Event Generator Tuning Incorporating Systematic Uncertainty
arXiv (Cornell University)(2023)
摘要
Event generators play an important role in all physics programs at the Large Hadron Collider and beyond. Dedicated efforts are required to tune the parameters of event generators to accurately describe data. There are many tuning methods ranging from expert-based manual tuning to surrogate function-based semi-automatic tuning, to machine learning-based re-weighting. Although they scale differently with the number of generator parameters and the number of experimental observables, these methods are effective in finding optimal generator parameters. However, none of these tuning methods includes the Monte Carlo (MC) systematic uncertainties. That makes the tuning results sensitive to systematic variations. In this work, we introduce a novel method to incorporate the MC systematic uncertainties into the tuning procedure and to quantitatively evaluate uncertainties associated with the tuned parameters. Tested with a dummy example, the method results in a $\chi^2$ distribution that is centered around one, the optimal generator parameters are closer to the true parameters, and the estimated uncertainties are more accurate.
更多查看译文
关键词
systematic uncertainty,event
AI 理解论文
溯源树
样例
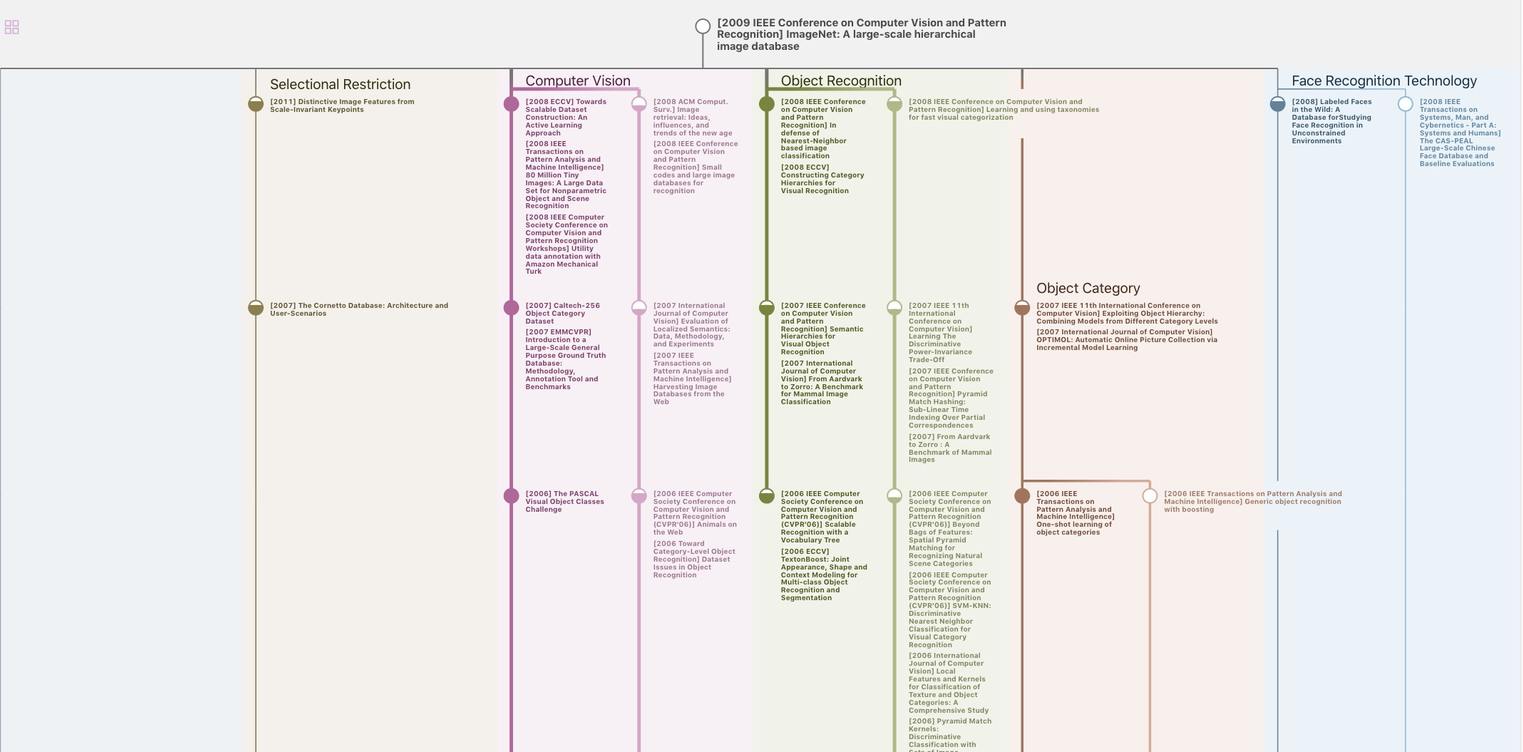
生成溯源树,研究论文发展脉络
Chat Paper
正在生成论文摘要