Real-Time Identification of Pancreatic Cancer Cases Using Artificial Intelligence Developed on Danish Nationwide Registry Data
JCO CLINICAL CANCER INFORMATICS(2023)
摘要
PURPOSE Pancreatic cancer is expected to be the second leading cause of cancer-related deaths worldwide within few years. Most patients are not diagnosed in time for curative-intent treatment. Accelerating the time of diagnosis is a key component of reducing pancreatic cancer mortality. We developed and tested a dynamic algorithm aiming at proactively identifying patients with a substantially elevated risk of having undiagnosed pancreatic cancer. METHODS Machine learning methodology was applied to a live stream of nationwide Danish registry data. A hybrid case-control and prospective cohort design relying on incidence density sampling was used. Three models with minimal tuning were tested. All performance evaluation metrics were based on out-of-sample, out-of-time data in a monthly walk-forward strategy to avoid any temporal biases or inflation of performance metrics. Outcome was a diagnosis of pancreatic cancer. RESULTS Subgroups identified had a 10.1% risk of being diagnosed with pancreatic cancer within 1 year, corresponding to a number needed to screen of 9.9. When considering competing, potentially computed tomography-detectable GI cancers, this number is reduced to 5.7. The time of diagnosis can be accelerated by up to 142 days. CONCLUSION Currently available nationwide live data and computational resources are sufficient for real-time identification of individuals with at least 10.1% risk of having undiagnosed pancreatic cancer and 17.7% risk of any GI cancer in the Danish population. For prospective identification of high-risk patients, the area under the curve is not a useful indication of the positive predictive values achieved. Viable design solutions are demonstrated, which address the main shortfalls of the existing cancer prediction efforts in relation to temporal biases, leaks, and performance metric inflation. Efficacy evaluations with resection rates and mortality as end points are needed.
更多查看译文
AI 理解论文
溯源树
样例
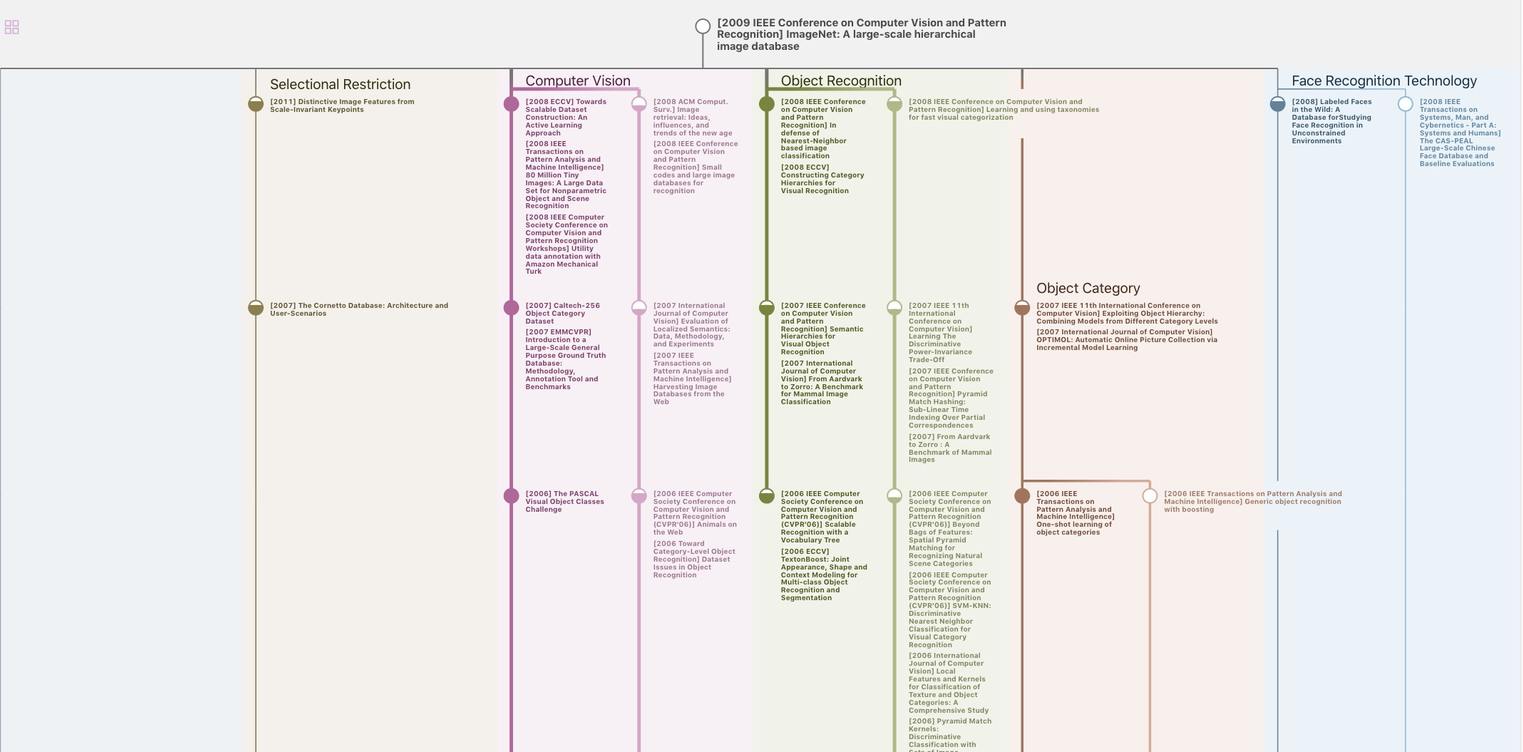
生成溯源树,研究论文发展脉络
Chat Paper
正在生成论文摘要