Learning bounded-degree polytrees with known skeleton
CoRR(2023)
摘要
We establish finite-sample guarantees for efficient proper learning of bounded-degree polytrees, a rich class of high-dimensional probability distributions and a subclass of Bayesian networks, a widely-studied type of graphical model. Recently, Bhattacharyya et al. (2021) obtained finite-sample guarantees for recovering tree-structured Bayesian networks, i.e., 1-polytrees. We extend their results by providing an efficient algorithm which learns $d$-polytrees in polynomial time and sample complexity for any bounded $d$ when the underlying undirected graph (skeleton) is known. We complement our algorithm with an information-theoretic sample complexity lower bound, showing that the dependence on the dimension and target accuracy parameters are nearly tight.
更多查看译文
关键词
known skeleton,learning,bounded-degree
AI 理解论文
溯源树
样例
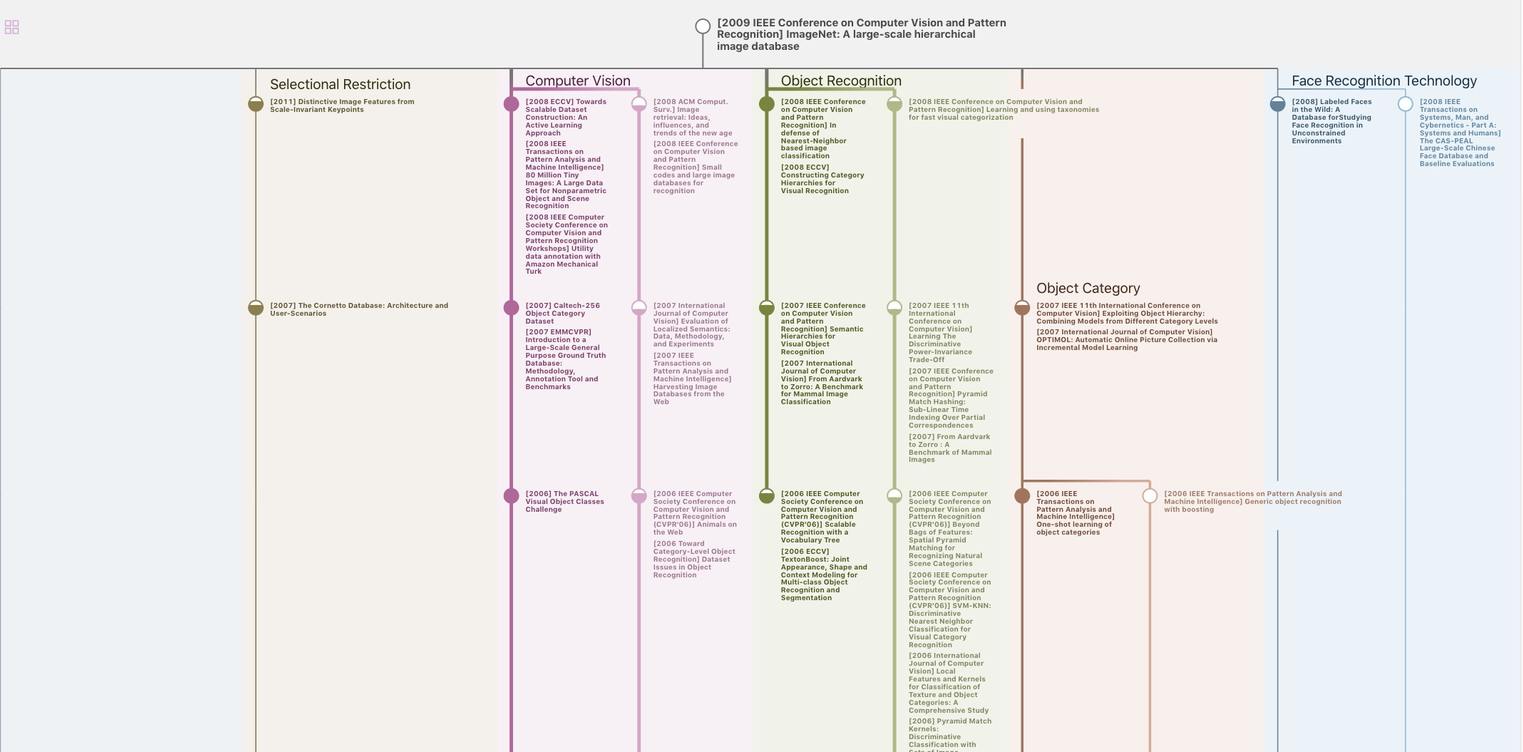
生成溯源树,研究论文发展脉络
Chat Paper
正在生成论文摘要