Self-Supervised Learning of Depth and Ego-Motion for 3D Perception in Human Computer Interaction
ACM TRANSACTIONS ON MULTIMEDIA COMPUTING COMMUNICATIONS AND APPLICATIONS(2024)
摘要
3D perception of depth and ego-motion is of vital importance in intelligent agent and Human Computer Interaction (HCI) tasks, such as robotics and autonomous driving. There are different kinds of sensors that can directly obtain 3D depth information. However, the commonly used Lidar sensor is expensive, and the effective range of RGB-D cameras is limited. In the field of computer vision, researchers have done a lot of work on 3D perception. While traditional geometric algorithms require a lot of manual features for depth estimation, Deep Learning methods have achieved great success in this field. In this work, we proposed a novel self-supervised method based on Vision Transformer (ViT) with Convolutional Neural Network (CNN) architecture, which is referred to as ViT-Depth. The image reconstruction losses computed by the estimated depth and motion between adjacent frames are treated as supervision signal to establish a self-supervised learning pipeline. This is an effective solution for tasks that need accurate and low-cost 3D perception, such as autonomous driving, robotic navigation, 3D reconstruction, and so on. Our method could leverage both the ability of CNN and Transformer to extract deep features and capture global contextual information. In addition, we propose a cross-frame loss that could constrain photometric error and scale consistency among multi-frames, which lead the training process to be more stable and improve the performance. Extensive experimental results on autonomous driving dataset demonstrate the proposed approach is competitive with the state-of-the-art depth and motion estimation methods.
更多查看译文
关键词
Autonomous driving,3D perception,monocular depth and motion estimation,Self-supervised Learning,visual SLAM
AI 理解论文
溯源树
样例
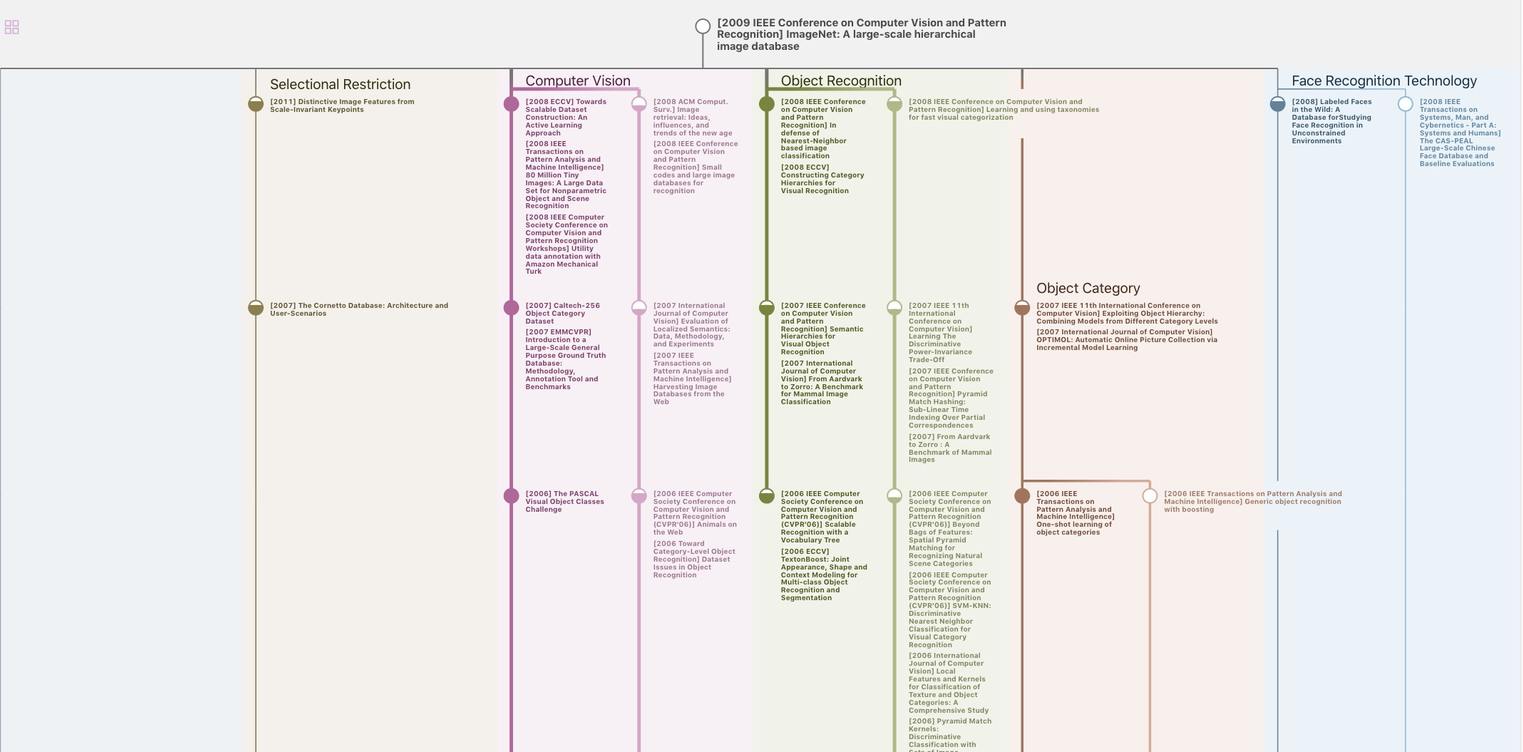
生成溯源树,研究论文发展脉络
Chat Paper
正在生成论文摘要