Towards Verifiable Generation: A Benchmark for Knowledge-aware Language Model Attribution
CoRR(2023)
摘要
Although achieving great success, Large Language Models (LLMs) usually suffer from unreliable hallucinations. In this paper, we define a new task of Knowledge-aware Language Model Attribution (KaLMA) that improves upon three core concerns on conventional attributed LMs. First, we extend attribution source from unstructured texts to Knowledge Graph (KG), whose rich structures benefit both the attribution performance and working scenarios. Second, we propose a new ``Conscious Incompetence" setting considering the incomplete knowledge repository, where the model identifies the need for supporting knowledge beyond the provided KG. Third, we propose a comprehensive automatic evaluation metric encompassing text quality, citation quality, and text citation alignment. To implement the above innovations, we build a dataset in biography domain BioKaLMA via a well-designed evolutionary question generation strategy, to control the question complexity and necessary knowledge to the answer. For evaluation, we develop a baseline solution and demonstrate the room for improvement in LLMs' citation generation, emphasizing the importance of incorporating the "Conscious Incompetence" setting, and the critical role of retrieval accuracy.
更多查看译文
关键词
verifiable generation,attribution,language
AI 理解论文
溯源树
样例
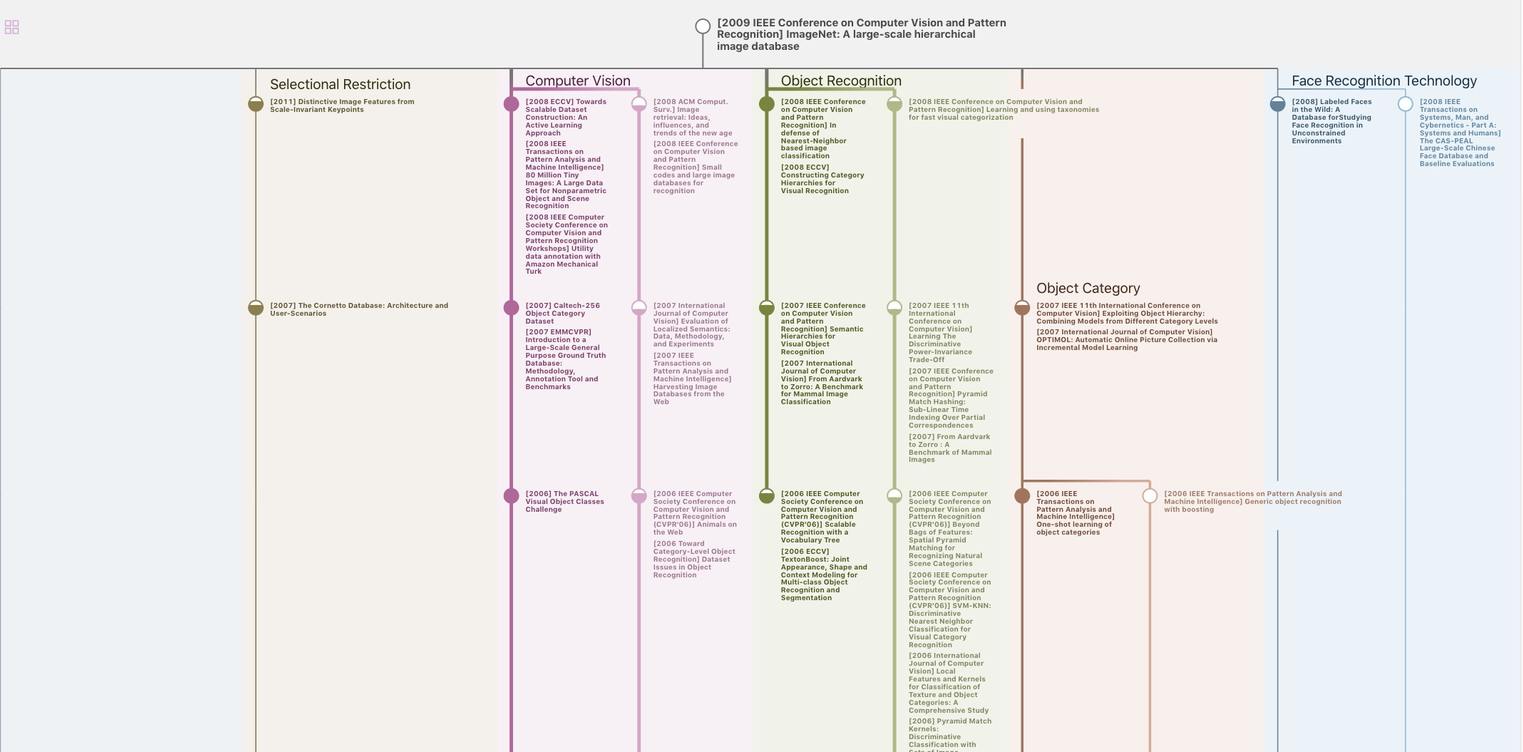
生成溯源树,研究论文发展脉络
Chat Paper
正在生成论文摘要