What can knowledge graph alignment gain with Neuro-Symbolic learning approaches?
CoRR(2023)
摘要
Knowledge Graphs (KG) are the backbone of many data-intensive applications since they can represent data coupled with its meaning and context. Aligning KGs across different domains and providers is necessary to afford a fuller and integrated representation. A severe limitation of current KG alignment (KGA) algorithms is that they fail to articulate logical thinking and reasoning with lexical, structural, and semantic data learning. Deep learning models are increasingly popular for KGA inspired by their good performance in other tasks, but they suffer from limitations in explainability, reasoning, and data efficiency. Hybrid neurosymbolic learning models hold the promise of integrating logical and data perspectives to produce high-quality alignments that are explainable and support validation through human-centric approaches. This paper examines the current state of the art in KGA and explores the potential for neurosymbolic integration, highlighting promising research directions for combining these fields.
更多查看译文
关键词
graph alignment gain,knowledge,learning
AI 理解论文
溯源树
样例
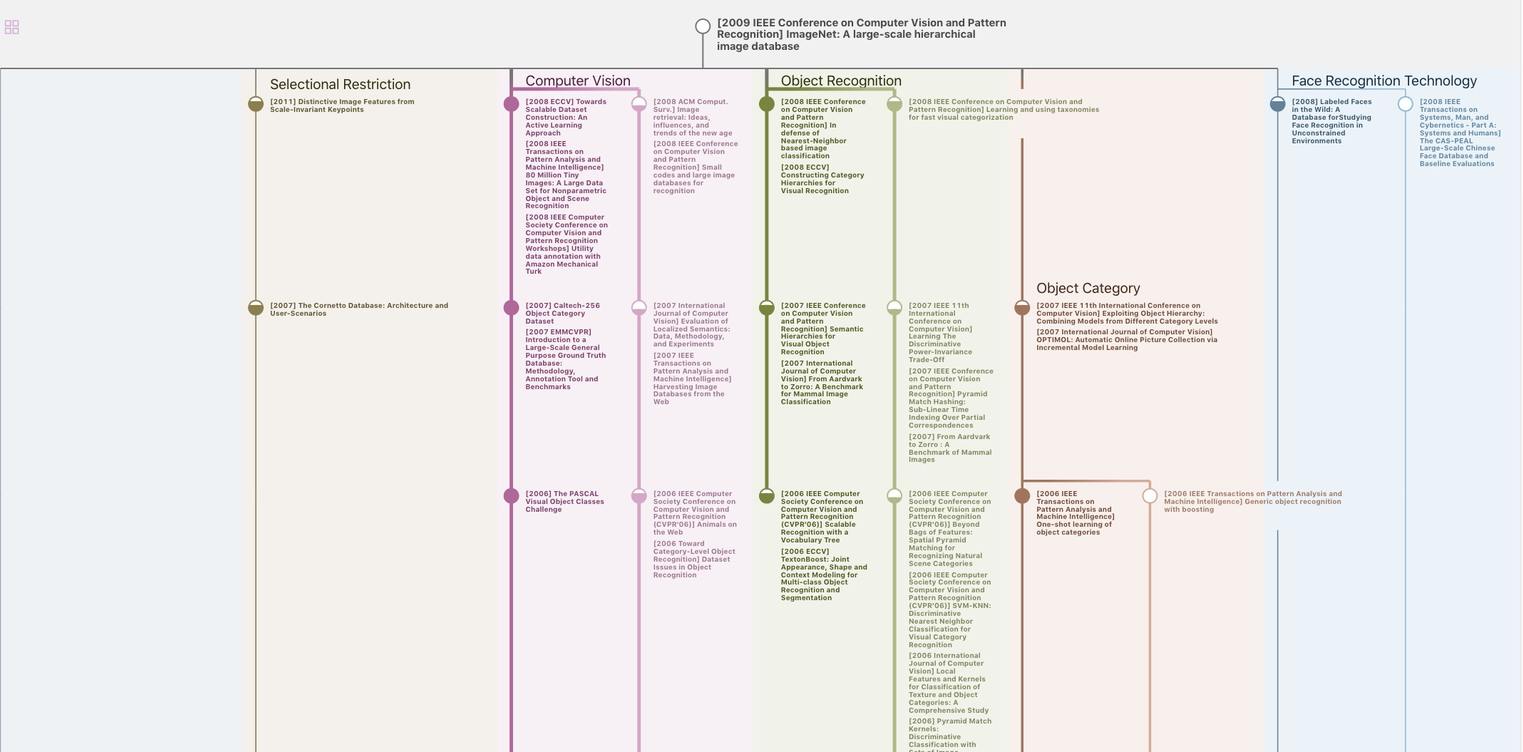
生成溯源树,研究论文发展脉络
Chat Paper
正在生成论文摘要