Low-Rank Tensor Completion via Novel Sparsity-Inducing Regularizers
CoRR(2023)
摘要
To alleviate the bias generated by the l1-norm in the low-rank tensor completion problem, nonconvex surrogates/regularizers have been suggested to replace the tensor nuclear norm, although both can achieve sparsity. However, the thresholding functions of these nonconvex regularizers may not have closed-form expressions and thus iterations are needed, which increases the computational loads. To solve this issue, we devise a framework to generate sparsity-inducing regularizers with closed-form thresholding functions. These regularizers are applied to low-tubal-rank tensor completion, and efficient algorithms based on the alternating direction method of multipliers are developed. Furthermore, convergence of our methods is analyzed and it is proved that the generated sequences are bounded and any limit point is a stationary point. Experimental results using synthetic and real-world datasets show that the proposed algorithms outperform the state-of-the-art methods in terms of restoration performance.
更多查看译文
关键词
tensor,low-rank,sparsity-inducing
AI 理解论文
溯源树
样例
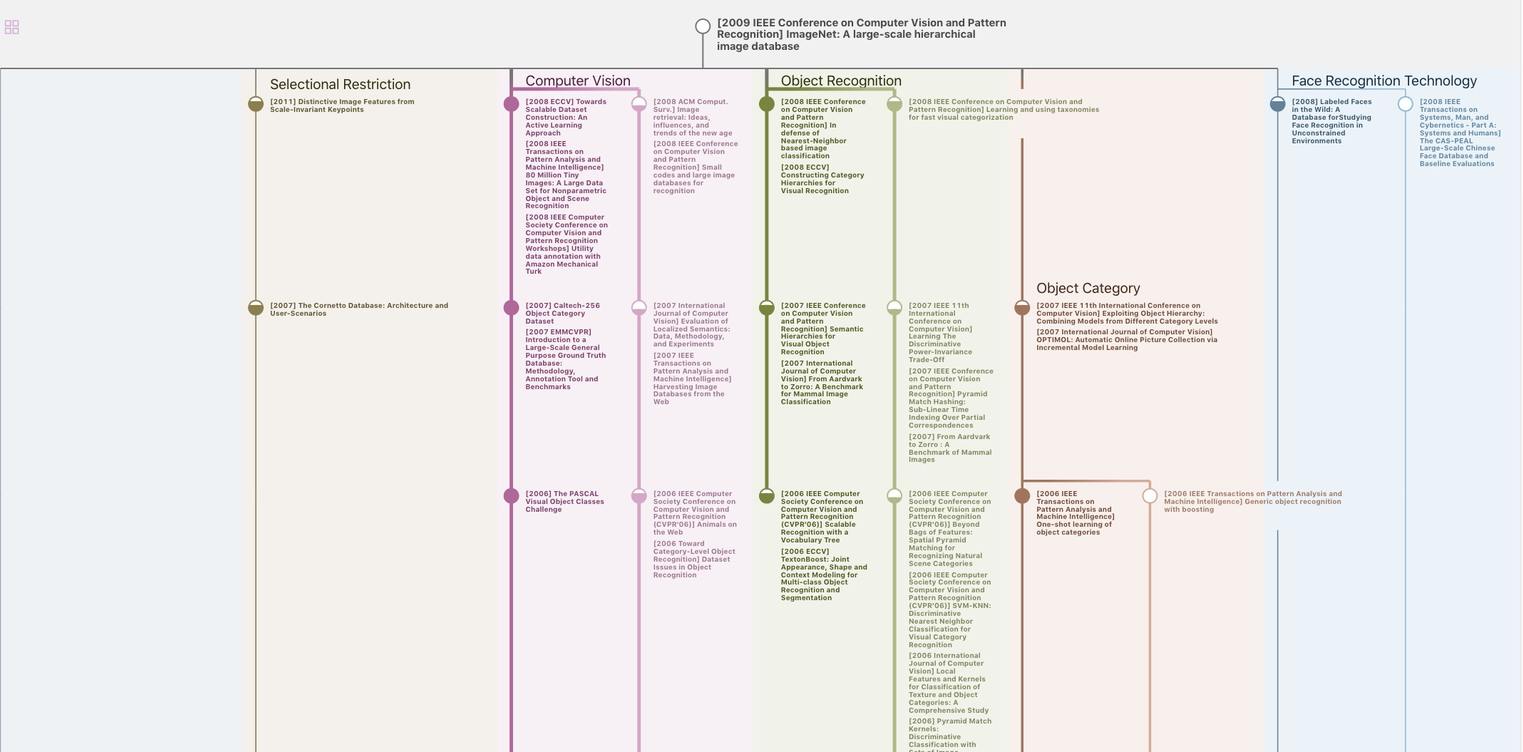
生成溯源树,研究论文发展脉络
Chat Paper
正在生成论文摘要