A Systematic Study on Reproducibility of Reinforcement Learning in Recommendation Systems.
ACM Transactions on Recommender Systems(2023)
摘要
Reproducibility is a main principle in science and fundamental to ensure scientific progress. However, many recent works point out that there are widespread deficiencies for this aspect in the AI field, making the reproducibility of results impractical or even impossible. We therefore studied the state of reproducibility support on the topic of Reinforcement Learning & Recommender Systems to analyse the situation in this context. We collected a total of 60 papers and analysed them by defining a set of variables to inspect the most important aspects that enable reproducibility, such as dataset, pre-processing code, hardware specifications, software dependencies, algorithm implementation, algorithm hyperparameters, and experiment code. Furthermore, we used the ACM Badges definitions assigning them to the selected papers. We discovered that, like in many other AI domains, the Reinforcement Learning & Recommender Systems field is grappling with a reproducibility crisis, as none of the selected papers were reproducible when strictly applying the ACM Badges definitions according to our analysis.
更多查看译文
关键词
reproducibility,reinforcement learning
AI 理解论文
溯源树
样例
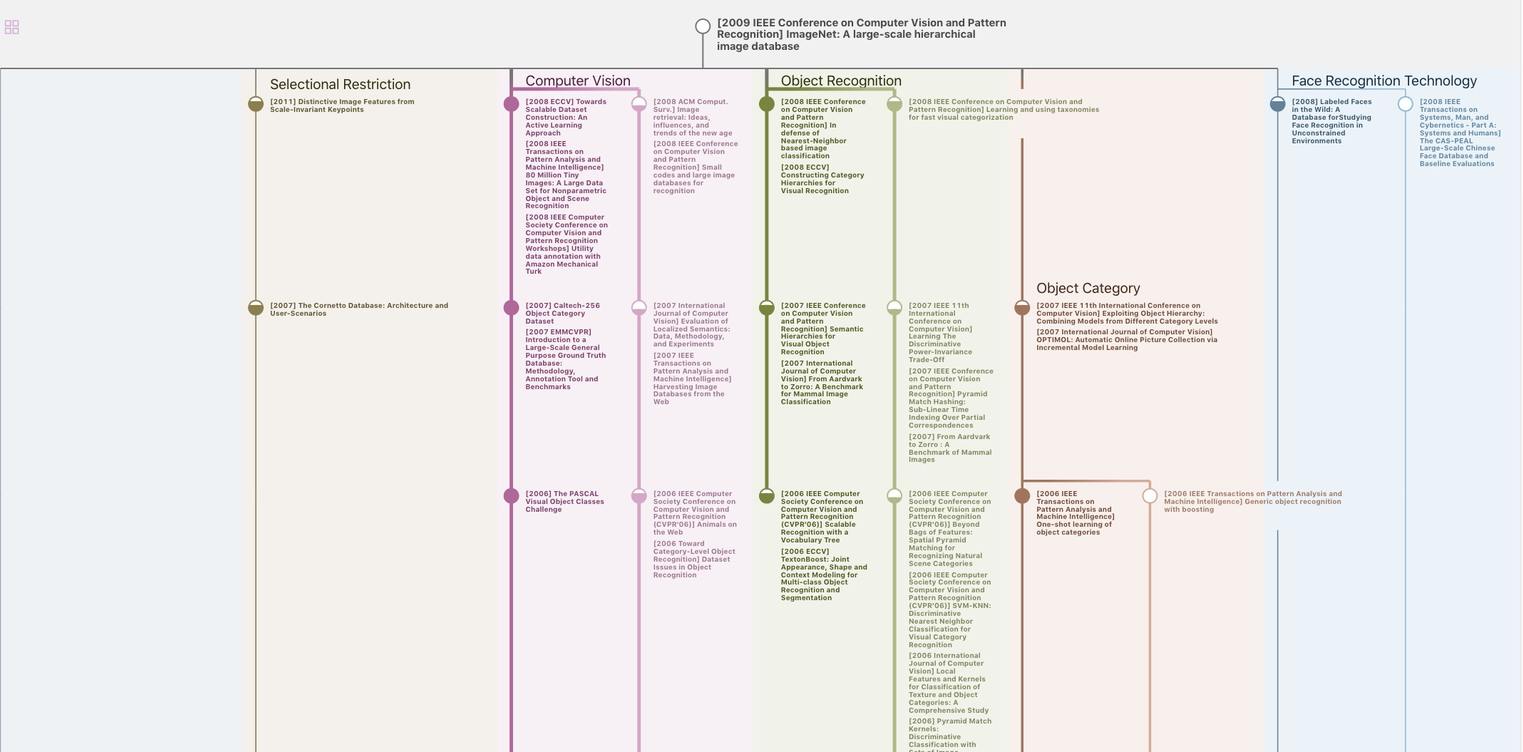
生成溯源树,研究论文发展脉络
Chat Paper
正在生成论文摘要