Terminology-Aware Translation with Constrained Decoding and Large Language Model Prompting.
CoRR(2023)
摘要
Terminology correctness is important in the downstream application of machine translation, and a prevalent way to ensure this is to inject terminology constraints into a translation system. In our submission to the WMT 2023 terminology translation task, we adopt a translate-then-refine approach which can be domain-independent and requires minimal manual efforts. We annotate random source words with pseudo-terminology translations obtained from word alignment to first train a terminology-aware model. Further, we explore two post-processing methods. First, we use an alignment process to discover whether a terminology constraint has been violated, and if so, we re-decode with the violating word negatively constrained. Alternatively, we leverage a large language model to refine a hypothesis by providing it with terminology constraints. Results show that our terminology-aware model learns to incorporate terminologies effectively, and the large language model refinement process can further improve terminology recall.
更多查看译文
关键词
constrained decoding,language,terminology-aware
AI 理解论文
溯源树
样例
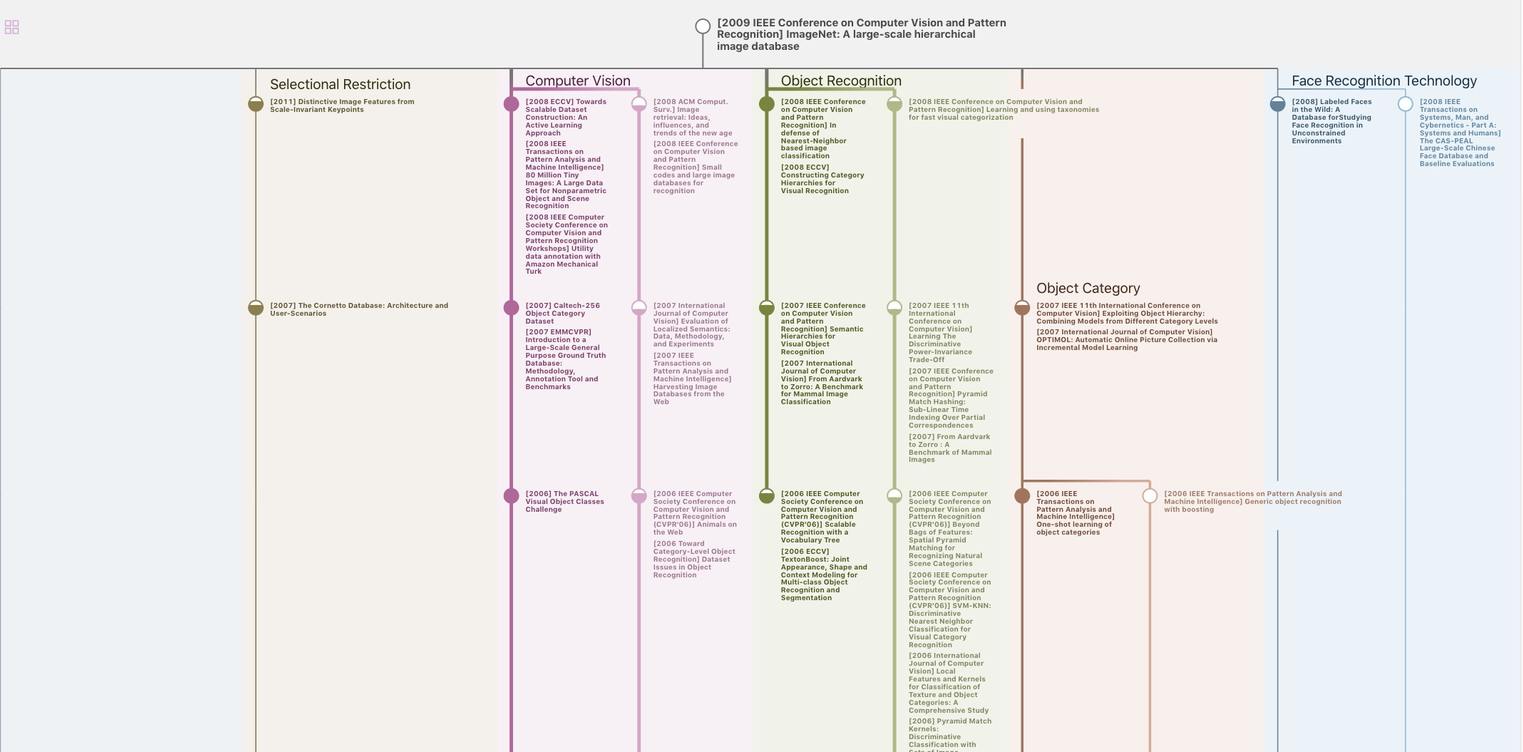
生成溯源树,研究论文发展脉络
Chat Paper
正在生成论文摘要