Factual and Personalized Recommendations using Language Models and Reinforcement Learning
CoRR(2023)
摘要
Recommender systems (RSs) play a central role in connecting users to content, products, and services, matching candidate items to users based on their preferences. While traditional RSs rely on implicit user feedback signals, conversational RSs interact with users in natural language. In this work, we develop a comPelling, Precise, Personalized, Preference-relevant language model (P4LM) that recommends items to users while putting emphasis on explaining item characteristics and their relevance. P4LM uses the embedding space representation of a user's preferences to generate compelling responses that are factually-grounded and relevant w.r.t. the user's preferences. Moreover, we develop a joint reward function that measures precision, appeal, and personalization, which we use as AI-based feedback in a reinforcement learning-based language model framework. Using the MovieLens 25M dataset, we demonstrate that P4LM delivers compelling, personalized movie narratives to users.
更多查看译文
关键词
personalized recommendations,language models,learning
AI 理解论文
溯源树
样例
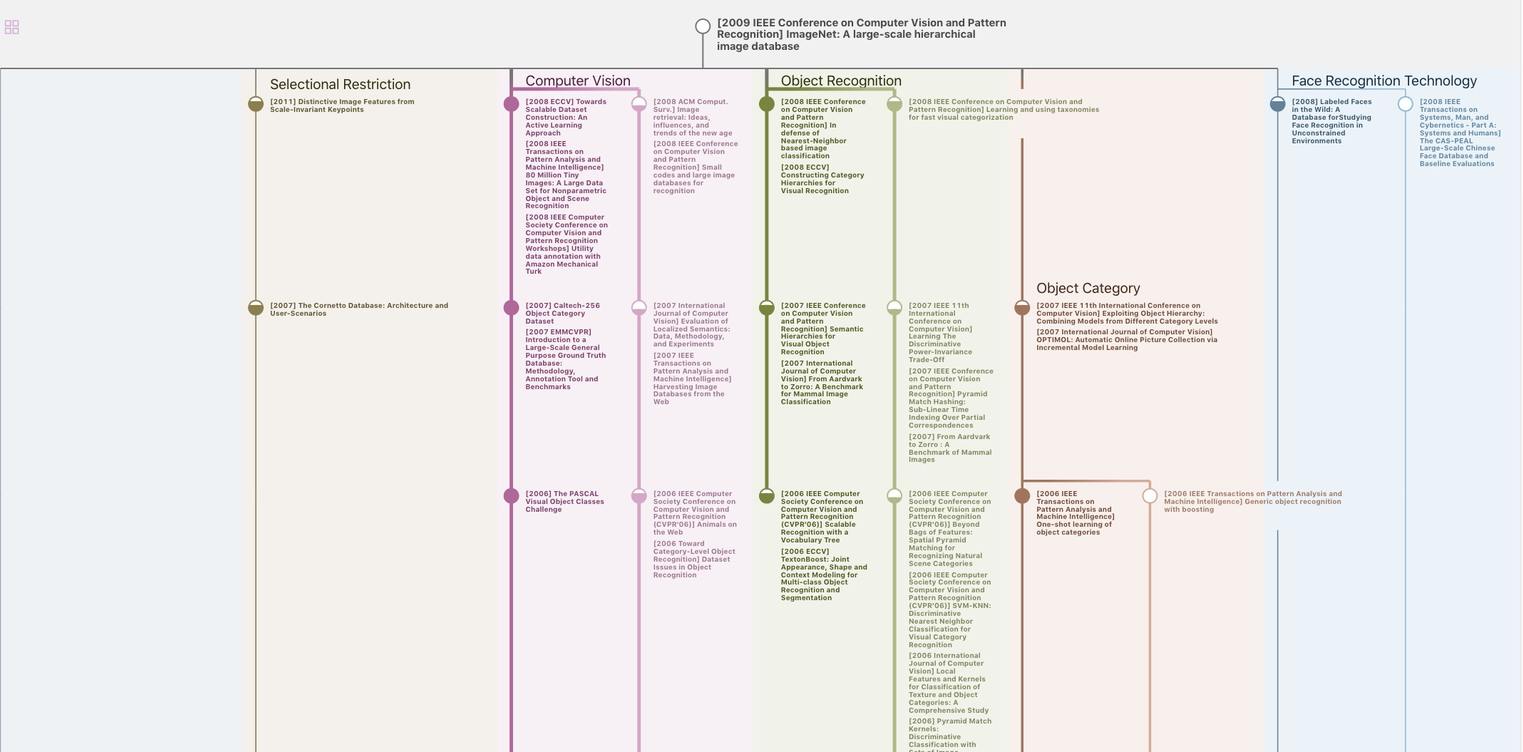
生成溯源树,研究论文发展脉络
Chat Paper
正在生成论文摘要