Estimating Shape Distances on Neural Representations with Limited Samples
ICLR 2024(2023)
摘要
Measuring geometric similarity between high-dimensional network representations is a topic of longstanding interest to neuroscience and deep learning. Although many methods have been proposed, only a few works have rigorously analyzed their statistical efficiency or quantified estimator uncertainty in data-limited regimes. Here, we derive upper and lower bounds on the worst-case convergence of standard estimators of shape distance$\unicode{x2014}$a measure of representational dissimilarity proposed by Williams et al. (2021). These bounds reveal the challenging nature of the problem in high-dimensional feature spaces. To overcome these challenges, we introduce a new method-of-moments estimator with a tunable bias-variance tradeoff. We show that this estimator achieves superior performance to standard estimators in simulation and on neural data, particularly in high-dimensional settings. Thus, we lay the foundation for a rigorous statistical theory for high-dimensional shape analysis, and we contribute a new estimation method that is well-suited to practical scientific settings.
更多查看译文
关键词
representational geometry,shape metrics,dissimilarity metrics
AI 理解论文
溯源树
样例
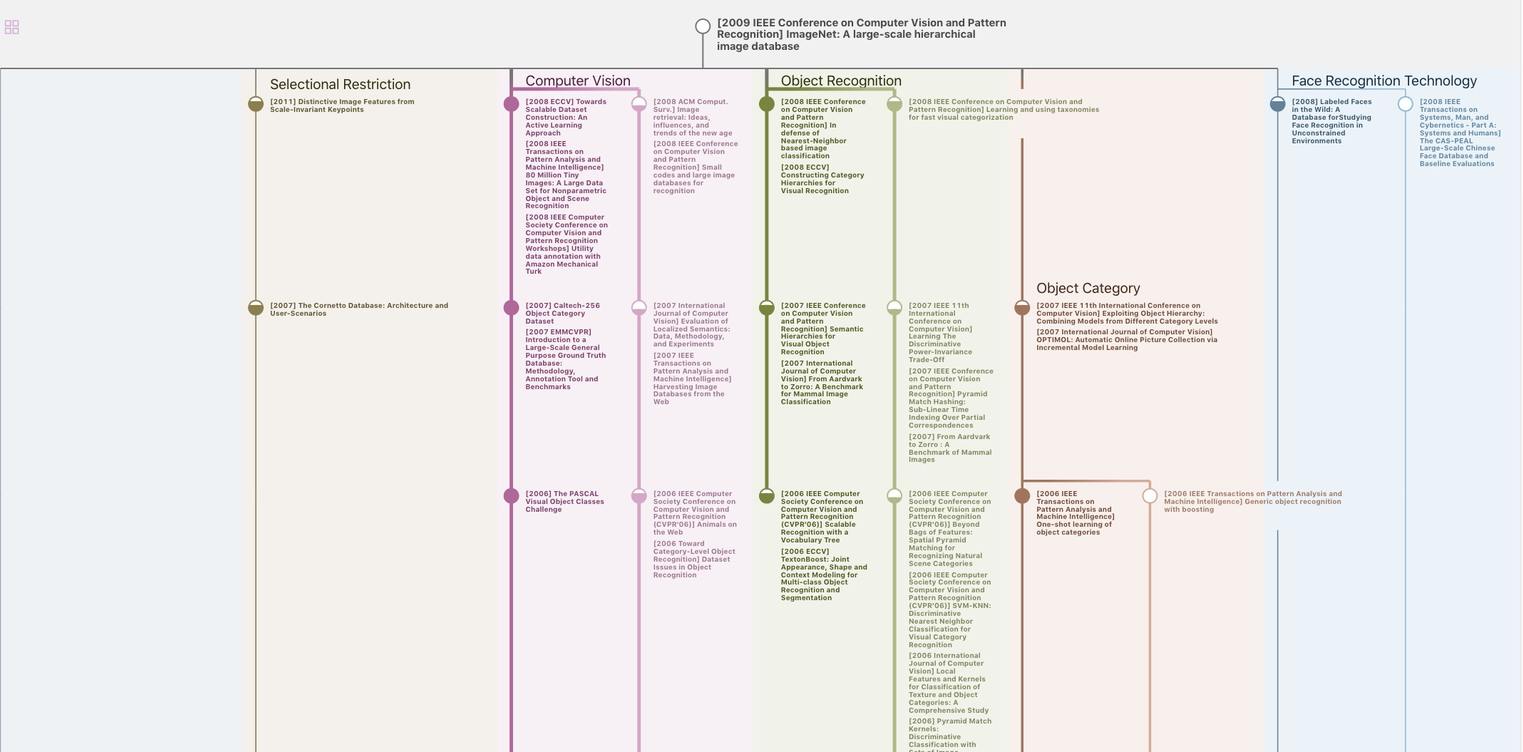
生成溯源树,研究论文发展脉络
Chat Paper
正在生成论文摘要