Sets of complementary LLRs to improve OSD post-processing of BP decoding
2023 12th International Symposium on Topics in Coding (ISTC)(2023)
摘要
This article deals with Ordered Statistics Decoding (OSD) applied to the soft outputs of the Belief Propagation (BP) algorithm. We first model the weighted sum of the a posteriori LLRs across BP decoding iterations into a neuron. The neuron is then trained with the focal loss to compute for each BP decoding failure a set of accumulated Log Likelihood Ratios (LLRs) suited for OSD post-processing. Then, we propose a recursive selection procedure of LLRs sets, for multiple OSD post-processing. This selection is carried out from the sets of a posteriori LLRs calculated at each BP iteration, and from the accumulated LLRs optimized for the OSD, based on their joint probabilities of failure with OSD post-processing. An OSD is then applied to each set of LLRs belonging to the selection. In addition, we propose to reduce the OSD post-processing decoding complexity without significantly degrading its performance. Our results show that this new decoding method provides an effective way to bridge the gap to maximum likelihood decoding for short and long Low Density Parity Check (LDPC) codes.
更多查看译文
关键词
LDPC,belief propagation,ordered statistics decoding post-processing
AI 理解论文
溯源树
样例
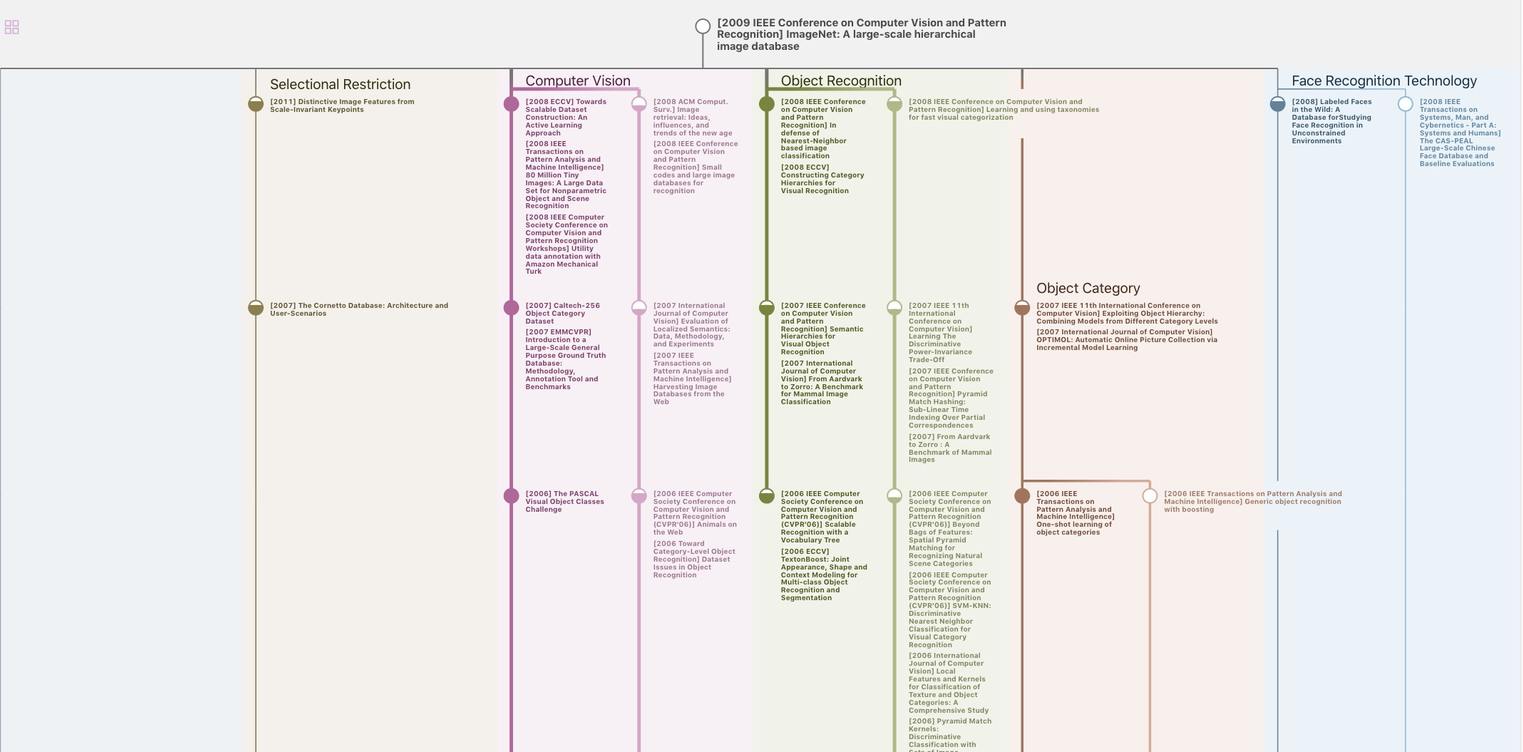
生成溯源树,研究论文发展脉络
Chat Paper
正在生成论文摘要