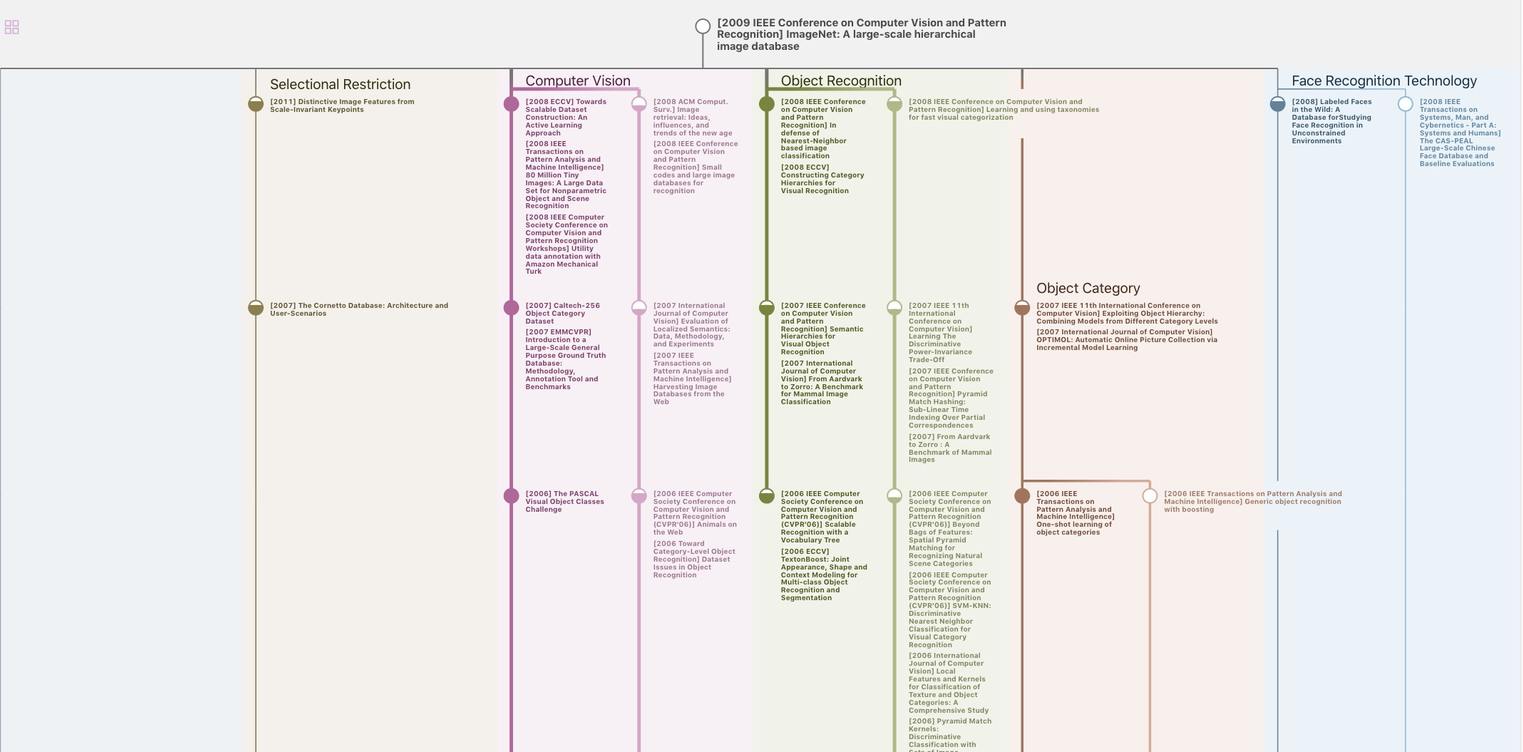
生成溯源树,研究论文发展脉络
Chat Paper
正在生成论文摘要