Cold-Start Model Adaptation: Evaluation of Short Baseline Calibration
UbiComp/ISWC '23 Adjunct: Adjunct Proceedings of the 2023 ACM International Joint Conference on Pervasive and Ubiquitous Computing & the 2023 ACM International Symposium on Wearable Computing(2023)
摘要
Human physiology and reactions to external stimuli differ between individuals. Researchers have developed strategies to adapt to these differences but the adaptations generally require data from each individual beforehand. A cold-start occurs when there is no data from a new individual. To address this, current study proposes user calibration which uses short segments of easily obtainable baseline data to adapt to new individuals. Experiments were conducted on two public stress and affect detection datasets, WESAD and SWELL-KW, to assess the effectiveness of the proposed calibration method and to determine suitable duration of the baseline measurement. Results showed that user calibration always beat the non-personalized model and segments of 3-8 minutes seemed to be most promising to consider for future use.
更多查看译文
AI 理解论文
溯源树
样例
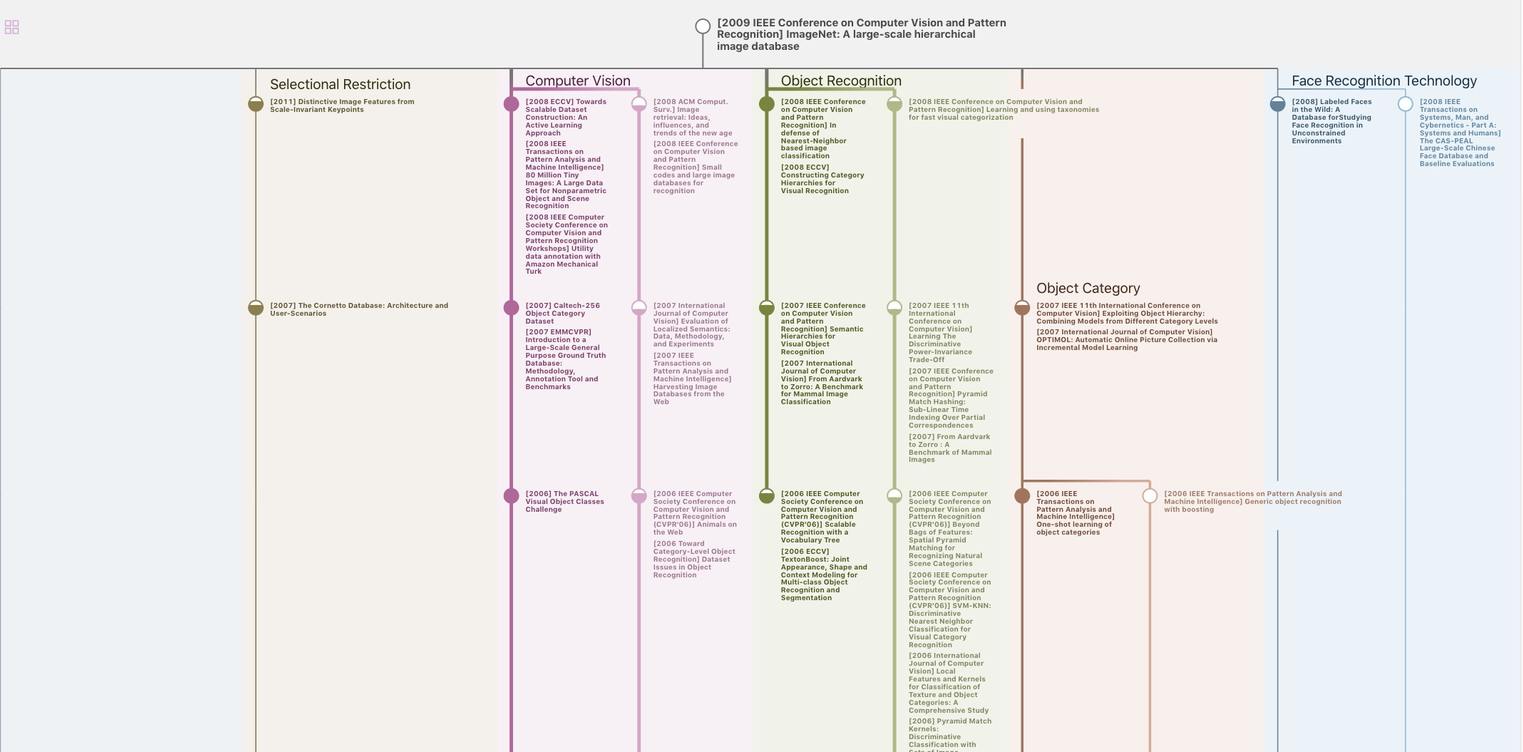
生成溯源树,研究论文发展脉络
Chat Paper
正在生成论文摘要