Utilizing Synthetic Data for Medical Vision-Language Pre-training: Bypassing the Need for Real Images
CoRR(2023)
摘要
Medical Vision-Language Pre-training (VLP) learns representations jointly from medical images and paired radiology reports. It typically requires large-scale paired image-text datasets to achieve effective pre-training for both the image encoder and text encoder. The advent of text-guided generative models raises a compelling question: Can VLP be implemented solely with synthetic images generated from genuine radiology reports, thereby mitigating the need for extensively pairing and curating image-text datasets? In this work, we scrutinize this very question by examining the feasibility and effectiveness of employing synthetic images for medical VLP. We replace real medical images with their synthetic equivalents, generated from authentic medical reports. Utilizing three state-of-the-art VLP algorithms, we exclusively train on these synthetic samples. Our empirical evaluation across three subsequent tasks, namely image classification, semantic segmentation and object detection, reveals that the performance achieved through synthetic data is on par with or even exceeds that obtained with real images. As a pioneering contribution to this domain, we introduce a large-scale synthetic medical image dataset, paired with anonymized real radiology reports. This alleviates the need of sharing medical images, which are not easy to curate and share in practice. The code and the dataset will be made publicly available upon paper acceptance.
更多查看译文
关键词
synthetic data,real images,vision-language,pre-training
AI 理解论文
溯源树
样例
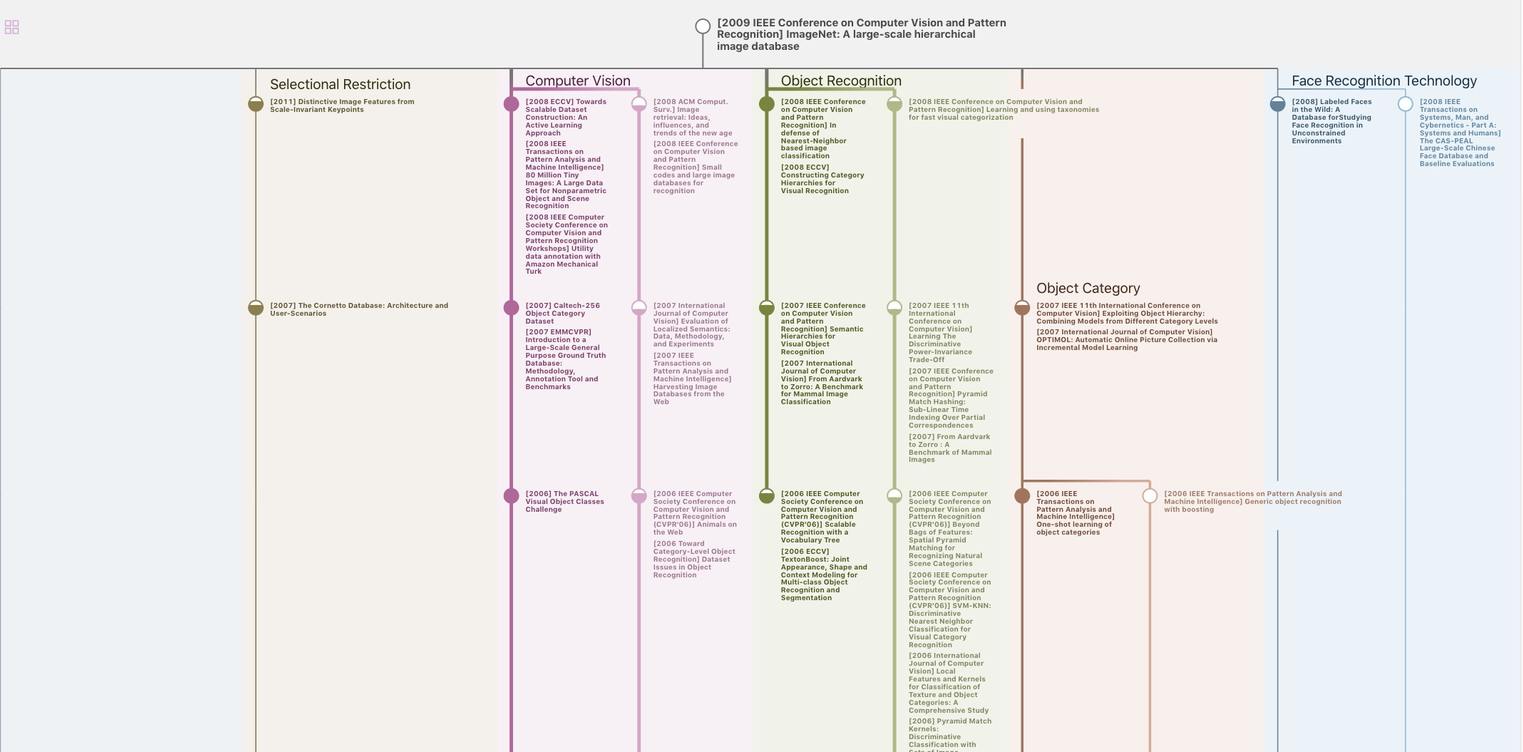
生成溯源树,研究论文发展脉络
Chat Paper
正在生成论文摘要