Learning from Hierarchical Structure of Knowledge Graph for Recommendation
ACM TRANSACTIONS ON INFORMATION SYSTEMS(2024)
摘要
Knowledge graphs (KGs) can help enhance recommendations, especially for the data-sparsity scenarios with limited user-item interaction data. Due to the strong power of representation learning of graph neural networks (GNNs), recentworks of KG-based recommendation deploy GNN models to learn from both knowledge graph and user-item bipartite interaction graph. However, theseworks have not well considered the hierarchical structure of knowledge graph, leading to sub-optimal results. Despite the benefit of hierarchical structure, leveraging it is challenging since the structure is always partly-observed. In this work, we first propose to reveal unknown hierarchical structures with a supervised signal detection method and then exploit the hierarchical structure with disentangling representation learning. We conduct experiments on two large-scale datasets, of which the results well verify the superiority and rationality of the proposed method. Further experiments of ablation study with respect to key model designs have demonstrated the effectiveness and rationality of our proposed model. The code is available at https://github.com/tsinghua-fib-lab/HIKE.
更多查看译文
关键词
Personalized recommendation,knowledge graph,disentangled representation learning,graph neural network
AI 理解论文
溯源树
样例
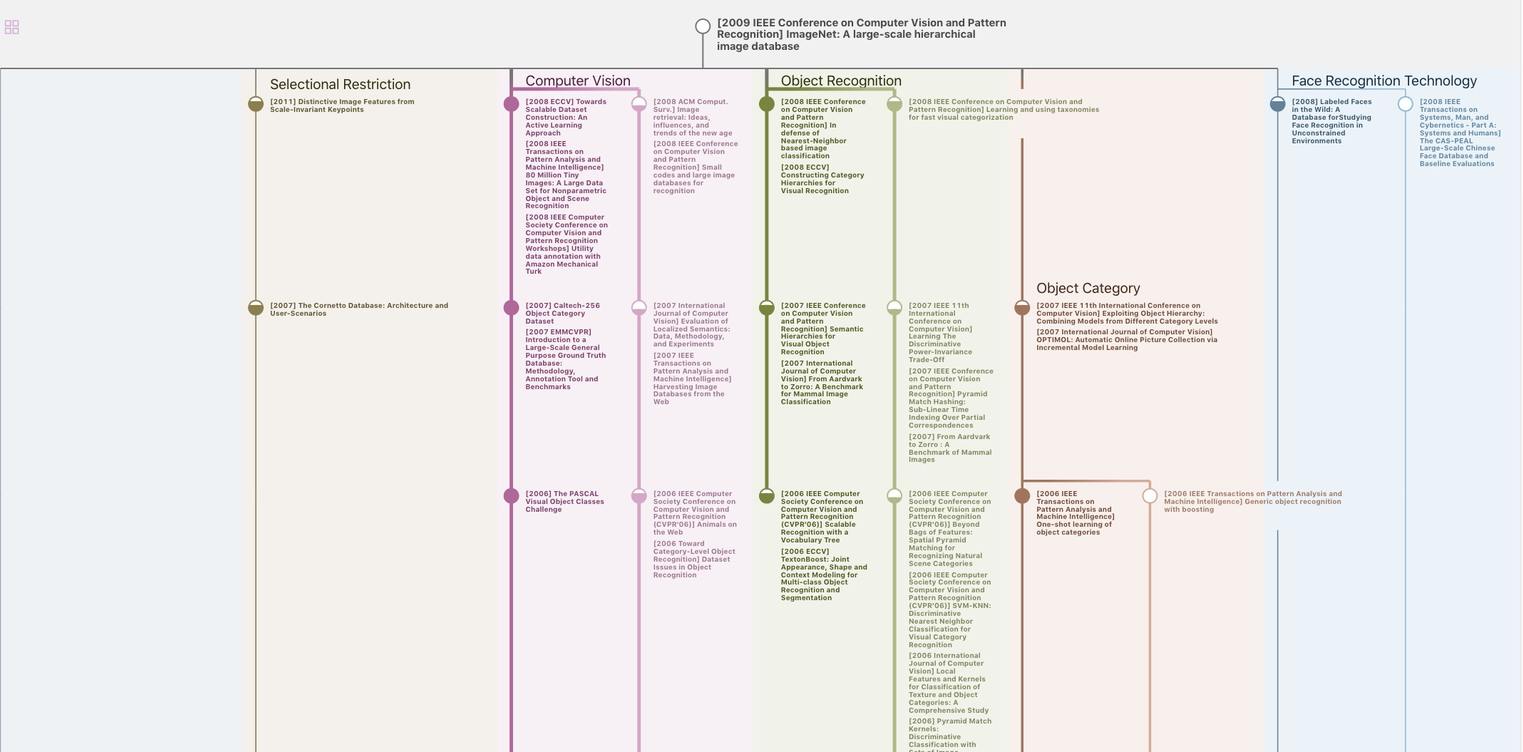
生成溯源树,研究论文发展脉络
Chat Paper
正在生成论文摘要