Instance-Based Continual Learning: A Real-World Dataset and Baseline for Fresh Recognition
ACM TRANSACTIONS ON MULTIMEDIA COMPUTING COMMUNICATIONS AND APPLICATIONS(2024)
摘要
Real-time learning on real-world data streams with temporal relations is essential for intelligent agents. However, current online Continual Learning (CL) benchmarks adopt the mini-batch setting and are composed of temporally unrelated and disjoint tasks aswell as pre-set class boundaries. In this paper, we delve into a real-world CL scenario for fresh recognition where algorithms are required to recognize a huge variety of products to facilitate the checkout speed. Products mainly consists of packaged cereals, seasonal fruits, and vegetables from local farms or shipped from overseas. Since algorithms process instance streams consisting of sequential images, we name this real-world CL problem as Instance-Based Continual Learning (IBCL). Different from the current online CL setting, algorithms are required to perform instant testing and learning upon each incoming instance. Moreover, IBCL has no task boundaries or class boundaries and allows the evolution and the forgetting of old samples within each class. To promote the researches on real CL challenges, we propose the first real-world CL dataset coined the Continual Fresh Recognition (CFR) dataset, which consists of fresh recognition data streams (766K labelled images in total) collected from 30 supermarkets. Based on the CFR dataset, we extensively evaluate the performance of current online CL methods under various settings and find that current prominent online CL methods operate at high latency and demand significant memory consumption to cache old samples for replaying. Therefore, we make the first attempt to design an efficient and effective Instant Training-Free Learning (ITFL) framework for IBCL. ITFL consists of feature extractors trained in the metric learning manner and reformulates CL as a temporal classification problem among several most similar classes. Unlike current online CL methods that cache image samples (150KB per image) and rely on training to learn new knowledge, our framework only caches features (2KB per image) and is free of training in deployment. Extensive evaluations across three datasets demonstrate that our method achieves comparable recognition accuracy to current methods with lower latency and less resource consumption. Our codes and datasets will be publicly available at https://github.com/detectRecog/IBCL.
更多查看译文
关键词
Online continual learning,fresh recognition
AI 理解论文
溯源树
样例
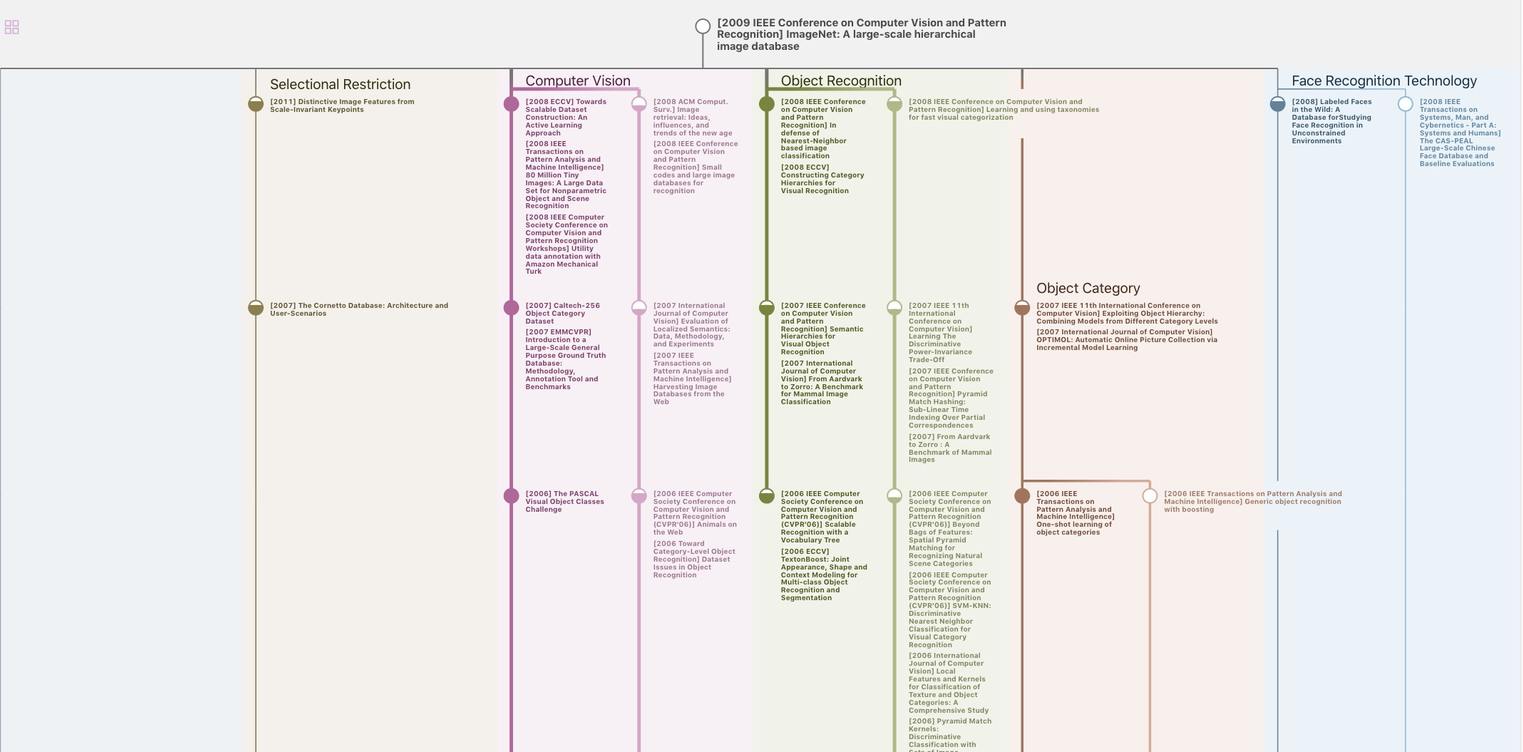
生成溯源树,研究论文发展脉络
Chat Paper
正在生成论文摘要