Let Models Speak Ciphers: Multiagent Debate through Embeddings
ICLR 2024(2023)
摘要
Discussion and debate among Large Language Models (LLMs) have gained considerable attention due to their potential to enhance the reasoning ability of LLMs. Although natural language is an obvious choice for communication due to LLM's language understanding capability, the token sampling step needed when generating natural language poses a potential risk of information loss, as it uses only one token to represent the model's belief across the entire vocabulary. In this paper, we introduce a communication regime named CIPHER (Communicative Inter-Model Protocol Through Embedding Representation) to address this issue. Specifically, we remove the token sampling step from LLMs and let them communicate their beliefs across the vocabulary through the expectation of the raw transformer output embeddings. Remarkably, by deviating from natural language, CIPHER offers an advantage of encoding a broader spectrum of information without any modification to the model weights. While the state-of-the-art LLM debate methods using natural language outperforms traditional inference by a margin of 1.5-8%, our experiment results show that CIPHER debate further extends this lead by 1-3.5% across five reasoning tasks and multiple open-source LLMs of varying sizes. This showcases the superiority and robustness of embeddings as an alternative "language" for communication among LLMs.
更多查看译文
关键词
multiagent debate,large language models,inter-model communication,embedding representation
AI 理解论文
溯源树
样例
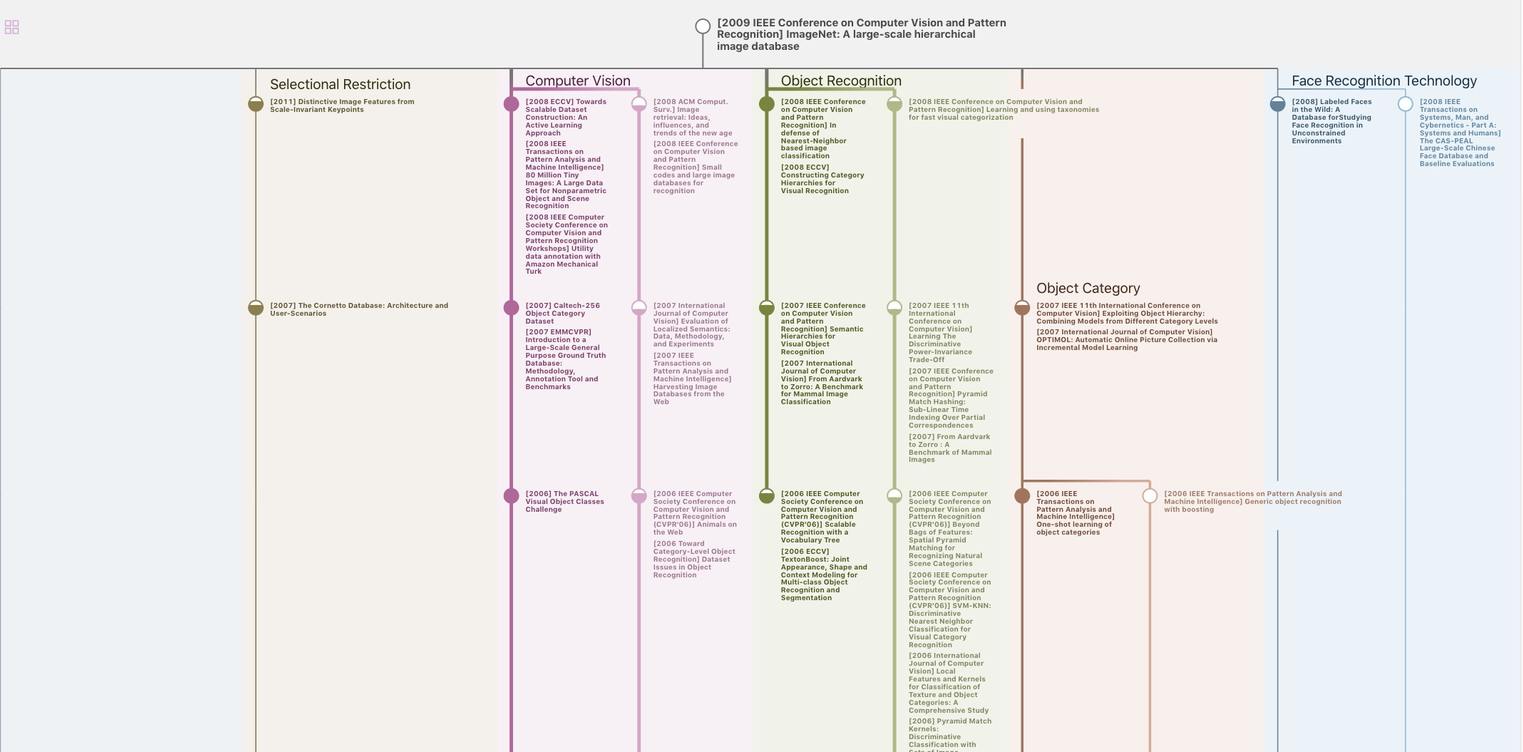
生成溯源树,研究论文发展脉络
Chat Paper
正在生成论文摘要