Diversity of Thought Improves Reasoning Abilities of LLMs
CoRR(2023)
摘要
Large language models (LLMs) are documented to struggle in settings that
require complex reasoning. Nevertheless, instructing the model to break down
the problem into smaller reasoning steps, or ensembling various generations
through modifying decoding steps boosts performance. However, these methods
assume that the input prompt is fixed and expect the decoding strategies to
introduce the diversity needed for ensembling. In this work, we discuss how one
can create and leverage variations of the input prompt as a means of diversity
of thought. We propose a method that automatically improves prompt diversity by
soliciting feedback from the LLM to ideate approaches that are apt for the
problem. We then ensemble the diverse prompts in our method DIVSE (DIVerse
reasoning path Self-Ensemble) across multiple inference calls, or use diverse
approaches within a single inference call; we call the latter IDIV-SE (In-call
DIVerse reasoning path Self-Ensemble). Apart from our approaches outperforming
prior work, DIV-SE(in particular) advances state-of-the-art performance on the
challenging planning and graph coloring benchmarks. Our results improve the
Pareto frontier of the accuracy-cost trade-off.
更多查看译文
关键词
reasoning abilities,large language
AI 理解论文
溯源树
样例
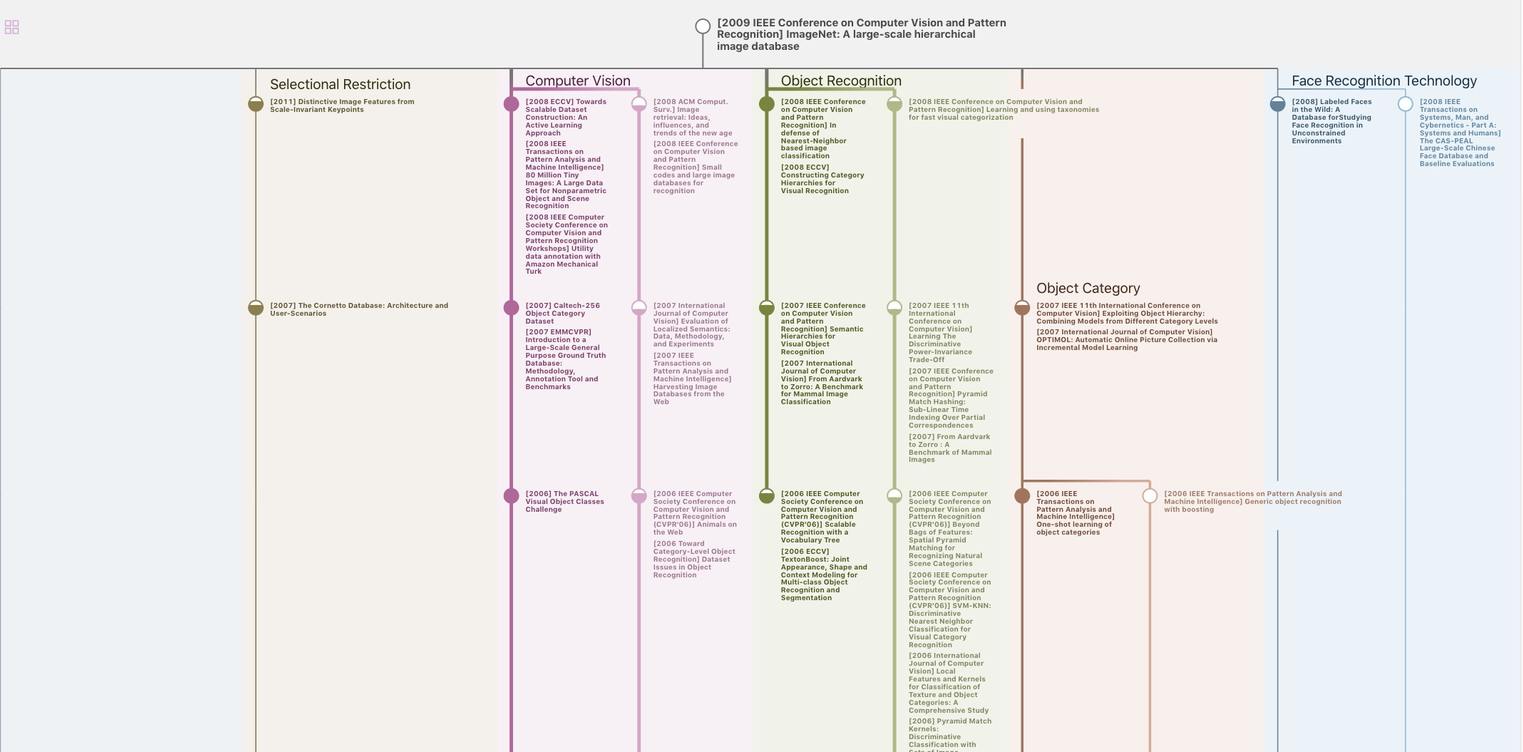
生成溯源树,研究论文发展脉络
Chat Paper
正在生成论文摘要