Attentional Composition Networks for Long-Tailed Human Action Recognition
ACM TRANSACTIONS ON MULTIMEDIA COMPUTING COMMUNICATIONS AND APPLICATIONS(2024)
摘要
The problem of long-tailed visual recognition has been receiving increasing research attention. However, the long-tailed distribution problem remains underexplored for video-based visual recognition. To address this issue, in this article we propose a compositional learning based solution for video-based human action recognition. Our method, named Attentional Composition Networks (ACN), first learns verb-like and prepositionlike components, then shuffles these components to generate samples for the tail classes in the feature space to augment the data for the tail classes. Specifically, during training, we represent each action video by a graph that captures the spatial-temporal relations (edges) among detected human/object instances (nodes). Then, ACN utilizes the position information to decompose each action into a set of verb and preposition representations using the edge features in the graph. After that, the verb and preposition features from different videos are combined via an attention structure to synthesize feature representations for tail classes. This way, we can enrich the data for the tail classes and consequently improve the action recognition for these classes. To evaluate the compositional human action recognition, we further contribute a new human action recognition dataset, namely NEU-Interaction (NEU-I). Experimental results on both Something-Something V2 and the proposed NEU-I demonstrate the effectiveness of the proposed method for long-tailed, few-shot, and zero-shot problems in human action recognition. Source code and the NEU-I dataset are available at https://github.com/YajieW99/ACN.
更多查看译文
关键词
Compositional learning,long tail,few-shot,zero-shot,action recognition
AI 理解论文
溯源树
样例
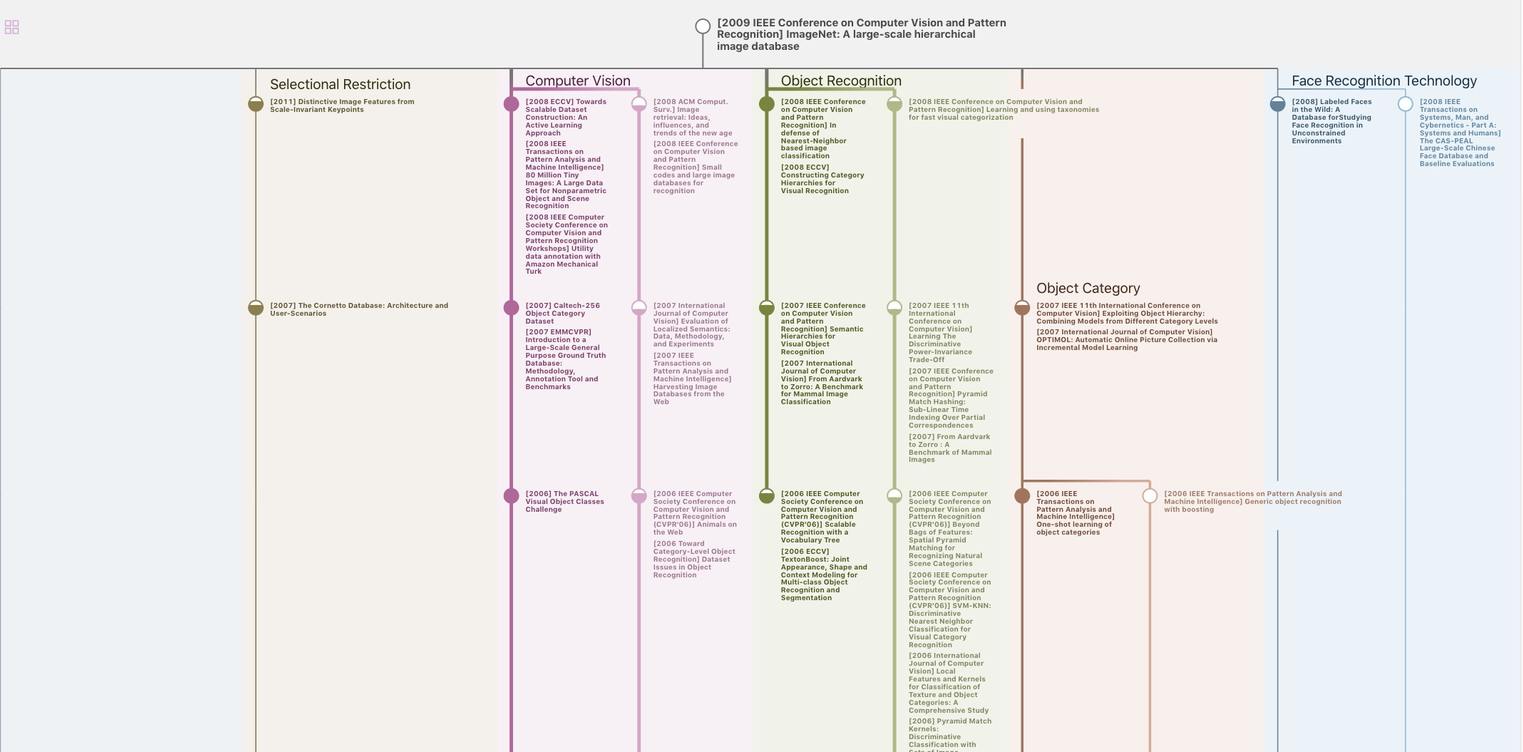
生成溯源树,研究论文发展脉络
Chat Paper
正在生成论文摘要