Early Prediction of Impending Exertional Heat Stroke With Wearable Multimodal Sensing and Anomaly Detection
IEEE JOURNAL OF BIOMEDICAL AND HEALTH INFORMATICS(2023)
摘要
We employed wearable multimodal sensing (heart rate and triaxial accelerometry) with machine learning to enable early prediction of impending exertional heat stroke (EHS). US Army Rangers and Combat Engineers (N = 2,102) were instrumented while participating in rigorous 7-mile and 12-mile loaded rucksack timed marches. There were three EHS cases, and data from 478 Rangers were analyzed for model building and controls. The data-driven machine learning approach incorporated estimates of physiological strain (heart rate) and physical stress (estimated metabolic rate) trajectories, followed by reconstruction to obtain compressed representations which then fed into anomaly detection for EHS prediction. Impending EHS was predicted from 33 to 69 min before collapse. These findings demonstrate that low dimensional physiological stress to strain patterns with machine learning anomaly detection enables early prediction of impending EHS which will allow interventions that minimize or avoid pathophysiological sequelae. We describe how our approach can be expanded to other physical activities and enhanced with novel sensors.
更多查看译文
关键词
Heating systems,Biomedical monitoring,Temperature measurement,Trajectory,Heart rate,Estimation,Stress,Anomaly detection,exercise physiology,exertional heat stroke (EHS),heat stress,machine learning,military medicine,wearable sensors
AI 理解论文
溯源树
样例
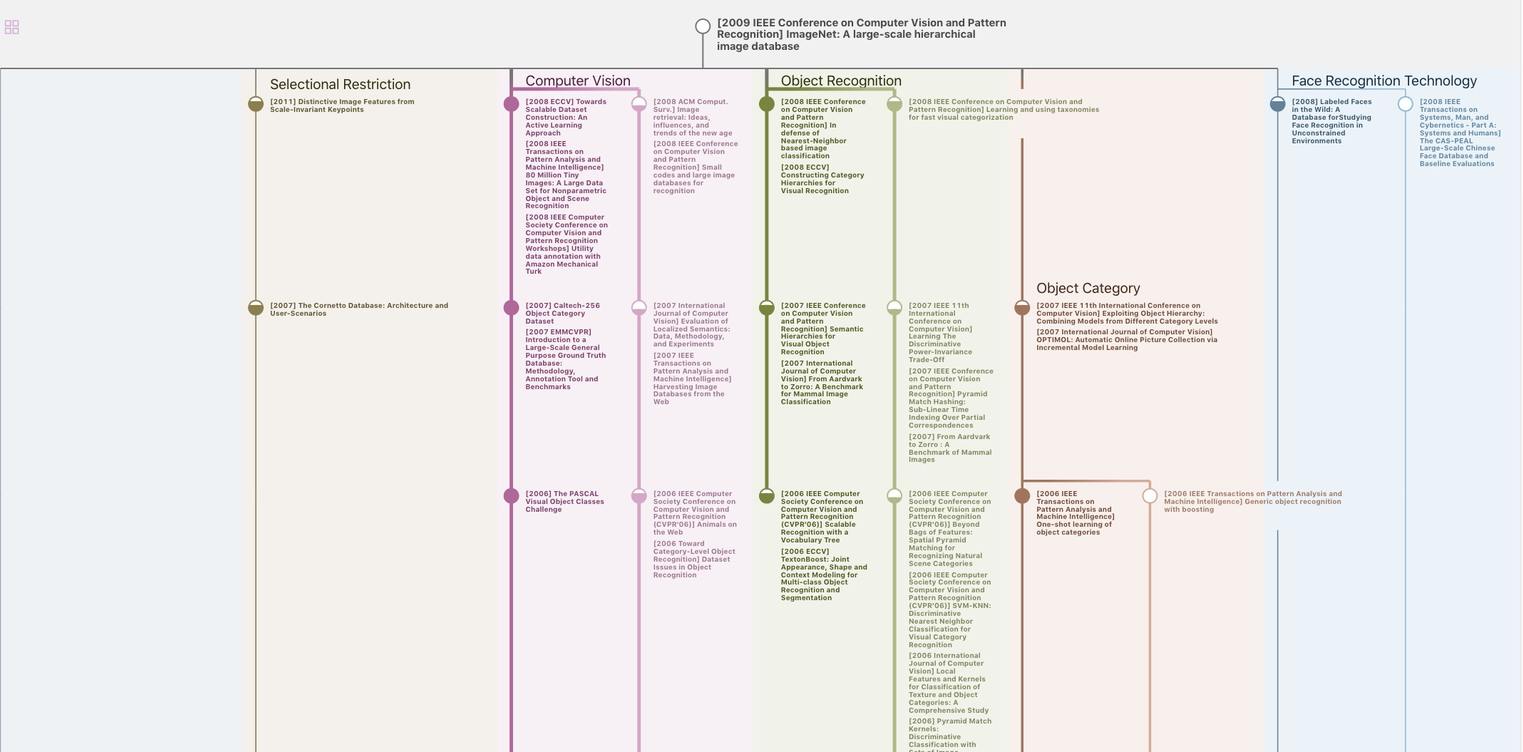
生成溯源树,研究论文发展脉络
Chat Paper
正在生成论文摘要