GCFormer: A Graph Convolutional Transformer for Speech Emotion Recognition
PROCEEDINGS OF THE 25TH INTERNATIONAL CONFERENCE ON MULTIMODAL INTERACTION, ICMI 2023(2023)
摘要
Graph convolutional networks (GCNs) have achieved excellent results in image classification and natural language processing. However, at present, the application of GCNs in speech emotion recognition (SER) is not widely studied. Meanwhile, recent studies have shown that GCNs may not be able to adaptively capture the long-range context emotional information over the whole audio. To alleviate this problem, this paper proposes a Graph Convolutional Transformer (GCFormer) model which empowers the model to extract local and global emotional information. Specifically, we construct a cyclic graph and perform concise graph convolution operations to obtain spatial local features. Then, a consecutive transformer network further strives to learn more high-level representations and their global temporal correlation. Finally and sequentially, the learned serialized representations from the transformer are mapped into a vector through a gated recurrent unit (GRU) pooling layer for emotion classification. The experiment results obtained on two public emotional datasets demonstrate that the proposed GCFormer performs significantly better than other GCN-based models in terms of prediction accuracy, and surpasses the other state-of-the-art deep learning models in terms of prediction accuracy and model efficiency.
更多查看译文
关键词
Graph Convolutional Network,Transformer,Gated Recurrent Unit,Speech Emotion Recognition
AI 理解论文
溯源树
样例
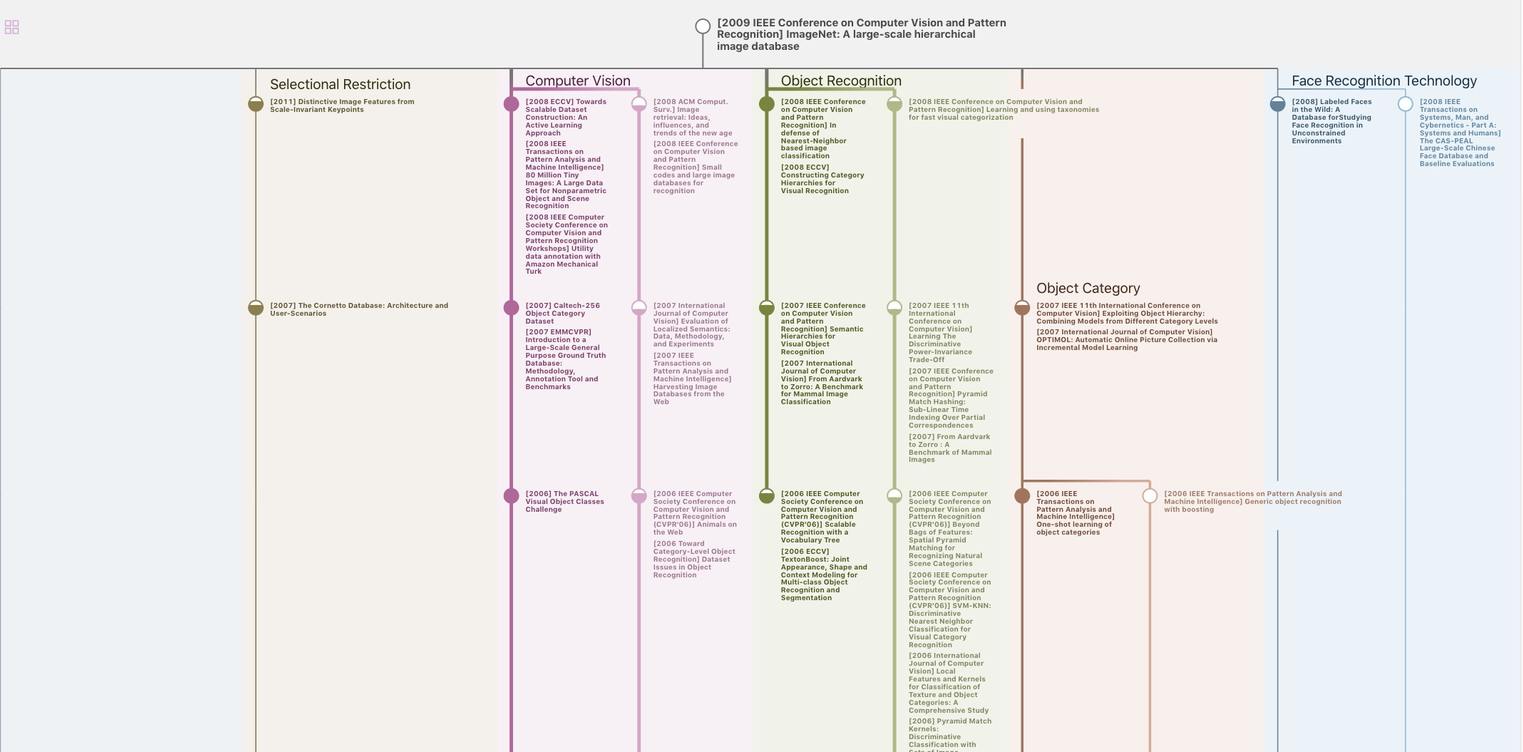
生成溯源树,研究论文发展脉络
Chat Paper
正在生成论文摘要