Bidirectional Recurrent Imputation and Abundance Estimation of LULC Classes With MODIS Multispectral Time-Series and Geo-Topographic and Climatic Data
IEEE JOURNAL OF SELECTED TOPICS IN APPLIED EARTH OBSERVATIONS AND REMOTE SENSING(2024)
摘要
Remotely sensed data are dominated by mixed land use and land cover (LULC) types. Spectral unmixing (SU) is a key technique that disentangles mixed pixels into constituent LULC types and their abundance fractions. While existing studies on deep learning (DL) for SU typically focus on single time-step hyperspectral or multispectral data, our work pioneers SU using MODIS MS time series, addressing missing data with end-to-end DL models. Our approach enhances a long-short-term-memory-based model by incorporating geographic, topographic (geo-topographic), and climatic ancillary information. Notably, our method eliminates the need for explicit endmember extraction, instead learning the input-output relationship between mixed spectra and LULC abundances through supervised learning. Experimental results demonstrate that integrating spectral-temporal input data with geo-topographic and climatic information significantly improves the estimation of LULC abundances in mixed pixels. To facilitate this study, we curated a novel labeled dataset for Andalusia (Spain) with monthly MODIS MS time series at 460-m resolution for 2013. Named Andalusia MultiSpectral MultiTemporal Unmixing, this dataset provides pixel-level annotations of LULC abundances along with ancillary information.
更多查看译文
关键词
Abundance estimation,bidirectional long-short term memory (LSTM),climatic data,deep learning (DL),geo-topographic data,land use and land cover (LULC),missing values,spectral unmixing (SU)
AI 理解论文
溯源树
样例
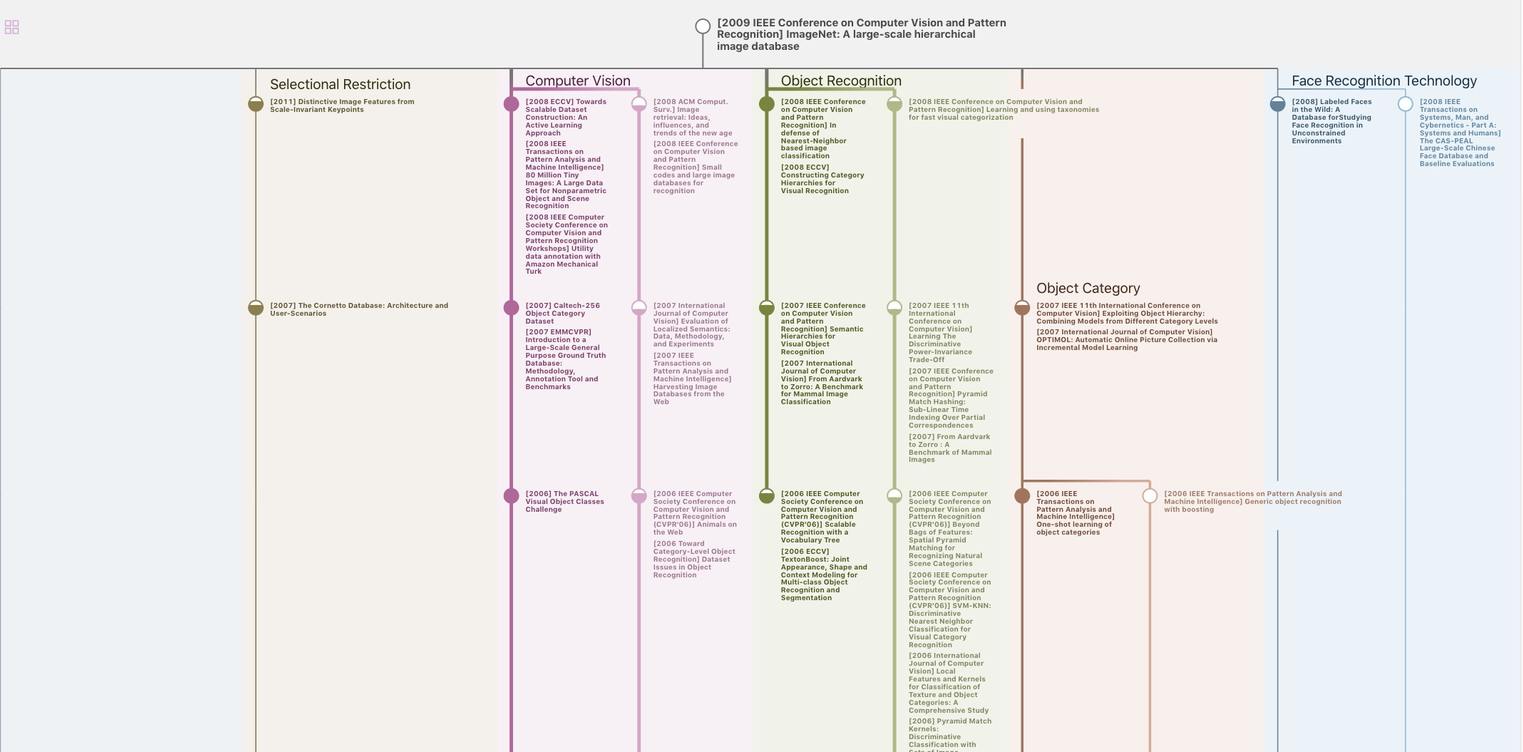
生成溯源树,研究论文发展脉络
Chat Paper
正在生成论文摘要