Leveraging Hierarchical Feature Sharing for Efficient Dataset Condensation
CoRR(2023)
摘要
Given a real-world dataset, data condensation (DC) aims to synthesize a significantly smaller dataset that captures the knowledge of this dataset for model training with high performance. Recent works propose to enhance DC with data parameterization, which condenses data into parameterized data containers rather than pixel space. The intuition behind data parameterization is to encode shared features of images to avoid additional storage costs. In this paper, we recognize that images share common features in a hierarchical way due to the inherent hierarchical structure of the classification system, which is overlooked by current data parameterization methods. To better align DC with this hierarchical nature and encourage more efficient information sharing inside data containers, we propose a novel data parameterization architecture, Hierarchical Memory Network (HMN). HMN stores condensed data in a three-tier structure, representing the dataset-level, class-level, and instance-level features. Another helpful property of the hierarchical architecture is that HMN naturally ensures good independence among images despite achieving information sharing. This enables instance-level pruning for HMN to reduce redundant information, thereby further minimizing redundancy and enhancing performance. We evaluate HMN on four public datasets (SVHN, CIFAR10, CIFAR100, and Tiny-ImageNet) and compare HMN with eight DC baselines. The evaluation results show that our proposed method outperforms all baselines, even when trained with a batch-based loss consuming less GPU memory.
更多查看译文
关键词
hierarchical feature sharing,efficient dataset
AI 理解论文
溯源树
样例
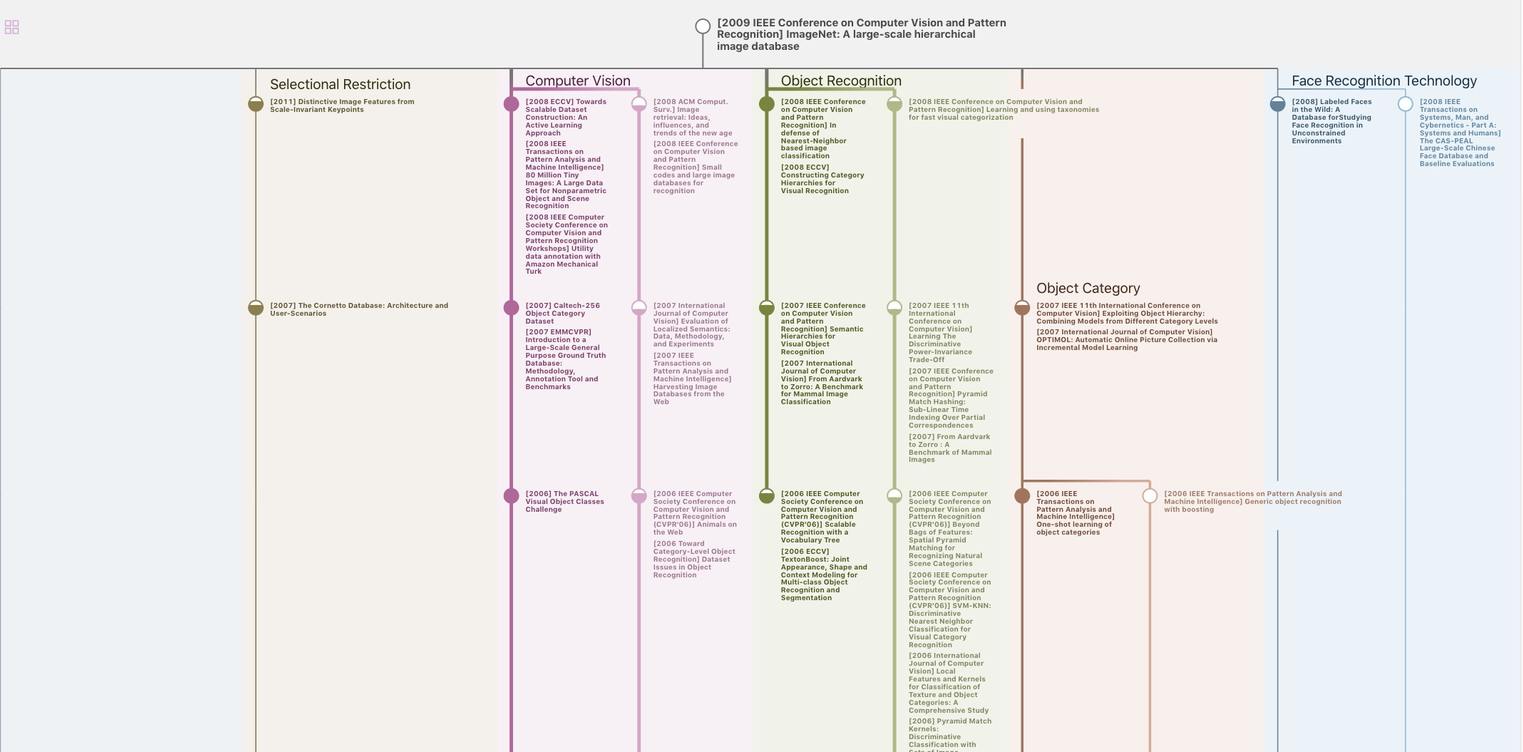
生成溯源树,研究论文发展脉络
Chat Paper
正在生成论文摘要