Controllable Data Generation Via Iterative Data-Property Mutual Mappings
CoRR(2023)
摘要
Deep generative models have been widely used for their ability to generate realistic data samples in various areas, such as images, molecules, text, and speech. One major goal of data generation is controllability, namely to generate new data with desired properties. Despite growing interest in the area of controllable generation, significant challenges still remain, including 1) disentangling desired properties with unrelated latent variables, 2) out-of-distribution property control, and 3) objective optimization for out-of-distribution property control. To address these challenges, in this paper, we propose a general framework to enhance VAE-based data generators with property controllability and ensure disentanglement. Our proposed objective can be optimized on both data seen and unseen in the training set. We propose a training procedure to train the objective in a semi-supervised manner by iteratively conducting mutual mappings between the data and properties. The proposed framework is implemented on four VAE-based controllable generators to evaluate its performance on property error, disentanglement, generation quality, and training time. The results indicate that our proposed framework enables more precise control over the properties of generated samples in a short training time, ensuring the disentanglement and keeping the validity of the generated samples.
更多查看译文
关键词
data-property
AI 理解论文
溯源树
样例
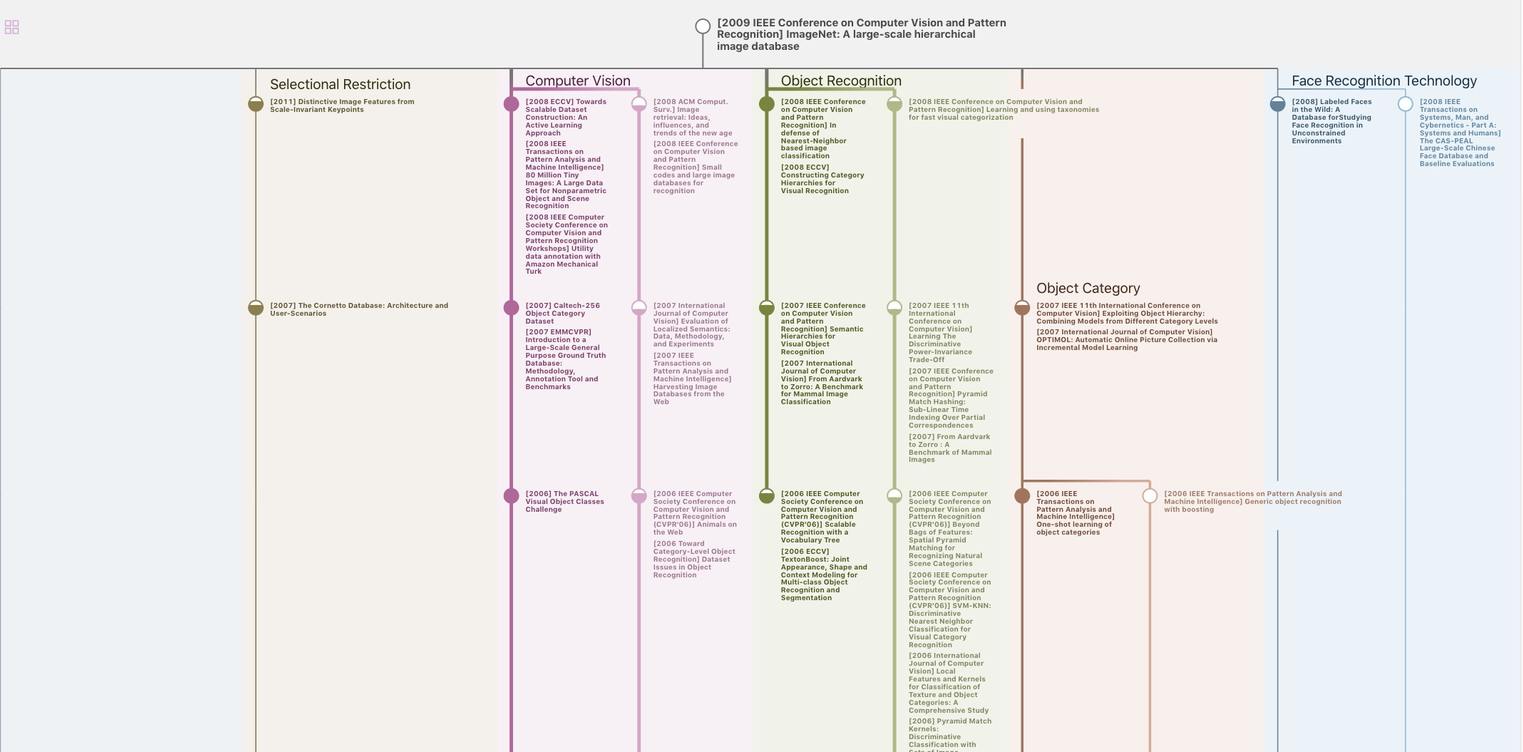
生成溯源树,研究论文发展脉络
Chat Paper
正在生成论文摘要