Fast Sampling and Inference via Preconditioned Langevin Dynamics
arXiv (Cornell University)(2023)
摘要
Sampling from distributions play a crucial role in aiding practitioners with statistical inference. However, in numerous situations, obtaining exact samples from complex distributions is infeasible. Consequently, researchers often turn to approximate sampling techniques to address this challenge. Fast approximate sampling from complicated distributions has gained much traction in the last few years with considerable progress in this field. Previous work has shown that for some problems a preconditioning can make the algorithm faster. In our research, we explore the Langevin Monte Carlo (LMC) algorithm and demonstrate its effectiveness in enabling inference from the obtained samples. Additionally, we establish a convergence rate for the LMC Markov chain in total variation. Lastly, we derive non-asymptotic bounds for approximate sampling from specific target distributions in the Wasserstein distance, particularly when the preconditioning is spatially invariant.
更多查看译文
关键词
preconditioned langevin dynamics,fast sampling,inference
AI 理解论文
溯源树
样例
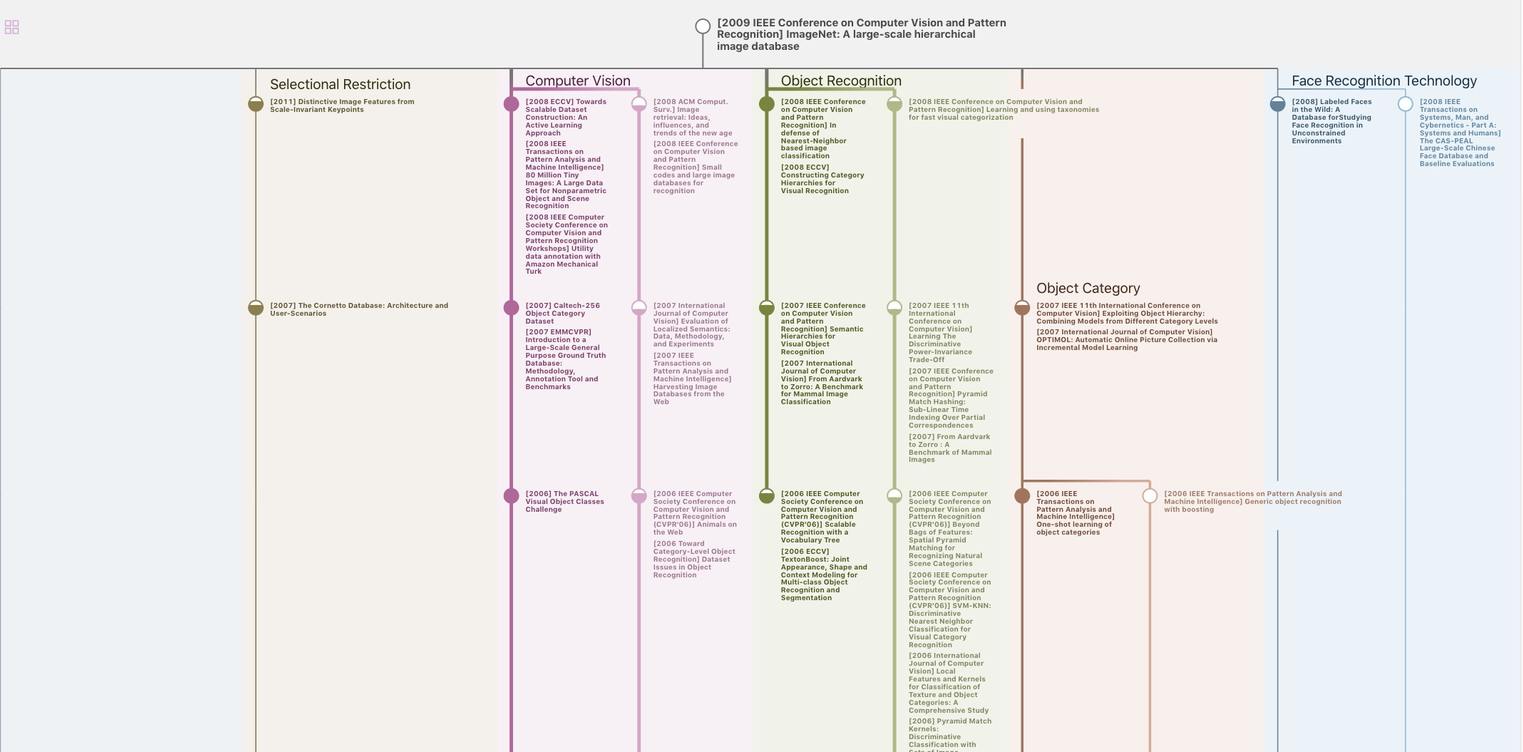
生成溯源树,研究论文发展脉络
Chat Paper
正在生成论文摘要