Split Knockoffs for Multiple Comparisons: Controlling the Directional False Discovery Rate
JOURNAL OF THE AMERICAN STATISTICAL ASSOCIATION(2024)
摘要
Multiple comparisons in hypothesis testing often encounter structural constraints in various applications. For instance, in structural Magnetic Resonance Imaging for Alzheimer's Disease, the focus extends beyond examining atrophic brain regions to include comparisons of anatomically adjacent regions. These constraints can be modeled as linear transformations of parameters, where the sign patterns play a crucial role in estimating directional effects. This class of problems, encompassing total variations, wavelet transforms, fused LASSO, trend filtering, and more, presents an open challenge in effectively controlling the directional false discovery rate. In this article, we propose an extended Split Knockoff method specifically designed to address the control of directional false discovery rate under linear transformations. Our proposed approach relaxes the stringent linear manifold constraint to its neighborhood, employing a variable splitting technique commonly used in optimization. This methodology yields an orthogonal design that benefits both power and directional false discovery rate control. By incorporating a sample splitting scheme, we achieve effective control of the directional false discovery rate, with a notable reduction to zero as the relaxed neighborhood expands. To demonstrate the efficacy of our method, we conduct simulation experiments and apply it to two real-world scenarios: Alzheimer's Disease analysis and human age comparisons. Supplementary materials for this article are available online.
更多查看译文
关键词
Alzheimer's disease,Multiple comparison hypothesis test,Pairwise comparison,Transformational sparsity,Variable splitting
AI 理解论文
溯源树
样例
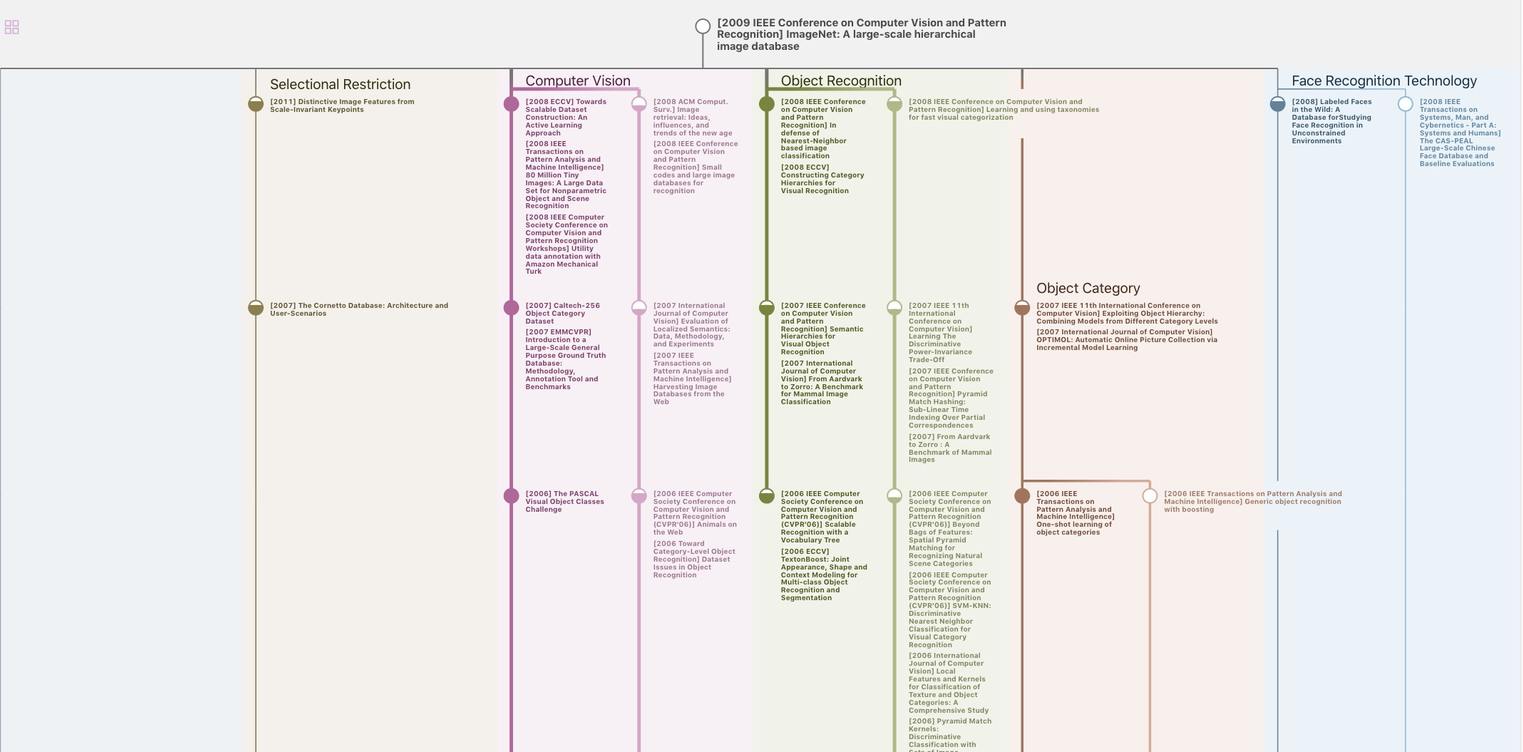
生成溯源树,研究论文发展脉络
Chat Paper
正在生成论文摘要