Treatment choice, mean square regret and partial identification
arXiv (Cornell University)(2023)
摘要
We consider a decision maker who faces a binary treatment choice when their welfare is only partially identified from data. We contribute to the literature by anchoring our finite-sample analysis on mean square regret, a decision criterion advocated by Kitagawa et al. in (2022) "Treatment Choice with Nonlinear Regret" . We find that optimal rules are always fractional, irrespective of the width of the identified set and precision of its estimate. The optimal treatment fraction is a simple logistic transformation of the commonly used t-statistic multiplied by a factor calculated by a simple constrained optimization. This treatment fraction gets closer to 0.5 as the width of the identified set becomes wider, implying the decision maker becomes more cautious against the adversarial Nature.
更多查看译文
关键词
mean square regret,treatment,choice
AI 理解论文
溯源树
样例
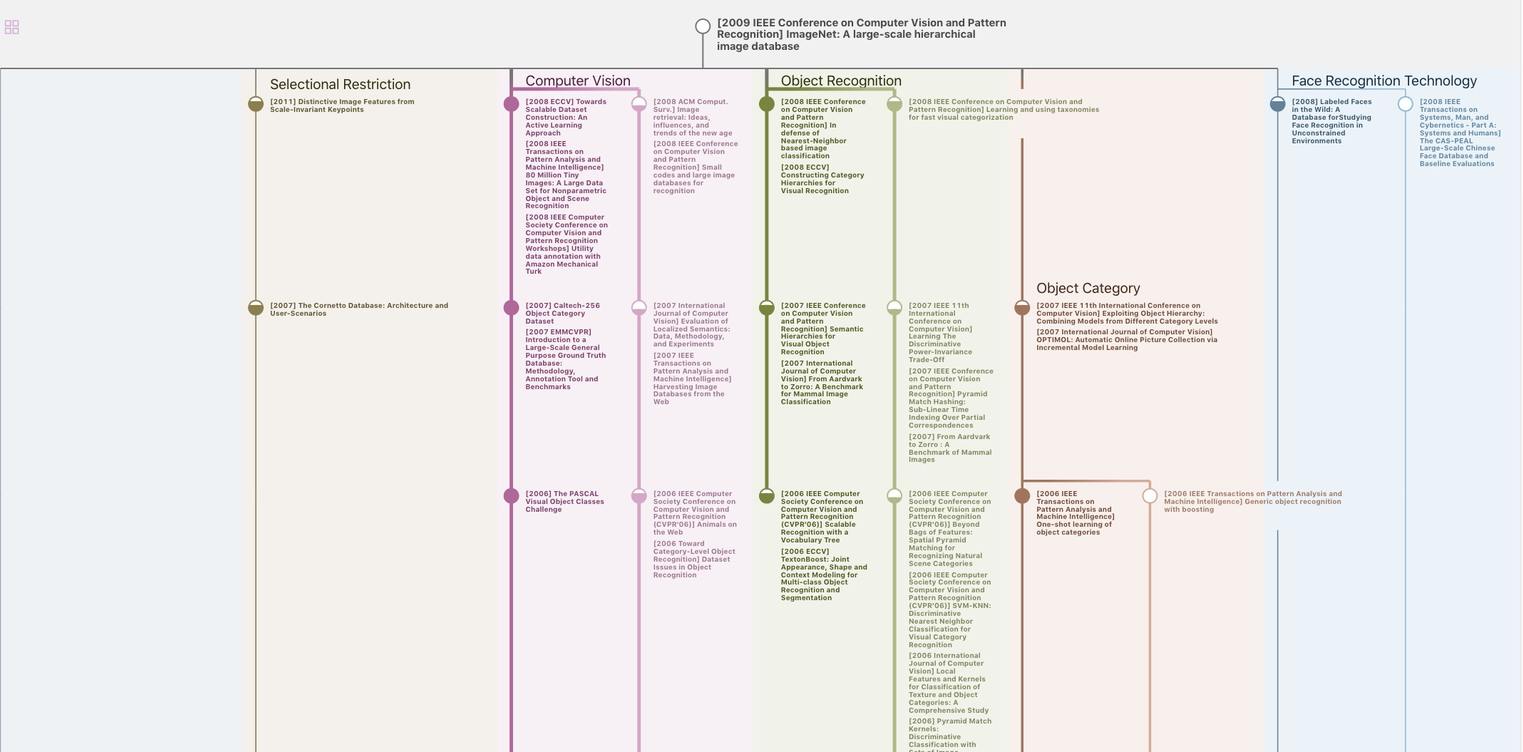
生成溯源树,研究论文发展脉络
Chat Paper
正在生成论文摘要