Imitation Learning from Purified Demonstration
CoRR(2023)
摘要
Imitation learning has emerged as a promising approach for addressing sequential decision-making problems, with the assumption that expert demonstrations are optimal. However, in real-world scenarios, expert demonstrations are often imperfect, leading to challenges in effectively applying imitation learning. While existing research has focused on optimizing with imperfect demonstrations, the training typically requires a certain proportion of optimal demonstrations to guarantee performance. To tackle these problems, we propose to purify the potential perturbations in imperfect demonstrations and subsequently conduct imitation learning from purified demonstrations. Motivated by the success of diffusion models, we introduce a two-step purification via the diffusion process. In the first step, we apply a forward diffusion process to effectively smooth out the potential perturbations in imperfect demonstrations by introducing additional noise. Subsequently, a reverse generative process is utilized to recover the optimal expert demonstrations from the diffused ones. We provide theoretical evidence supporting our approach, demonstrating that total variance distance between the purified and optimal demonstration distributions can be upper-bounded. The evaluation results on MuJoCo demonstrate the effectiveness of our method from different aspects.
更多查看译文
关键词
imitation,purified demonstration,learning
AI 理解论文
溯源树
样例
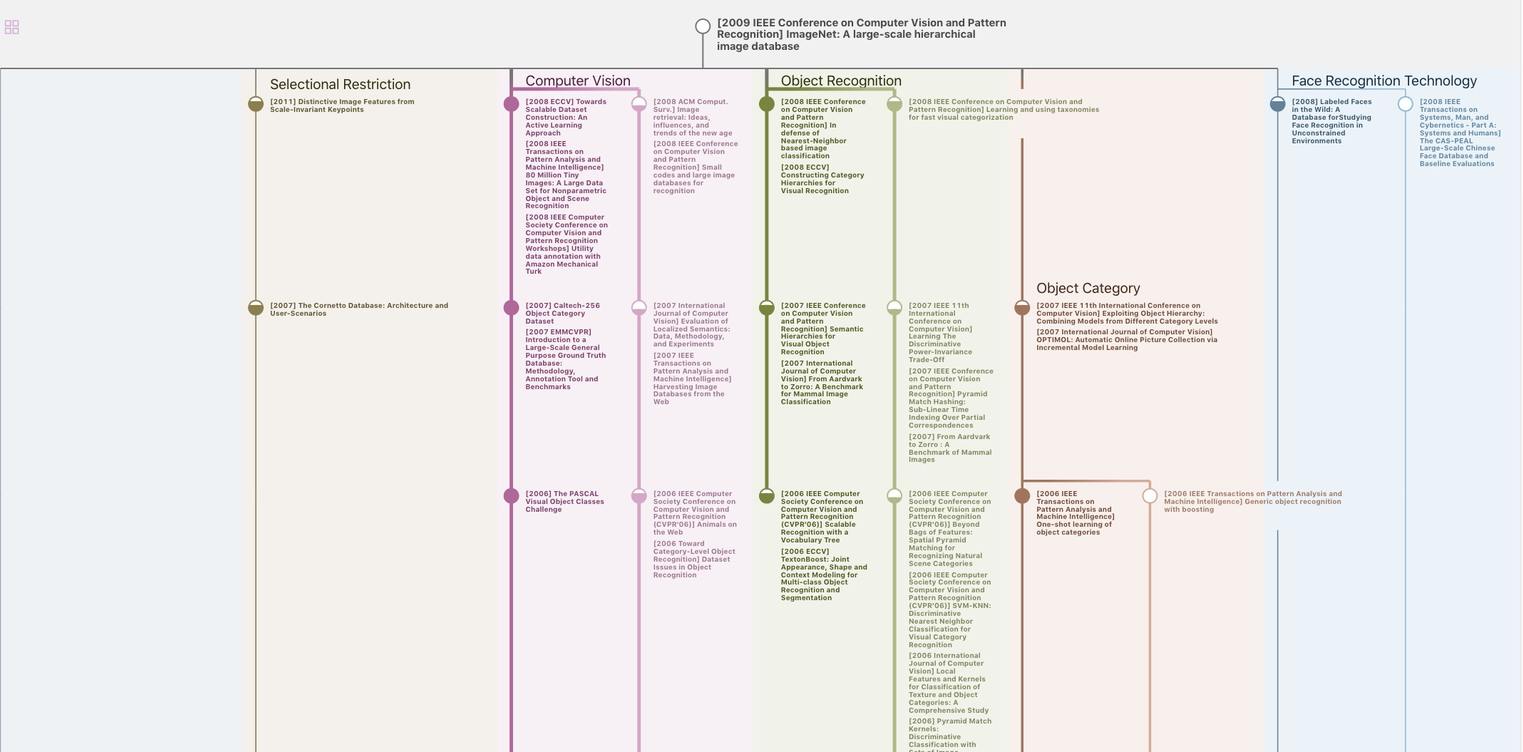
生成溯源树,研究论文发展脉络
Chat Paper
正在生成论文摘要