Digital Phenotyping of Autoimmune Diseases Using Non-Contact Radio Frequency Sensing: A Longitudinal Study Comparing Systemic Lupus Erythematosus and Healthy Participants.
UbiComp/ISWC '23 Adjunct: Adjunct Proceedings of the 2023 ACM International Joint Conference on Pervasive and Ubiquitous Computing & the 2023 ACM International Symposium on Wearable Computing(2023)
摘要
Recent ubiquitous sensing technologies make it possible to capture streaming digital data that reports aspects of a patient’s physiology, behavior, and symptoms both quantitatively and in real time. As a result, it may be possible to develop streaming disease readouts that are more accurate and less obtrusive than relying on patient and caregiver reports alone. This study investigates the feasibility of leveraging physiological and behavioral signals extracted from a radio frequency sensing device to characterize metrics indicative of breathing, mobility, and sleep patterns. We investigate the variations in these signals between individuals with Systemic Lupus Erythematosus (SLE) and healthy participants in a 6-months longitudinal, exploratory, in-home study involving 19 SLE and 28 healthy participants. Results show that many signals (e.g., breathing rate, sleep efficiency, and gait speed) significantly distinguish SLE and healthy participants and demonstrate the potential of using remote sensing as an unobtrusive low-burden tool to assess disease symptoms continuously and in real time.
更多查看译文
AI 理解论文
溯源树
样例
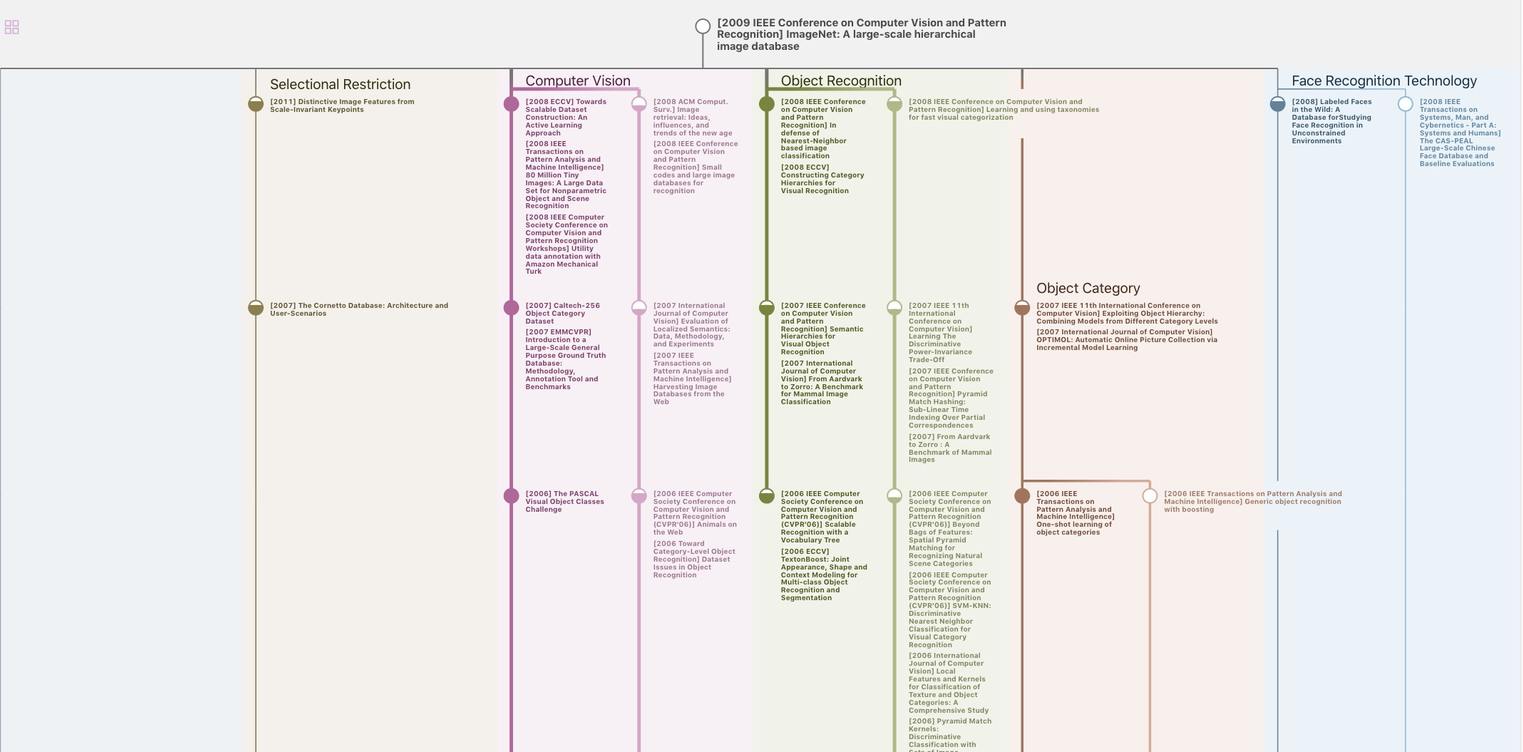
生成溯源树,研究论文发展脉络
Chat Paper
正在生成论文摘要