Land Use and Land Cover Change Detection Using the Random Forest Approach: The Case of The Upper Blue Nile River Basin, Ethiopia
Global Challenges(2023)
摘要
Abstract Monitoring land use change dynamics is critical for tackling food security, climate change, and biodiversity loss on a global scale. This study is designed to classify land use and land cover in the upper Blue Nile River Basin (BNRB) using a random forest (RF) algorithm. The Landsat images for Landsat 45, Landsat 7, and Landsat 8 are used for classification purposes. The study area is classified into seven land use/land cover classes: cultivated lands, bare lands, built‐ups, forests, grazing lands, shrublands, and waterbodies. The accuracy of classified images is 83%, 85%, and 91% using the Kappa index of agreements. From 1983 to 2022 periods, cultivated lands and built‐up areas increased by 47541 and 1777 km2, respectively, at the expense of grazing lands, shrublands, and forests. Furthermore, the area of water bodies has increased by 662 km2 due to the construction of small and large‐scale irrigation and hydroelectric power generation dams. The main factors that determine agricultural land expansion are related to population growth. Therefore, land use and land cover change detection using a random forest is an important technique for multispectral satellite data classification to understand the optimal use of natural resources, conservation practices, and decision‐making for sustainable development.
更多查看译文
关键词
change detection,land use/land cover,landsat,random forest,upper Blue Nile River basin
AI 理解论文
溯源树
样例
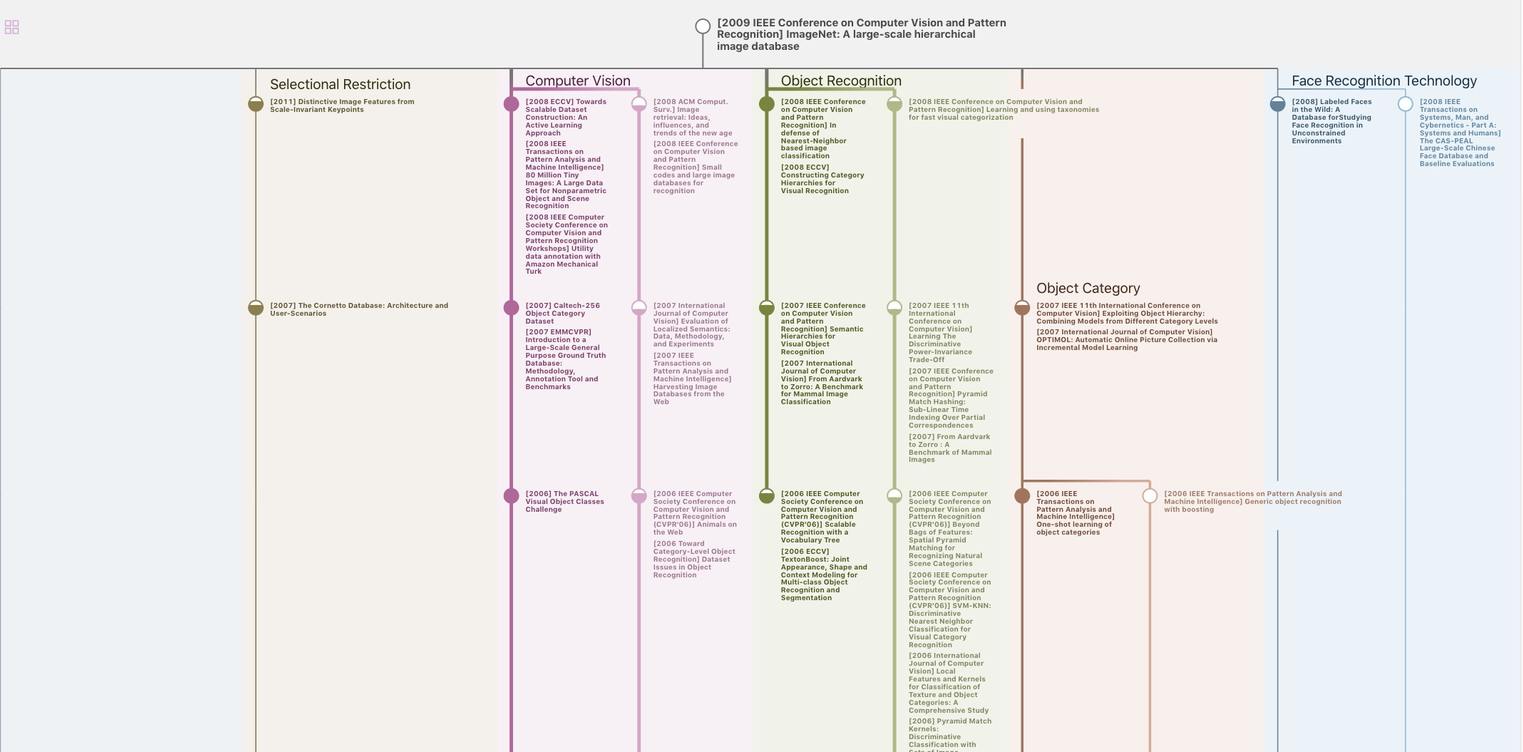
生成溯源树,研究论文发展脉络
Chat Paper
正在生成论文摘要