Diverse Image Captioning via Conditional Variational Autoencoder and Dual Contrastive Learning
ACM TRANSACTIONS ON MULTIMEDIA COMPUTING COMMUNICATIONS AND APPLICATIONS(2024)
摘要
Diverse image captioning has achieved substantial progress in recent years. However, the discriminability of generative models and the limitation of cross entropy loss are generally overlooked in the traditional diverse image captioning models, which seriously hurts both the diversity and accuracy of image captioning. In this article, aiming to improve diversity and accuracy simultaneously, we propose a novel Conditional Variational Autoencoder (DCL-CVAE) framework for diverse image captioning by seamlessly integrating sequential variational autoencoder with contrastive learning. In the encoding stage, we first build conditional variational autoencoders to separately learn the sequential latent spaces for a pair of captions. Then, we introduce contrastive learning in the sequential latent spaces to enhance the discriminability of latent representations for both image-caption pairs andmismatched pairs. In the decoding stage, we leverage the captions sampled from the pre-trained Long Short-Term Memory (LSTM), LSTM decoder as the negative examples and perform contrastive learning with the greedily sampled positive examples, which can restrain the generation of common words and phrases induced by the cross entropy loss. By virtue of dual constrastive learning, DCL-CVAE is capable of encouraging the discriminability and facilitating the diversity, while promoting the accuracy of the generated captions. Extensive experiments are conducted on the challenging MSCOCO dataset, showing that our proposed methods can achieve a better balance between accuracy and diversity compared to the state-of-the-art diverse image captioning models.
更多查看译文
关键词
Diverse image captioning,variational autoencoder,contrastive learning,sequential latent space
AI 理解论文
溯源树
样例
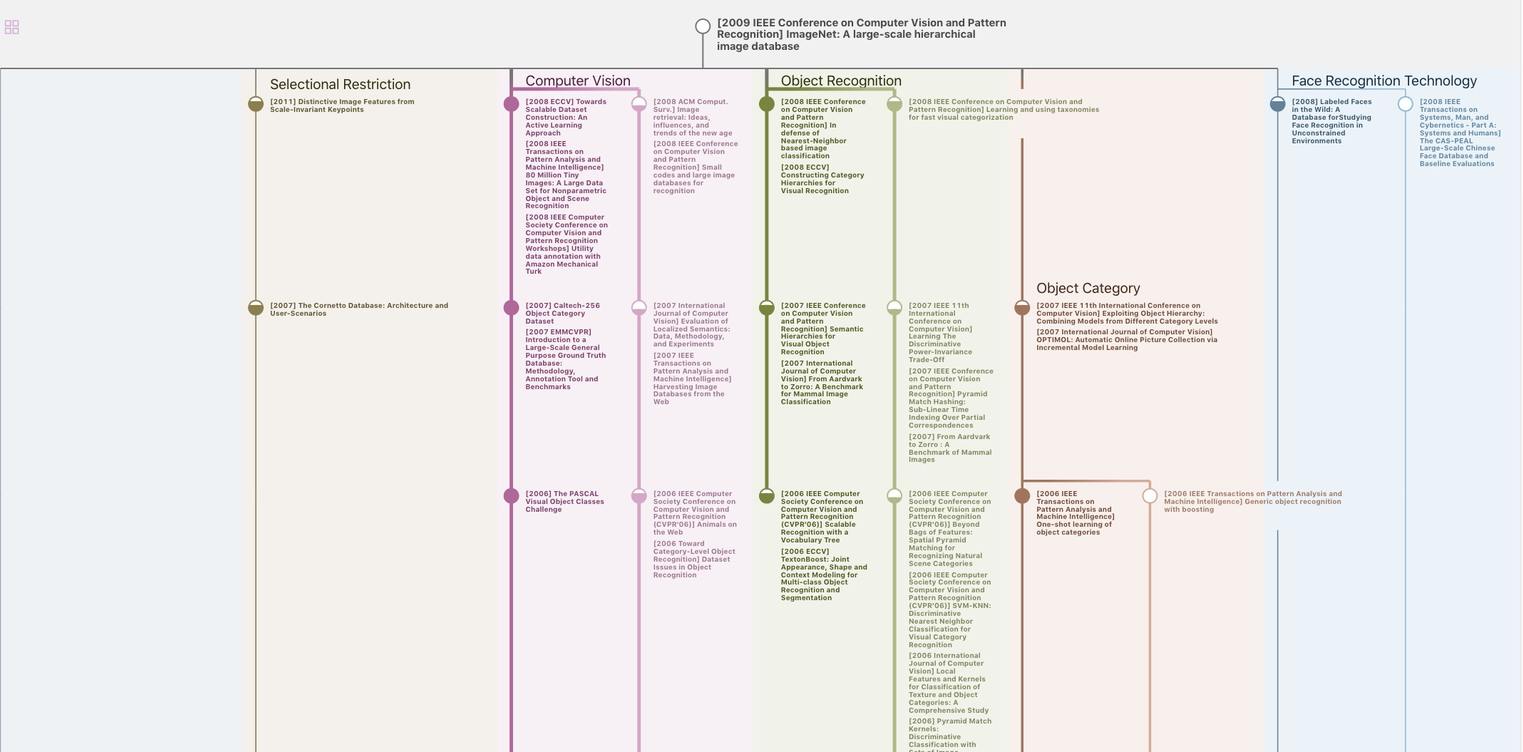
生成溯源树,研究论文发展脉络
Chat Paper
正在生成论文摘要